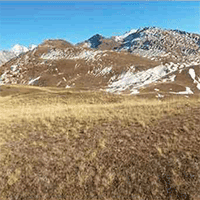
Harmonized dataset of surface fuels under Alpine, temperate and Mediterranean conditions in Italy. A synthesis supporting fire management
iForest - Biogeosciences and Forestry, Volume 13, Issue 6, Pages 513-522 (2020)
doi: https://doi.org/10.3832/ifor3587-013
Published: Nov 13, 2020 - Copyright © 2020 SISEF
Research Articles
Abstract
Surface biomass characterization plays a key role in wildfire management. It allows classifying vegetation fuels flammability for fire risk analysis, to define silvicultural prescriptions for fire hazard reduction, to plan prescribed burning, or to model fire behavior and its effects, such as greenhouse gas and pollutant emissions. To facilitate fuel classification and analysis of potential fire behavior and effects in Italy, we harmonized 634 measurements of surface wildland fuels from Alpine, temperate and Mediterranean environments. The dataset provides quantitative data for duff, fine dead fuels and downed woody material, live grasses and shrubs fuel components. Surface fuel data were harmonized by subdividing loads (Mg ha-1) to standard size classes for dead (0-6, 6-25 and 25-75 mm) and live (0-6, 6-25 mm) fuels, collecting percent cover and depth/height (cm) of the various fuel components, and classifying observations into 19 fuelbed categories. To ensure comparability with existing vegetation classification systems, we classified each observation according to the European Fuel Map, the Corine Land Cover classes (level IV), the European Forest Types, and the forest categories of the Italian National Forest Inventory. The dataset and a photo description of each fuelbed category are available as Supplementary material. This dataset is the first step to develop several products at the national scale such as: (i) fuel type classification and mapping; (ii) carbon stock and wildfire emission estimates; (iii) calibration of fuel models for the simulation of fire behavior and effects.
Keywords
Introduction
Fire is a widespread disturbance in most vegetation types in Italy under Alpine, temperate and Mediterranean conditions ([30]). Regional fire management agencies and National Parks are in an increasing need of fire risk assessment, estimates of fire emissions at different spatial scales, fuel management and prescribed burning planning ([6], [21], [9]). The use of decision support systems (DSS) for fire effects modeling, fire behavior simulation and risk analysis is spreading in both the research and operational sectors in Italy ([53], [58], [28]). A quantitative characterization of fuels in Italian forests, shrublands and grasslands is the first step towards developing harmonized fuel datasets to support fire management decisions and calibrating fuel inputs for fire software applications and DSS used by professionals and researchers ([8], [7]).
Several approaches have been proposed to quantify and classify fuel complexes, each compatible with a specific modeling system for fire behavior and effects prediction ([55], [24], [1], [39], [22]). Fire effect models, e.g., FOFEM ([50]), requires several information about fuel components (e.g., litter, downed woody fuel), often based on vegetation types or fuelbed characteristics ([50], [44]). Widespread DSS for fire behavior prediction - such as BehavePlus ([3]), Farsite ([33]), FlamMap ([34]), the Fire and Fuel Extension to the Forest Vegetation Simulator ([2]), or the Rothermel R package ([59]) - require a number of parameters represented by “fire behavior fuel models” as input (hereafter “fuel model”), related to surface fuels according to the fuel modeling system developed by Rothermel ([52]). However, a source of uncertainty in available fuel description derives from the use of different terminologies to indicate similar fuel classes or categories ([39]). This paper employs the terminology provided by Keane ([40]), who defined the fuelbed as the array of biomass types for a given area, further composed by fuel types (e.g., litter, shrubs, grasses, downed woody material and tree crown foliage) and components (e.g., diameter size range; live or dead status) with specific properties. Furthermore, a fuel complex identifies the dominant fuel of a fuelbed ([11]).
Due to the increased use of Rothermel’s based fire software and DSS in Italy by fire managers and researchers ([4], [5], [8], [59], [7], [29], [54]), a standardized field protocol for inventorying surface fuels according to Rothermel’s fuel models inputs has been proposed in Italy ([13], [14]). This protocol represented the basis for several unpublished surveys carried out nationwide in the last decade. However, a complete and harmonized inventory of Italian wildland fuels is still lacking.
The aims of the present paper are to: (i) harmonize and make freely available unpublished surface fuel data across major vegetation types in Italy; (ii) categorize data into fuelbeds and establish associations with land cover classes and forest classifications used in Europe and Italy; (iii) summarize fuel data for each fuelbed, providing descriptive statistics of average characteristics and their variability; (iv) release a fuel characteristics dataset useful to calibrate Rothermel’s based models and DSS.
Material and methods
Sampling design
Provided the spatial distribution of the available data, an a priori sampling design was not applicable to this study, which is instead based on an a posteriori collection of unpublished inventories carried out over the last 10 years. Among existing surveys, only those with data harmonizable in terms of the protocol adopted to characterize the surface fuel complex were included in the study. Most fuel observations in the dataset (65%) followed the field protocol described by Bovio & Ascoli ([13]) and Bovio et al. ([14]). This uses a mixed approach with both destructive (i.e., vegetation removed and analyzed in the laboratory) and non-destructive techniques (see next paragraph), such as indirect estimates of fuel loads based on allometry equations ([20], [17], [56], [19]). The remaining 35% of survey data adopted protocols that are similar to the ones mentioned, but applied a fully destructive sampling to the shrub component, as they were mostly carried out in shrubland fuelbeds ([8], [26], [17], [58]).
The study sites encompass grasslands, shrublands and forest ecosystems growing in Alpine, temperate, and Mediterranean environments throughout a large part of insular and peninsular Italy (Fig. 1). Site selection excluded forests disturbed in the previous 10 years (e.g., affected by wildfire or windthrow, intensively grazed areas, or silvicultural interventions); thus the data set includes 377 sites with a number of randomly located sample plots ranging from 1 to 15 per site (average of 2.28 ± 2.11). According to the aforementioned protocols ([13], [14]), the structure of the fuel complex was assessed along three 10-m long transects, positioned to form an equilateral triangle (i.e., each transect had a different direction to avoid directional bias). Along each transect, surface litter depth, grasses height, and shrub crown height were measured at 1 m intervals. Surface litter and duff were collected through destructive sampling in three 0.0625 m2 squares (1 square along each transect); downed wood < 75 mm, grasses and shrub fuels were collected on three 1 m2 squares. Harvested fuels were oven-dried in the laboratory at 90 °C for 12 to 48 hours depending on the fuel component (i.e., 12 hours only for fully cured, very fine grasses) to determine the dry weight ([46], [25], [57]). For each sampling point, we calculated the dry weight load of dead (duff, litter, downed woody material, dead fraction of grasses and shrubs) and live (grasses, shrubs) fuel components and structural variables as described in the next paragraph and synthetized in the Fig. S1 (Supplementary material).
