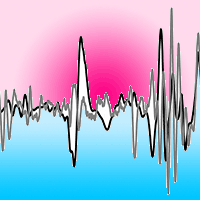
Estimation of total extractive content of wood from planted and native forests by near infrared spectroscopy
iForest - Biogeosciences and Forestry, Volume 14, Issue 1, Pages 18-25 (2021)
doi: https://doi.org/10.3832/ifor3472-013
Published: Jan 09, 2021 - Copyright © 2021 SISEF
Research Articles
Abstract
The aim of this study was to evaluate the performance of multivariate models using Near infrared (NIR) spectra for predicting total extractives content of solid and powdered wood of planted and native species from tropical savanna. NIR spectra were recorded on the milled wood and radial surface of solid wood specimens of Cedrela sp., Jacaranda sp., Apuleia sp., Aspidosperma sp. and clones of Eucalyptus hybrids via an integrating sphere and fiber optics probe. NIR spectral signatures were evaluated by Principal Component Analysis (PCA) and then associated to the total extractive content quantified by extraction in toluene/ethanol (2:1) solutions, pure ethanol and hot water by Partial Least Squares (PLS) regressions. PCA revealed that NIR spectra measured in solid wood by integrating sphere gave a better discrimination of wood species. A global PLS model was developed based on NIR obtained by integrating sphere with satisfactory estimations both for solid wood (R²cv= 0.87, RMSECV= 1.08%) and wood powder (R²cv= 0.85, RMSECV= 1.19%). An independent test-set validation was performed with 25% of the samples and yielded R²p= 0.93 and RMSEP= 0.95% (for solid wood) and R²p= 0.87 and RMSEP= 1.40% (for wood powder). Both models can be applied for rapid screenings, though models developed from NIR spectra by integrating sphere on solid wood are considered more suitable for rapid predictions of extractive content in unknown wood specimens.
Keywords
Lumber, Multivariate Analysis, Non-destructive Testing, Test Set Validation, Wood Chemistry
Introduction
The chemical composition of wood is complex. Wood tissues are composed of many chemical components that are unevenly distributed as a result of the anatomical structure ([24]). Different combinations of chemical compositions, resulting from the effects of the environment and/or genetics, are also responsible for differences among and within wood species ([29]). According to Pereira et al. ([14]) a large proportion of the variation found among specific wood samples is an expression of chemical characteristics and these greatly affect end use properties and material quality.
Wood structure has been described as an interpenetrating system of polymers comprising holocellulose and lignin ([2]). In addition to these structural components, wood is made up of non-structural organic and inorganic components grouped into two classes: extractives and mineral compounds ([14]). According to Pettersen ([16]), the presence of some of these extractives influences wood resistance to fungal and insect attack, coloration, odor, permeability, density and hardness of wood. The extractives can constitute 4% to 10% of the dry mass of temperate hardwood species and up to 20% in species from tropical climates ([16]). According to Foelkel ([4]) the most important extractives are terpenes and resin acids (both consisting of isoprene units) and polyphenols (flavonols, anthocyanins, quinones, lignans, and tannins). There is a great variation in the distribution of extractives throughout the wood of a tree: the sugars and other soluble constituents of the sap as well as food reserves (starch and fats) are found in the sapwood, while the phenolic substances are normally deposited in the heartwood ([14]). The benzene alcohol extractives are often higher in heartwood while the sapwood has a higher acidic/pH ([10]).
Although the extractives contribute to only a small percentage of the wood composition, the solubility of extractives in wood has importance regarding its properties and, therefore, in industrial applications ([21]). The extractives have, for example, a significantly negative effect on the pulping and bleaching process of cellulosic pulp ([6]) and their oxidation tends to increase the acidity of the wood, thereby promoting its degradation ([21]). According to Várhegyi et al. ([28]), the removal of some extractives decreases the fixed carbon content, reducing the charcoal yield and shifting the thermo-gravimetric curve to higher temperatures. However, the extracts contained in the wood of forest species may have potential for nutraceutical applications ([20]), as antioxidants ([3]) and antifungal/antimicrobial agents ([11]). Thus, investigating the role of wood extractives is critical for many industrial applications.
The traditional method to determine the extractive content of wood is time-consuming, costly, and operationally inappropriate for large sample quantities. There is no universal solvent that removes all substances; each solvent is selective for one or more classes of extractives, thus the use of a sequence of solvents is needed. In general, an extraction sequence initiated by the ethanol/toluene mixture, followed by ethanol and finished by hot water is used to quantify the extractives ([13]). Therefore, the development of new solutions for chemical characterization of wood, preferably being fast and economical, is important for industrial use of wood. Near infrared (NIR) spectroscopy presents great potential for the evaluation of several materials, such as polymers, textiles, pharmaceuticals and petrochemicals ([26]). Many studies have shown that NIR spectroscopy can be applied to evaluate the chemical, physical, mechanical and anatomical properties of wood ([25]).