Fig. 1 - Distribution of study sites (black dots) where fuel data were inventoried in Italy. Pie charts represent the relative amount of samples in different fuel complexes (grass only, grass + shrub, litter only, litter + grass + shrub, shrub only). Pie dimension is relative to sample abundance.
Dataset description
The dataset includes 634 surface fuel samples, each described by 41 variables (Tab. S1), and is available in Excel® (.xlsx) format as Supplementary material (Tab. S3). The first dataset field is an identification code (ID) combining the broad fuel type (2nd field), the study site code (3rd field), and the number of the fuel sample collected at each site (4th field), useful for filtering data. According to the protocol ([13], [14]), surveys were carried out during the typical environment-specific fire season, to describe fuel characteristics dynamics (e.g., level of grass curing) in the nature of their flammable status. The field SamplingSeason (Tab. S1) reports winter or summer classes, corresponding to Alpine and Mediterranean fire regime seasons in Italy ([30]), respectively. FuelComplex is a broad fuel classification system to identify the dominant fuel of a fuelbed driving fire spread (i.e., litter, grass, shrubs, grass + shrubs, litter + grass + shrubs). Fields from the 5th position to the 20th are variables that quantitatively characterize each fuel sample (Fig. S1). All fields with a W as the first letter (i.e., Wduff, W1h, W10h, W100h, WGr, WSh1h, WSh10h) report fuel load in Mg ha-1 on a dry weight basis. Loads of dead fuels (W1h, W10h, W100h) are partitioned by particle diameter classes (<6 mm, 6-25 mm, 25-75 mm, >75 mm), corresponding to timelag classes 1h, 10h, 100h, i.e., the amount of time necessary for dead fuels to lose-gain 63% of the difference between their initial moisture content and their equilibrium moisture ([15]). Live grasses (WGr) included <6 mm fuels only and cured grasses were added to the 1h dead fuels. Live shrub fuel load was partitioned in <6 mm (WSh1h) and 6-25 mm (WSh10h) size classes. Fuel cover (%) and fuelbed depth (cm) of dead fuels (Cdead, Ddead), live grasses (CGr, DGr) and shrubs (CSh, DSh) fuels were measured in 81% and 79% of sampling points, respectively. In addition, 45% of observations included data on duff fuel load (Wduff) - a key component to estimate fire emissions, especially in forest ecosystems - while 96% of observations reported an estimate of the tree canopy cover (CanCov). Each observation contains information about elevation, slope and aspect, and climate information according to the Köppen-Geiger climate classification ([12]) and the “Worldwide Bioclimatic Classification System” ([51]) available in raster format for Italy at 900 m grain ([47]). Most sampling sites belong to Köppen-Geiger temperate and mesothermal climates (41% Csa Mediterranean hot summer, 32% Cfb Oceanic, 21% Cfa Humid subtropical), with a small fraction (6%) of boreal and tundra climates at the topmost elevations up to 2100 m a.s.l. From Rivas-Martinez classification, we extracted values for bioclimate, continentality type based on the difference between annual maximum and minimum temperature (63% subcontinental and semicontinental, 37% euoceanic or semihyperoceanic), ombrotype (Tab. S1) based on the annual ombrothermic index (24% dry and semiarid, 21% subhumid, 55% humid and hyperhumid), and thermotype (Tab. S1 in Supplementary material) based on thermicity index and yearly positive temperatures (38% Thermo-, Meso- and Supramediterranean , 23% Mesotemperate, 39% Supra- and Orotemperate).
Fuel observations were then grouped into 19 surface fuelbeds (fields: FuelBed, FuelBedCode - Tab. 1), based on expert opinion considering the vegetation type, dominant fuel, its spatial arrangement and species-specific flammability properties that determine potential surface fire behavior ([44], [39]). For example, fuel observations in Mediterranean conifer forests with a long-needle and porous litter (i.e., Pinus halepensis, P. pinaster and P. pinea forests) were grouped into two fuelbeds according to the absence (fuelbed 16) or presence (fuelbed 17) of flammable understory grasses and shrubs. Fuelbeds with codes from 1 to 3 represent grass-dominated fuel complexes, 4 to 6 shrublands, 7 to 12 broadleaved forests, 13 to 17 coniferous forests, while 18 and 19 relate to riparian vegetation and marshes, respectively. A photo description of each fuelbed is provided in Fig. S2 (Supplementary material).
Tab. 1 - Fuelbed association with the European Forest Fire Information System (EFFIS) fuel map classes, Corine Land Cover classes (level IV), the European Forest Types and the forest categories of the Italian National Forest Inventory (INFC). For fuelbeds 1 to 6, 12 and 19, there was no meaningful correspondence (“na”) with either the European Forest Types or the forest categories of the Italian National Forest Inventory.
Fuelbed | EFFIS fuel map class | Corine LC IV level | EU Forest Types | INFC Categories |
---|---|---|---|---|
1. Sparse and very short grasslands | 4. Sparse grasslands | 3212. Discontinuous grassland | na | na |
2. Continuous short grasslands | 5. Mediterranean grasslands and steppes; 6. Temperate, Alpine and Northern grasslands |
3211. Continuous grassland | na | na |
3. Continuous tall Mediterranean grasslands | 5. Mediterranean grasslands and steppes | 3211. Continuous grassland | na | na |
4. Temperate and Alpine heathlands | 8. Temperate, Alpine and Northern moors and heathlands | 322. Moors and heathlands | na | na |
5. Short Mediterranean shrublands and garrigues | 9. Mediterranean open shrublands (sclerophylous) | 3232. Low maquis and garrigues | na | na |
6. Tall Mediterranean shrublands and heathlands | 10. Mediterranean shrublands (sclerophylous) | 3231. High maquis | na | na |
7. Montane beech litter | 33. Montane beech forest; 38. Mixed beech with conifers forest |
3115. Beech forests | 7.1 South western European mountainous beech forest; 7.3 Apennine-Corsican mountainous beech forest |
Faggete |
8. Compact mesophytic broadleaved litter | 31. Mesophytic broadleaved forest | 2241. Young tree plantations for wood production; 3112. Deciduous oak forests; 3113. Mesophilous broad-leaved forests; 3117. Non-native broadleaved forests | 5.1 Pedunculate oak-hornbeam forest; 5.9 Other mesophytic deciduous forests; 8.2 Turkey oak, Hungarian oak and Sessile oak forest; 14 Introduced tree species forest | Boschi a rovere, roverella e farnia; Cerrete, boschi di farnetto, fragno, vallonea; Ostrieti, carpineti |
9. Porous thermophilous broadleaved litter | 30. Thermophilous broadleaved forest | 3112. Deciduous oak forests 3113. Mesophilous broad-leaved forests | 8.1 Downy oak forest; 8.2 Turkey oak, Hungarian oak and Sessile oak forest; 8.8 Other thermophilous deciduous forests | Boschi a rovere, roverella e farnia; Cerrete, boschi di farnetto, fragno, vallonea; Ostrieti, carpineti |
10. Mediterranean evergreen broadleaved litter | 29. Mediterranean evergreen broadleaved forest | 3111. Mediterranean evergreen oak forests | 9.1 Mediterranean evergreen oak forest | Leccete; Sugherete; Altre latifoglie sempreverdi |
11. Long broadleaved litter | 30. Thermophilous broadleaved forest | 3114. Chestnut forests | 8.7 Chestnut forest | Castagneti |
12. Eucaliptus litter | na | 3117. Non-native broad-leaved forests | 14.2 Plantations of not-site-native species and self-sown exotic forest | na |
13. Alpine and Mediterranean short needled conifer litter | 23. Mediterranean montane short needled conifer forest (fir); 26. Alpine short needled conifer forest (fir, alpine spruce) |
3123. Silver fir and/or Norway spruce forests | 3.2 Subalpine and mountainous spruce and mountainous mixed spruce-silver fir forest; 7.9 Mountainous Silver fir forest | Boschi di abete rosso; Boschi di abete bianco |
14. Montane long needled conifer litter | 22. Mediterranean montane long needled conifer forest (Black and scots pines); 25. Alpine long needled conifer forest (pine) |
3122. Mountain and oro-Mediterranean pine forests; 3124. Larch and/or Arolla pine forests; 3125. Non-native coniferous forests |
10.2 Mediterranean and Anatolian Black pine forest; 14.2 Plantations of not-site-native species and self-sown exotic forest; 3.1 Subalpine larch-arolla pine and dwarf pine forest; 3.3 Alpine Scots pine and Black pine | Pinete di pino silvestre e montano; Pinete di pino nero, laricio e loricato; Piantagioni di conifere |
15. Montane long needled conifer understory with shrubs | 22. Mediterranean montane long needled conifer forest (Black and scots pines); 25. Alpine long needled conifer forest (pine) |
3122. Mountain and oro-Mediterranean pine forests 3124. Larch and/or Arolla pine forests | 10.2 Mediterranean and Anatolian Black pine forest; 14.2 Plantations of not-site-native species and self-sown exotic forest; 3.1 Subalpine larch-arolla pine and dwarf pine forest; 3.3 Alpine Scots pine and Black pine | Pinete di pino silvestre e montano; Pinete di pino nero, laricio e loricato; Piantagioni di conifere |
16. Mediterranean long needled conifer litter | 20. Mediterranean long needled conifer forest (Med. pines) | 3121. Mediterranean pine forests | 10.1 Mediterranean pine forest | Pinete di pini mediterrane |
17. Mediterranean long needled conifer understory with shrubs | 20. Mediterranean long needled conifer forest (Med. pines) | 3121. Mediterranean pine forests | 10.1 Mediterranean pine forest | Pinete di pini mediterrane |
18. Riparian vegetation | 39. Riparian vegetation | 3116. Hygrophilous forests | 12.1 Riparian forest | Boschi igrofili |
19. Aquatic marshes | 41. Aquatic marshes | na | na | na |
The vegetation physiognomy at each sample point was used to associate (sensu [39]) fuelbed classes to relevant fuel, land cover and forest classification systems. Cross-links were established with existing vegetation classification systems in Europe and in Italy (Tab. 1): (i) European fuel map classification ([27]); (ii) Corine Land Cover, level IV ([38]); (iii) European Forest Types ([10]); and (iv) forest categories of the Italian National Forest Inventory ([37]), for forest fuelbeds only. In some cases, a fuelbed was uniquely associated with a vegetation class. For example, fuelbed 7 “Mountain beech litter”, which has a distinctive combustibility due to the compactness of the beech litter and the lack of grasses and shrubs in the understory typical in mountain beech forests ([42]), was uniquely associated with the “Faggete” class in the INFC (Tab. 1). However, different fuelbeds may be associated to the same vegetation class, and conversely, more vegetation classes may be associated to the same fuelbed description (e.g., fuelbeds 8 and 9 are both associated to the European Forest Type 8.2 and the INFC category “Boschi a rovere, roverella e farnia”), which is a typical issue in fuelbed classification systems ([39]).
In addition to surface fuel data, a bibliographic research on crown foliage and branch fuel loads is presented in Tab. S2 (Supplementary material) with the aim to complement forest fuelbeds (from 7 to 19) with canopy information, necessary for several fire effect models, e.g., FOFEM ([50]).
Results
Dataset descriptive statistics are presented by the sampled variability across the fuel complexes (i.e., dominant fuel of the fuelbed), and then by the variability within the fuelbeds. A comparison with international established fuel inventories is also presented to verify that the range in loads by fuel components falls within the range of variability in reference inventories (see also the Discussion). Finally, a multivariate analysis was carried out to describe geographical and climatic gradients in the dataset.