In regard to the NIR based models for wood extractives of softwoods, Gierlinger et al. ([5]) developed models to estimate the extractive content of the wood core of Larix sp. in acetone and hot water by means of powdered wood and solid wood and reported that spectra of powdered wood generated more accurate models. Uner et al. ([27]) evaluated the extractive and lignin contents in the Pinus brutia wood powder by NIR spectroscopy and presented models whose standard error of prediction ranged from 0.26% to 1.85% for the extractive content. He & Hu ([6]) applied the NIR spectroscopy technique to estimate lignin and extractive content of various coniferous and hardwood species and presented satisfactory models for the prediction of extractive content in ethanol/benzene (R² = 0.96) and cross-validation error of 0.18%.
NIR models for extractive content of hardwoods also are reported in the literature. Silva et al. ([22]) developed NIR models to estimate the total content of phenolic compounds and extractives soluble in hot water in mahogany, with a calibration error of 0.46% and cross-validation of 0.63%. Poke et al. ([17]) studied the extractive content of Eucalyptus globulus wood in methanol with the NIR spectra measured in wood powder and reported that the calibration error was 0.56%. Later, Poke & Raymond ([18]) verified that the existing calibrations from wood powder were inadequate for the prediction of extractive content from spectra measured in solid wood. Li & Altaner ([8]) have associated NIR data with the extractives content determined by accelerated solvent extraction (ASE) of Eucalyptus bosistoana, E. globoidea and E. argophloia wood. They generated NIR-based models able to predict extractives content with errors from 0.34% to 18.9% and a residual mean square error (RMSE) of 0.9%.
The above studies demonstrated the potential of spectroscopy in estimating the extractive content in several species of wood using several solvents. However, the influence of the sample preparation (solid or powdered wood) on the fit of the predictive models for extractive content is still not clear. Thus, the first objective of this study was to develop predictive models to monitor the total extractive content of planted and natural forest wood from NIR spectra measured in solid wood and powder, and validate them using independent sets. Moreover, the study also aimed to verify whether NIR models developed for wood specimens from planted forests can predict the extractive content of wood specimens from natural forest species.
Material and methods
Plant material
The wood samples used in this study were collected from different forest species from the Cerrado (tropical savanna) and commercial plantation biomes in Brazil. The Cerrado species (21° 10′ S, 44° 54′ W) are: Cedrela sp. (Cedar), Jacaranda sp. (Jacaranda), Apuleia sp. (Garapa) and Aspidosperma sp. (Peroba). These species were chosen because they stand out among the native woods of Brazil used commercially. The woods were obtained from sawmills and lumber companies in July 2015. The commercial plantation species are represented by clones of Eucalyptus grandis × E. urophylla hybrids from two forestry companies: Vallourec Florestal Ltda plantations (19° 16′ S, 44° 24′ W) which focuses on charcoal production (clones of 6.5 years) and Cenibra SA plantations (19° 17′ S, 42° 23′ W) focused on cellulose production (clones of 6 years).
Sample preparation and total extractives determination
For each plant material, six specimens with dimensions of approximately 35 × 35 × 100 mm (R × T × L) representing native and commercial plantation woods, were prepared as described by Ramalho et al. ([19]), totaling 36 specimens. The specimens were taken from central planks and without shell. The specimens were processed using a planing machine and the shavings produced was ground in a Wiley Mill® (Thomas Scientific, LLC, Swedesboro, NJ, USA) fitted with a 2 mm screen. The powdered wood was classified by means of 40 and 60 mesh screen sets. Total material extraction was performed on the sawdust that passed through the 40 mesh sieve and was retained in the 60 mesh sieve.
For the determination of the total extractives of the wood, the technical standard NBR 14853 ([1]) was used. In the process of total sample extraction, 2.00 g of wood dry sawdust, previously classified in the 40 and 60 mesh sieves, and the following sequence of solvents were used: 150 ml of the toluene/ethanol mixture (2:1) for five hours, 150 ml of ethanol for four hours and hot water for two hours. Different flasks were used for each solvent sequence. The process was carried out in duplicate using heating plates equipped with a soxhlet extraction system. The temperature of heating plates was kept slightly below the boiling temperature of the solvents, around 80 °C. In the soxhlet extraction system, the sample was placed in a porous crucible that was gradually filled with the solvent. When the liquid reached the overflow level, a siphon aspirated the solute and discharged it back into the distillation flask, thus transporting the extracts. This operation was repeated until the extraction was completed.