Dataset descriptive statistics
Fuel complexes show distinct characteristics (Fig. 2). Loads of dead fine fuels (size <6 mm) and downed fuels (size 6-75 mm) were generally higher in fuel complexes with litter only (LI), or litter mixed with grasses and shrubs (LS), and shrubs only (SH). Although in grasses the mean load of dead fine fuels was very low, several outliers were recorded (Fig. 2a), mainly due to fuelbed 3 “Continuous tall Mediterranean grasslands”, which is dominated by the tall grass Ampelodesmos mauritanicus (Poir.) T. Durand & Schinz and characterized by relatively high loads of fine dead leaves within the tussock. This represents a species-specific surface fire environment characterizing fire-prone grasslands of the southern Tyrrhenian cost ([36]) and was therefore identified as a specific fuelbed (fuelbed 3 in Fig. S1). Live fuel load with particle size 6-25 mm was particularly abundant in the shrub fuel complex (Fig. 2d).
Fig. 2 - Boxplot of fuel load (Mg ha-1) by fuel complex (GR = grass; GS = grass + shrub; LI = litter only; LS = litter + grass + shrub; SH = shrub only) and fuel components: (a) Dead fuel with particle size < 6 mm; (b) larger dead downed fuels (size 6-75 mm); (c) live grass fuels (< 6 mm); (d) live shrub fuels (<25 mm). Data excluded: duff fuel component; fuelbed 19 “Aquatic marshes”.
Tab. 2 and Tab. 3 provides descriptive statistics of mean characteristics and variability of fuel loads and cover for grasslands and shrublands fuelbeds (Tab. 2) and for broadleaved and coniferous forests, and aquatic fuelbeds (Tab. 3). The fuelbed with the lowest value in total surface fuel load (excluding duff) is fuelbed 1 “Sparse and very short grasslands” with an average of 2.47 Mg ha-1. Fuelbed 19 “Aquatic marshes” shows the highest total surface fuel load with an average of 80 Mg ha-1. Although sharing the same vegetation classes (Tab. 3), long-needle conifer forests of both montane and Mediterranean areas showed marked differences between fuelbed with litter fuels only (fuelbeds 14 and 16) and litter mixed with grasses and shrubs (fuelbeds 15 and 17). For example, shrub live loads (particle size <25 mm), cover and depth in fuelbeds 16 and 17 was 0.87 vs. 11.30 Mg ha-1, 12% vs. 45%, and 47 cm vs. 157 cm, respectively, highlighting the marked difference among these fuelbeds, though associations to vegetation classifications are the same (Tab. 3).
Tab. 2 - Number of observations (Count), mean fuel load (Mg ha-1), standard deviation (STD), range (min-max) and interquartile range (IQR) by vegetation type (grassland, shrubland), fuelbed and fuel component. Variable names: Duff: fuel duff load; Dead 1h: dead fuels with particle size < 6 mm; Dead (10h, 100h): dead fuels with particle size 6-25 mm, 25-75 mm; Live fuels (grass, shrub): live grasses < 6 mm and live shrubs < 25 mm; Total load: total fuel load (dead + live fuels, but duff excluded).
Type | Fuelbed | Fuel component |
Count | Mean | STD | Range | IQR |
---|---|---|---|---|---|---|---|
Grasslands | 1. Sparse and very short grasslands | Duff | na | na | na | na | na |
Dead 1h | 41 | 0.08 | 0.31 | 0-1.7 | 0 | ||
Dead (10h, 100h) | 41 | 0.08 | 0.37 | 0-2.2 | 0 | ||
Live fuels (grass, shrub) | 41 | 2.32 | 1.48 | 0.3-7.2 | 1.4 | ||
Total load | 41 | 2.47 | 1.66 | 0.3-8.9 | 1.5 | ||
2. Continuous short grasslands | Duff | 4 | 23.7 | 24.78 | 4.6-60.1 | 15.6 | |
Dead 1h | 43 | 0.28 | 0.9 | 0-4.8 | 0 | ||
Dead (10h, 100h) | 43 | 0.03 | 0.18 | 0-1.1 | 0 | ||
Live fuels (grass, shrub) | 43 | 3.77 | 2.03 | 0.5-10.3 | 2.6 | ||
Total load | 43 | 4.08 | 2.27 | 0.5-10.8 | 3 | ||
3. Continuous tall Mediterranean grasslands | Duff | 25 | na | na | na | na | |
Dead 1h | 25 | 3.96 | 4.54 | 0-15.7 | 4.3 | ||
Dead (10h, 100h) | 25 | 0 | 0 | 0-0 | 0 | ||
Live fuels (grass, shrub) | 25 | 12.26 | 7.25 | 3.5-29.5 | 7.8 | ||
Total load | 25 | 16.17 | 10.44 | 3.5-40.7 | 10.2 | ||
Shrublands | 4. Temperate and Alpine heathlands | Duff | 11 | 3.18 | 2.36 | 0.1-6.9 | 3.8 |
Dead 1h | 11 | 2.35 | 2.38 | 0.03-6.2 | 3 | ||
Dead (10h, 100h) | 11 | 0 | 0 | 0-0 | 0 | ||
Live fuels (grass, shrub) | 11 | 6.15 | 2.37 | 3.3-10.6 | 3.4 | ||
Total load | 11 | 8.5 | 4.33 | 3.6-16.2 | 5.6 | ||
5. Short Mediterranean shrublands and garrigues | Duff | 25 | 6.89 | 4.36 | 0.2-16.7 | 5.5 | |
Dead 1h | 51 | 2.26 | 2.19 | 0-12.9 | 1.9 | ||
Dead (10h, 100h) | 51 | 2.92 | 5.5 | 0-32 | 2.7 | ||
Live fuels (grass, shrub) | 51 | 10.35 | 7.69 | 1.8-37.5 | 6.6 | ||
Total load | 51 | 15.52 | 11.03 | 2.5-54.5 | 10.5 | ||
6. Tall Mediterranean shrublands and heathlands | Duff | 11 | 11.65 | 4.24 | 6.5-20.1 | 5.4 | |
Dead 1h | 39 | 5.33 | 4.34 | 0.7-12.3 | 8.4 | ||
Dead (10h, 100h) | 39 | 3.55 | 3.2 | 0-17.8 | 3.8 | ||
Live fuels (grass, shrub) | 39 | 25.53 | 8.67 | 10.4-47.3 | 15.7 | ||
Total load | 39 | 34.42 | 12.59 | 16.8-53.3 | 25.3 |
Tab. 3 - Number of observations (Count) and fuel load (Mg ha-1) by vegetation type (broadleaved forests, conifer forests, aquatic vegetation), fuelbed and fuel component. Mean, standard deviation (STD), range (min-max) and interquartile range (IQR) are reported. Variable names: Duff: duff fuel load; Dead 1h: dead fuels with particle size < 6 mm; Dead (10h, 100h): dead fuels with particle size 6-25 mm and 25-75 mm, respectively; Live fuels (grass, shrub): live grasses < 6 mm and live shrubs < 25 mm; Total load: total fuel load (dead + live fuels, excluding duff).
Type | Fuelbeds | Fuel component |
Count | Mean | STD | Range | IQR |
---|---|---|---|---|---|---|---|
Broadleaved forests | 7. Montane beech litter | Duff | 7 | 35.06 | 26.75 | 9.3-90.1 | 16.5 |
Dead 1h | 17 | 1.88 | 2.37 | 0.1-6.74 | 3.1 | ||
Dead (10h, 100h) | 17 | 4.09 | 3.91 | 0.3-14.8 | 3.3 | ||
Live fuels (grass, shrub) | 17 | 0.24 | 0.55 | 0-2.2 | 0.2 | ||
Total load | 17 | 6.2 | 4.26 | 0.5-14.9 | 7.1 | ||
8. Compact mesophitic broadleaved litter | Duff | 68 | 33.07 | 25.84 | 2.3-92.4 | 40.1 | |
Dead 1h | 95 | 2.18 | 1.87 | 0.1-8 | 3.1 | ||
Dead (10h, 100h) | 95 | 6.83 | 6.1 | 0-28.4 | 7.5 | ||
Live fuels (grass, shrub) | 95 | 1.61 | 2.54 | 0-16.5 | 1.9 | ||
Total load | 95 | 10.63 | 7.14 | 0.6-37.6 | 9.4 | ||
9. Porous thermophilous broadleaved litter | Duff | 9 | 39.87 | 31.65 | 3-97 | 36.6 | |
Dead 1h | 66 | 1.73 | 2.35 | 0.1-10.6 | 3 | ||
Dead (10h, 100h) | 66 | 3.01 | 3.33 | 0-13.3 | 4.2 | ||
Live fuels (grass, shrub) | 66 | 1.58 | 1.83 | 0-9.8 | 2.1 | ||
Total load | 66 | 6.32 | 3.84 | 1-21 | 4.4 | ||
10. Mediterranean evergreen broadleaved litter | Duff | na | na | na | na | na | |
Dead 1h | 19 | 2.25 | 1.15 | 0.1-3.8 | 1.5 | ||
Dead (10h, 100h) | 19 | 4.69 | 4.82 | 0.2-15.9 | 7.1 | ||
Live fuels (grass, shrub) | 19 | 3.76 | 6.52 | 0-27.3 | 2.7 | ||
Total load | 19 | 10.71 | 7.74 | 2.3-31.7 | 9.5 | ||
11. Long broadleaved litter | Duff | 33 | 35.69 | 27.5 | 5.8-104 | 41.5 | |
Dead 1h | 46 | 2.52 | 2.65 | 0.1-11.4 | 1.9 | ||
Dead (10h, 100h) | 46 | 6.47 | 4.84 | 0.7-17.1 | 7.3 | ||
Live fuels (grass, shrub) | 46 | 2.08 | 2.83 | 0-13.7 | 2 | ||
Total load | 46 | 11.06 | 6.05 | 2-22.5 | 10.6 | ||
12. Eucaliptus litter | Duff | na | na | na | na | na | |
Dead 1h | 9 | 9.08 | 4 | 0.1-13.9 | 4.2 | ||
Dead (10h, 100h) | 9 | 4.41 | 1.39 | 2.5-6.4 | 1.8 | ||
Live fuels (grass, shrub) | 9 | 0.26 | 0.31 | 0-0.9 | 0.4 | ||
Total load | 9 | 13.74 | 4.63 | 4.6-20.7 | 4 | ||
Conifer forests | 13. Alpine and Mediterranean short needled conifer litter | Duff | 10 | 39.36 | 16.73 | 19.8-63.8 | 25.6 |
Dead 1h | 15 | 2.36 | 2.02 | 0.1-5.9 | 3.8 | ||
Dead (10h, 100h) | 15 | 4.11 | 2.44 | 1-9.1 | 3.3 | ||
Live fuels (grass, shrub) | 15 | 0.44 | 0.54 | 0-1.6 | 0.8 | ||
Total load | 15 | 6.91 | 2.29 | 3-11.7 | 2.9 | ||
14. Montane long needled conifer litter | Duff | 13 | 39.58 | 30.17 | 1.1-93.1 | 49.9 | |
Dead 1h | 36 | 3.32 | 2.63 | 0.1-8.7 | 4.2 | ||
Dead (10h, 100h) | 36 | 3.96 | 3.75 | 0-14.4 | 4.9 | ||
Live fuels (grass, shrub) | 36 | 0.79 | 1.02 | 0-4.7 | 0.8 | ||
Total load | 36 | 8.07 | 5.29 | 0.7-22.9 | 7.5 | ||
15. Montane long needled conifer understory with shrubs | Duff | 24 | 23.92 | 20.1 | 2.7-74.1 | 11.7 | |
Dead 1h | 24 | 1.11 | 0.94 | 0.1-3.2 | 0.8 | ||
Dead (10h, 100h) | 24 | 11.61 | 11.88 | 0-36.5 | 17.2 | ||
Live fuels (grass, shrub) | 24 | 4.5 | 3.26 | 0.5-12.9 | 3.8 | ||
Total load | 24 | 17.23 | 12.96 | 2.7-45.3 | 19.9 | ||
16. Mediterranean long needled conifer litter | Duff | 12 | 31.81 | 25.73 | 3.4-96.6 | 25.6 | |
Dead 1h | 29 | 2.43 | 1.52 | 0.1-5.6 | 2.2 | ||
Dead (10h, 100h) | 29 | 3.3 | 3.78 | 0.2-16.9 | 2.6 | ||
Live fuels (grass, shrub) | 29 | 0.89 | 0.99 | 0-4.3 | 1.1 | ||
Total load | 29 | 6.61 | 4.23 | 0.8-19.7 | 2.9 | ||
17. Mediterranean long needled conifer understory with shrubs | Duff | 18 | 32.83 | 25.06 | 22.7-91.4 | 18.9 | |
Dead 1h | 44 | 4.31 | 2.77 | 0.5-12 | 3.1 | ||
Dead (10h, 100h) | 44 | 3.42 | 3.28 | 0-14.2 | 3.7 | ||
Live fuels (grass, shrub) | 44 | 11.3 | 8.78 | 1.1-29.3 | 11.9 | ||
Total load | 44 | 20.13 | 11.11 | 4.7-42 | 16.4 | ||
Aquatic vegetation | 18. Riparian vegetation | Duff | na | na | na | na | na |
Dead 1h | - | 0.49 | 0.54 | 0.1-1.2 | 0.9 | ||
Dead (10h, 100h) | 8 | 3.6 | 4.2 | 0.7-10.7 | 3.7 | ||
Live fuels (grass, shrub) | 8 | 3.11 | 4.04 | 0.9-13 | 0.8 | ||
Total load | 8 | 7.2 | 4.94 | 2.6-14.6 | 8.5 | ||
19. Aquatic marshes | Duff | na | na | na | na | na | |
Dead 1h | 10 | 15.22 | 5.43 | 9-26.5 | 7.2 | ||
Dead (10h, 100h) | 10 | 17.73 | 5.54 | 8.1-25.1 | 7.9 | ||
Live fuels (grass, shrub) | 10 | 47.1 | 20.58 | 24.7-76 | 36.8 | ||
Total load | 10 | 80.05 | 20.69 | 53.4-112.7 | 32.4 |
Comparison with international dataset
To verify the congruence of fuel load values devised from the present study with those of similar fuelbeds, the fuel dataset was compared to the Fuel Characteristics Classification System (FCCS - [44]). The FCCS stores and classifies fuels data as fuelbeds across the United States, and provides a description of fuel characteristics and properties for 6 horizontal strata including the duff, litter, downed woody material, grasses, and tree canopy, obtained from literature and field data sets. It represents a helpful way for cataloging fuelbeds consumed during the fire ([44], [45]). Although the FCCS has been created for the United States, it can be used as reference for different ecosystems, thanks to its accurate description of the horizontal strata of each fuel. When comparing the Italian fuel dataset and FCCS for both total fine fuels (size < 6 mm), larger fuels (size 6-75 mm) and duff fuels (Fig. 3), fuel loads in each size class did not differ markedly between the two (except for a higher load of fine fuels in FCCS), which suggests that the Italian inventory explores the fuel data space in a fairly complete way.