After the extraction process, the porous crucibles with extractive-free wood powder were placed in an oven at 103 ± 2 °C until reaching constant mass, and were later weighed to determine their dry mass. The total extractive content of the samples was then quantified by the difference between the dry mass of the material before and after extraction. The volatile organic compounds possibly emitted as gases during the drying were not taken into account in the calculations.
NIR spectra acquisition
NIR signatures were recorded initially in solid wood and, after processing the material, in the powdered wood before the extraction process. An MPA FT-NIR® spectrometer (Bruker Optik GmbH, Ettlingen, Germany) was used to acquire the NIR spectra, which is a Fourier transform (FT) based spectrometer equipped with an integrating sphere and an optical fiber. The software OPUS ver. 7.5 (Bruker) was used for data storage.
NIR signatures of solid wood
NIR signatures of solid wood were recorded directly on the radial face (transition between core and sapwood) of the specimens, in an air-conditioned room, using the integrating sphere (Fig. 1a), by diffuse reflection mode, in the acquisition range of 12,500 to 3,500 cm-1 and with a spectral resolution of 8 cm-1. Each spectrum represents an average of 32 scans. For modeling, only the NIR range between 9,000 and 4,000 cm-1 was considered. NIR spectra were taken only in the radial surface, because a spectrum on the tangential surface portrays the chemical information of a short period of the tree growth, as the radiation penetrates a few millimeters in one growth ring, while on the radial surface the light will interact with material developed during several months or years of growth.
Fig. 1 - Acquisition of spectra with NIR spectrometer (b) in solid wood using integrating sphere (a and c) and powder wood using optical fiber (c).
NIR signatures of powder wood
The same procedure and equipment for acquisition of the solid wood spectra was used for recording the spectra in the powdered wood, but the two acquisition paths were used: integrating sphere and optical fiber (Fig. 1c). Spectra of powdered wood were recorded with the material at equilibrium moisture (12%) and oven dried (0%).
Multivariate statistics
Principal Component Analysis (PCA) and Partial Least Square Regression (PLS) analyses were conducted using the free software Chemoface ver. 1.61 ([12]). The analyses were carried out both on the raw spectra (untreated) and after mathematical pre-treatment (first derivative).
PCA was used to verify the spectral similarity of the different samples of the species used, both from solid wood and powdered wood spectra. The PLS regression was used to correlate the NIR spectral data (independent variables) and the extractive content obtained by wet-chemistry analyses (dependent variables). NIR spectra obtained by the two acquisition pathways (integrating sphere and optical fiber) on solid wood and powdered wood at equilibrium moisture were used for these analyses.
Calibration models were cross-validated by leave-one-out and external set validation methods. The external validation was carried out using two different approaches: first, PLS models were developed with 75% of the randomly chosen specimens and validated with the remaining 25%. In the second step, the models were calibrated using data from five species and the validation was done against the sixth species (not included in the calibrated model). This procedure was repeated six times, for the six plant materials.
The models were developed with eight latent variables (LV). Preliminary tests indicated that eight LVs minimized the root mean standard error (RMSE) and maximized the coefficient of determination (R²). No samples were considered outliers. The criteria for selection of the prediction models were the values of coefficient of determination of the cross-validation leave-one-out (R²cv) and for prediction (R²p), root mean standard error of the cross-validation (RMSECV) and ratio performance deviation (RPD).
Results and discussion
The natural species and the Eucalyptus hybrids investigated in the present study were chosen to verify whether chemical similarity between wood from natural and planted forests can be a barrier for developing NIR models aimed at discriminating the origin of wood (natural and commercial plantations). Ramalho et al. ([19]) implemented this approach for rapid discrimination of wood type that has been transported and sold illegally in many countries, and reported promising findings. The present study was carried out within the framework of this major project.
Determination of total extractives content
The descriptive statistics of the values of total extractives determined by traditional laboratory method for each plant material which was used as reference for model calibration are shown in Tab. 1.
Tab. 1 - Descriptive statistics of the total content of extractives from different wood species determined by solvent sequence. (CV): coefficient of variation; (N): sample intensity.