Fig. 3 - Comparison between total load (Mg ha-1) of fine fuels (size < 6 mm), larger fuels (size 6-75 mm) and duff loads in both the Italian fuel survey (red) and in the Fine Fuel Classification System database (blue).
Multivariate relationship in the dataset
To test for relationships between fuel loads and topographical and climatic drivers, we carried out a Redundancy Analysis (RDA). Response variables were 1h, 10h and 100h dead fuel loads, live grasses and live shrubs fuel loads; predictors were elevation, slope, southness (calculated as negative cosine of aspect), canopy cover, ombrotype, thermotype and continentality type according to Pesaresi et al. ([47]). Observations were scaled to unit variance; the model was chosen by forward model selection based on adjusted R2 using the “ordiR2step” function in the “vegan” package for R ([49]). The final model was checked for collinearity by making sure that variance Inflation factors for each predictors were <10. The significance of the model, predictors, and RDA axes was checked by an ANOVA-like permutation test with 999 iterations (α =0.05).
The final model had an adjusted R2 of 0.16. The first four RDA axes were significant, and explained 62.3%, 25.8%, 5.3% and 3.5% of the model variance, respectively. Axis 1 was strongly correlated to canopy cover (standardized loading = 0.83) and climate indices (thermotype loading = 0.77, ombrotype = -0.68), while axis 2 was explained by a combination of all predictors (Fig. 4). Fine dead fuel loadings responded to increasing slope, southness and temperature, while live grass and shrub loadings increased in wetter ombrotypes and decreasing elevation and canopy cover. The latter was true also for live grass depth and cover; dead fuel depth and cover were more strongly associated to thermotype/slope and canopy cover/elevation, respectively, while live shrub depth and cover responded mostly to increasing southness (Fig. 4).
Fig. 4 - Biplot relationships between fuel load and site predictors. Red dots: individual sites; black arrow: response variables (W1h: fine dead fuel with particle size < 6 mm; W10h: fine dead fuel with particle size 6-25 mm; W100h: fine dead fuel with particle size 25-75 mm; WGr: grass and grass live fuel < 6mm; WSh: shrub live fuel <25 mm); blue arrows: predictors (CanCov: canopy cover; Elev: elevation; Southness: -cos [aspect]; tt: thermotype in the bioclimate map of Italy according to [47], where increasing values correspond to decreasing temperatures; ot: ombrotype in the bioclimate map of Italy according to [47], where increasing values correspond to increasing aridity). Predictors were scaled to improve graphical representation.
Discussion
The fuel dataset deriving from this study provides quantitative characteristics of fuel beds in grasslands, shrublands, broadleaved and coniferous forests of the Alpine, temperate and Mediterranean regions of Italy. It includes information useful for estimates on fuel biomass, surface fire behavior and effects. Although the sample size was limited and unevenly spatially distributed across the Italian peninsula, the survey covered a broad range of bioclimatic regions and vegetation types. This is apparent from the associations established between the 19 fuelbeds and the European Forest Types ([10]), and the forest categories of the Italian National Forest Inventory ([37]). Although resulting from an a posteriori compilation of fuel observations from different surveys, the fuel dataset covers all the classes of forest, grassland and shrubland mapped in Italy by Corine Land Cover IV level ([38]).
In this Italian fuel dataset, the fuelbed with the lowest total surface fuel load was “Sparse and very short grasslands” with an average of 2.47 Mg ha-1. This was due to the dominance of very short grasses with a relatively low cover, which is typical of nutrient-poor and climatically limited sites such as those at high elevation or on very dry sites (fuelbed 1, Fig. S1 in Supplementary material). On the contrary, the fuelbed with the highest total surface fuel load (duff excluded) is “Aquatic marshes”. These are very flammable fuel complexes with high spatial continuity, a deep fuelbed (326 ± 19 cm on average), and dead fuels <25 mm representing 40% of total surface fuel load (fuelbed 19, Fig. S1 in Supplementary material).
Fuel characteristics of Italian vegetation fuels are within the range of values in the Fuel Characteristics Classification System (FCCS), one of the most extensive databases on fuel load and structure ([44]). Only fine fuel loads (<6 mm) were markedly lower than FCCS loads. This could be related to the higher variability of vegetation types included in FCCS (from tropical to boreal ecosystems), specific fuelbeds with very high biomass levels such as “White fir-giant sequoia-sugar pine forest”, and an older stage of development of North American forests ([18]), leading to higher productivity when compared to Italian forests. Fuel inventories carried out in similar fuelbeds displayed comparable fuel loads for both Alpine and Mediterranean shrublands. For example, Fréjaville et al. ([35]) reported a total fuel load (excluding duff) of 7.7-12.3 Mg ha-1 in Alpine mountainous forest categories, which falls within the range of 6.2 to 17.2 Mg ha-1 for fuelbeds 7 to 15 (Tab. 3), i.e., mountain forests under Alpine and temperate conditions. For Mediterranean shrublands, Dimitrakopoulos ([24]) reported a total fuel load in Kermes oak and tall evergreen sclerophylous shrubland (excluding duff and diameters > 7.5 cm) of 34.7 and 46.9 Mg ha-1, respectively, which falls within the range of fuelbed 6 “Tall Mediterranean shrublands and heathlands” of 16.8-53.3 Mg ha-1 (Tab. 2). Similarly, fuel load in Mediterranean grasslands according to Dimitrakopoulos ([24]) was 4.85 Mg ha-1, which approximates the mean total fuel load of 4.08 Mg ha-1 of fuelbed 2 “Continuous short grasslands”. Total fuel load in long-needled Mediterranean pine forests with sparse understory in Fernandes ([32]) ranged from 5.25 to 14.09 Mg ha-1, which is within the range of fuelbed 16 “Mediterranean long needled conifer litter” of 0.8-19.7 Mg ha-1. Furthermore, fuel loads in forest fuelbeds were similar to understory dry weight biomass values published by the Italian National Forest Inventory ([37]). Indeed, mean litter biomass in the INFC (i.e., leaves and twigs up to 25 mm) is 6.4 Mg ha-1 while in this fuel dataset the sum of W1h and W10h loads of forest fuelbeds (mean of fuelbeds 7 to 18) is 5.6 ± 0.21 Mg ha-1. Similarly, load of W100h downed woody material (25-75 mm) was 2.1 ± 0.19 Mg ha-1, which is close to INFC mean values for fine deadwood (25-94 mm), i.e., 1.8 Mg ha-1.
Conclusions
This study developed the first Italian vegetation fuel dataset based on 634 surface fuel complex observations collected within 337 study sites and covering the major types of ecosystems found in Italy. Overall, 19 fuelbeds were established, characterized by a set of fuel variables (41 fields), ranging from fuel component loads to the fuel type cover and depth. Although lacking the a priori sampling design needed for robust statistical inference, the fuelbed dataset presented in this study includes a broad set of fuel features that can be used for several modeling applications and decision support systems. For example, the range of fuel loadings can be used to calibrate Rothermel’s fire behavior fuel models by genetic algorithms ([7]) against observed rate of spread in a given fire environment to achieve realistic fire simulations by Rothermel’s based software and DSS ([59]). The available fuelbed data can be also used to estimate fuel consumption and emissions of greenhouse gases and particulate through the application of tools such as FOFEM ([50]) or CONSUME ([48]). Moreover, the fuel dataset provides ranges of carbon pools not yet available in the Italian National Forest Inventory such as grass and shrub biomass, despite their relevance for carbon inventories ([23]). Further analysis of this data could allow to calibrate allometry relationships between above ground and dead biomass pools ([31]) to broad the carbon stock estimates in forest inventories. Additional field surveys and national sampling efforts, along with a standardization of the sampling scheme/approach, are certainly needed to enhance reliability and accuracy of estimates in fuelbeds/fuel complexes currently less represented (i.e., fuelbed 12 - Eucaliptus litter; fuelbed 18 - Riparian vegetation) or affected by high variability due to the intrinsic heterogeneity of the vegetation and site conditions. Future research will also foresee the association of the fuelbed characteristics to specific non spatial (e.g., forest typologies) and spatial (e.g., forest types maps) products commonly used in natural resource management at the regional scale in Italy. This approach will provide cost-effective fuel related information and maps providing many advantages for land and resource management analysis, e.g., fuel hazard assessment, preventive fire management measures, strategic fire management planning, through the prioritization of areas and resource allocation for fuel treatment to mitigate potential fire danger, risk, and fire emission and model carbon-cycle and air quality. Finally, spatially-explicit information coupled with future climate ([16]) and land use change scenarios ([43]) would offer a valuable support towards more comprehensive landscape planning able to minimize risk related disturbances such as wildfires ([41]), as well as maximize ecosystem services provisioning and their trade-offs at the landscape level ([60]).
Acknowledgments
The study is the result of a research program carried out by the “Fire Management Working Group” of the Italian Society of Silviculture and Forest Ecology (⇒ https://sisef.org/category/gdl/incendi-boschivi).
References
Gscholar
Gscholar
Gscholar
Gscholar
CrossRef | Gscholar
Gscholar
Online | Gscholar
Gscholar
Gscholar
Gscholar
Gscholar
CrossRef | Gscholar
Supplementary Material

Tab. S2 - Foliage and branches fuel load and source of data by fuel complex.
Fig. S1 - Scheme showing the structure of the dataset as regards quantitative variables characterizing each fuel observation.
Fig. S2 - Photos describing the 19 fuelbeds included in the dataset.

Authors’ Info
Authors’ Affiliation
Simone Meytre
Costantino Sirca 0000-0001-7605-5700
Dept. of Agriculture, Forest and Food Sciences, University of Torino, l.go Paolo Braccini 4, Grugliasco, TO (Italy)
Costantino Sirca 0000-0001-7605-5700
Dept. of Agricultural and Environmental Science, University of Milano, v. Celoria 2, Milano (Italy)
Bachisio Arca 0000-0002-2834-2753
Grazia Pellizzaro 0000-0003-0860-897X
Michele Salis 0000-0002-0499-9726
Andrea Ventura 0000-0003-3120-0390
Institute of Bio-Economy, National Research Council, trav. La Crucca 3, Sassari (Italy)
Dept. for Innovation in Biological, Agro-food and Forest systems, University of Tuscia, v. S. Camillo de Lellis snc, VT (Italy)
Assunta Esposito 0000-0003-2937-7373
Dept. of Environmental, Biological and Pharmaceutical Sciences and Technologies, University of Campania “Luigi Vanvitelli”, v. Vivaldi 43, 81100 Caserta (Italy)
Paola Mairota 0000-0002-0989-419X
Dept. of Agricultural and Environmental Sciences, University of Bari “A. Moro”, v. Amendola 165/A, 70126 Bari (Italy)
Marco Marchetti 0000-0002-5275-5769
Marco Ottaviano 0000-0002-8891-8814
Lorenzo Sallustio 0000-0001-9204-5123
Dept. of Biosciences and Territory, University of Molise, c.da Fonte Lappone, Pesche, IS (Italy)
Nuoro Forestry School, Dept. of Agriculture, University of Sassari, v. Cristoforo Colombo 1, I-08100 Nuoro (Italy)
Dept. of Agriculture, Food, Environment and Forestry, University of Florence, v. S. Bonaventura 13, 50145 Firenze (Italy)
Direzione Protezione Civile e Polizia locale, Regione Veneto, Marghera, VE (Italy)
Valentina Bacciu 0000-0002-2361-1416
Fondazione Centro Euro-Mediterraneo sui Cambiamenti Climatici (CMCC) - IAFES Division, Sassari (Italy)
Independent researcher, Agricultural High School “Duca degli Abruzzi”, v. Merlin 1, Padova (Italy)
Corresponding author
Paper Info
Citation
Ascoli D, Vacchiano G, Scarpa C, Arca B, Barbati A, Battipaglia G, Elia M, Esposito A, Garfì V, Lovreglio R, Mairota P, Marchetti M, Marchi E, Meytre S, Ottaviano M, Pellizzaro G, Rizzolo R, Sallustio L, Salis M, Sirca C, Valese E, Ventura A, Bacciu V (2020). Harmonized dataset of surface fuels under Alpine, temperate and Mediterranean conditions in Italy. A synthesis supporting fire management. iForest 13: 513-522. - doi: 10.3832/ifor3587-013
Academic Editor
Francisco Lloret Maya
Paper history
Received: Jul 15, 2020
Accepted: Oct 26, 2020
First online: Nov 13, 2020
Publication Date: Dec 31, 2020
Publication Time: 0.60 months
Copyright Information
© SISEF - The Italian Society of Silviculture and Forest Ecology 2020
Open Access
This article is distributed under the terms of the Creative Commons Attribution-Non Commercial 4.0 International (https://creativecommons.org/licenses/by-nc/4.0/), which permits unrestricted use, distribution, and reproduction in any medium, provided you give appropriate credit to the original author(s) and the source, provide a link to the Creative Commons license, and indicate if changes were made.
Web Metrics
Breakdown by View Type
Article Usage
Total Article Views: 7434
(from publication date up to now)
Breakdown by View Type
HTML Page Views: 4616
Abstract Page Views: 537
PDF Downloads: 1829
Citation/Reference Downloads: 4
XML Downloads: 448
Web Metrics
Days since publication: 1352
Overall contacts: 7434
Avg. contacts per week: 38.49
Article Citations
Article citations are based on data periodically collected from the Clarivate Web of Science web site
(last update: Nov 2020)
(No citations were found up to date. Please come back later)
Publication Metrics
by Dimensions ©
Articles citing this article
List of the papers citing this article based on CrossRef Cited-by.
Related Contents
iForest Similar Articles
Research Articles
Historical fire ecology and its effect on vegetation dynamics of the Lagunas de Montebello National Park, Chiapas, México
vol. 14, pp. 548-559 (online: 01 December 2021)
Research Articles
Assessing the performance of fire danger indexes in a Mediterranean area
vol. 11, pp. 563-571 (online: 01 September 2018)
Research Articles
Wildfire risk and its perception in Kabylia (Algeria)
vol. 11, pp. 367-373 (online: 04 May 2018)
Research Articles
Differences of fire activity and their underlying factors among vegetation formations in Greece
vol. 6, pp. 132-140 (online: 08 April 2013)
Technical Notes
Goat grazing as a wildfire prevention tool: a basic review
vol. 7, pp. 260-268 (online: 26 March 2014)
Research Articles
The economic value of fire damages in Tuscan agroforestry areas
vol. 14, pp. 41-47 (online: 14 January 2021)
Review Papers
Prescribed burning in Italy: issues, advances and challenges
vol. 6, pp. 79-89 (online: 07 February 2013)
Short Communications
Upscaling the estimation of surface-fire rate of spread in maritime pine (Pinus pinaster Ait.) forest
vol. 7, pp. 123-125 (online: 13 January 2014)
Research Articles
Developing wildfire risk probability models for Eucalyptus globulus stands in Portugal
vol. 6, pp. 217-227 (online: 27 May 2013)
Research Articles
Analysis of factors influencing deployment of fire suppression resources in Spain using artificial neural networks
vol. 9, pp. 138-145 (online: 19 July 2015)
iForest Database Search
Search By Author
- D Ascoli
- G Vacchiano
- C Scarpa
- B Arca
- A Barbati
- G Battipaglia
- M Elia
- A Esposito
- V Garfì
- R Lovreglio
- P Mairota
- M Marchetti
- E Marchi
- S Meytre
- M Ottaviano
- G Pellizzaro
- R Rizzolo
- L Sallustio
- M Salis
- C Sirca
- E Valese
- A Ventura
- V Bacciu
Search By Keyword
Google Scholar Search
Citing Articles
Search By Author
- D Ascoli
- G Vacchiano
- C Scarpa
- B Arca
- A Barbati
- G Battipaglia
- M Elia
- A Esposito
- V Garfì
- R Lovreglio
- P Mairota
- M Marchetti
- E Marchi
- S Meytre
- M Ottaviano
- G Pellizzaro
- R Rizzolo
- L Sallustio
- M Salis
- C Sirca
- E Valese
- A Ventura
- V Bacciu
Search By Keywords
PubMed Search
Search By Author
- D Ascoli
- G Vacchiano
- C Scarpa
- B Arca
- A Barbati
- G Battipaglia
- M Elia
- A Esposito
- V Garfì
- R Lovreglio
- P Mairota
- M Marchetti
- E Marchi
- S Meytre
- M Ottaviano
- G Pellizzaro
- R Rizzolo
- L Sallustio
- M Salis
- C Sirca
- E Valese
- A Ventura
- V Bacciu
Search By Keyword