Plant material | Common name |
Abbr. | Mean(%) | Max (%) |
Min (%) |
CV (%) |
N |
---|---|---|---|---|---|---|---|
Eucalyptus (Cenibra) | Eucalypt | EC | 4.25 | 6.85 | 1.75 | 39.32 | 12 |
Eucalyptus (Vallourec) | Eucalypt | EV | 5.04 | 6.41 | 3.80 | 19.82 | 12 |
Cedrela sp. | Cedar | C | 7.03 | 9.33 | 5.94 | 15.35 | 12 |
Apuleia sp. | Garapa | G | 3.66 | 4.18 | 3.20 | 8.39 | 12 |
Jacaranda sp. | Jacaranda | J | 9.46 | 11.77 | 6.69 | 19.66 | 12 |
Aspidosperma sp. | Peroba | P | 10.99 | 14.25 | 8.04 | 18.49 | 12 |
Aspidosperma sp. presented the highest total extractive content, with an average of 10.99%, followed by Apuleia sp. and Eucalyptus (EC and EV) with an average of 4.25%, 5.04% and 3.66%, respectively (Tab. 1). Eucalyptus specimens (EC) presented the greatest variation among the values of its samples with a coefficient of variation of 39.32%. The lowest variation was observed for Apuleia sp., with an 8.39% coefficient of variation, and also presented the lowest average extractive content. Pereira et al. ([15]) found 4.7% to 4.97% of extractives for Eucalyptus urophylla and 4.15% for Eucalyptus grandis wood at 7.5 years. Hsing et al. ([7]) obtained extractive values between 5.89% and 7.75% for clones of Eucalyptus grandis × E. urophylla at 2.5 years of age, which are higher than those obtained in this study; however, the hybrids had different ages.
NIR Spectra
The mean NIR spectra obtained by diffuse reflection of solid wood through the integrating sphere and of the powdered wood obtained by the two acquisition pathways of the equipment are shown in Fig. 2. The NIR signature from 9000 to 4000 cm-1 was used for models because this range was more informative.
Fig. 2 - Mean spectra untreated (a) and treated by second derivative (b), collected in solid wood through the integrating sphere and in powder wood through the two acquisition pathways of the equipment. (C): Cedrela sp.; (EC): Eucalyptus C; (EV): Eucalyptus V; (J): Jacaranda sp.; (P): Aspidosperma sp.; (G): Apuleia sp.
The most prominent absorption bands in all plant materials are approximately at 7000 and 5100 cm-1 (Fig. 2). These bands are consistent with the results of Silva et al. ([22]) who observed higher absorption at wavelengths of approximately 1410 and 1900 nm and attributed these absorption peaks to the O-H bonds and O-H and C=O bands combinations, respectively.
Principal Component Analysis (PCA)
PCA was carried out using the raw spectra obtained by integrating sphere from solid wood and powdered wood (Fig. 3), to obtain a preliminary classification of the species used according to its chemical properties, in which the extractive content of the latter is highlighted.
Fig. 3 - Results of the principal components analysis of NIR spectra obtained in solid wood (a) and wood powder (b) through the integrating sphere. (C): Cedrela sp.; (EC): Eucalyptus C; (EV): Eucalyptus V; (J): Jacaranda sp.; (P): Aspidosperma sp.; (G): Apuleia sp.
The contribution of each principal component in the spectral variance obtained in the two approaches (solid and powder) is presented in the graph axis of Fig. 3. Principal components 1 and 2, for all analyses, accounted for more than 99% of the variation. The principal components (PCs) help in the identification and interpretation of the dependencies that exist among the variables and, also, in the examination of the relationships that can exist among the individuals ([23]).
The PCA scores resulted in better classification, as regards the grouping (dashed groups) according to the species, when using the spectra obtained through the solid wood (Fig. 3a). When the spectra obtained using the powdered wood were used a larger overlap of some samples occurred. Thus, a full separation of each species based on extractive content data was not possible, although the samples from Eucalyptus differed from the grouping (dashed groups) of the native woods, except for the cedar (C) (Fig. 3b). The overlap between the Eucalyptus (EC and EV) specimens was expected as they belong to the same genus, though from different locations, and therefore they show a high chemical similarity. On the other hand, the species Garapa (G) was not well classified in any analysis, overlapping several species and showing no classification trend.
Overall, a better discrimination between species was obtained when PCA was performed on solid samples than on wood powder data. A possible explanation is that the spectrum measured from solid wood contains physical information from the wood specimens, in addition to variations in their chemical composition. Spectra capture variations due to wood texture, color, brightness, surface quality, etc. On the other hand, the physical component effect is canceled out in the wood powder spectra. A possible physical effect on the wood powder spectra could be the response to the particle size, though in this study the particle dimension was kept constant (40 to 60 mesh) for all wood samples.
The PCA loadings from untreated (Fig. 4a) and treated (Fig. 4b) NIR spectra are presented to help interpreting spectral bands.
Global PLS model for extractive content
Partial Least Squares (PLS) regression analysis was performed with the aim of obtaining global models for the estimation of total extractive content of the wood using both original spectra and those treated by the first derivative method. The results of these analyses are summarized in Tab. 2.
Tab. 2 - Global calibrations and cross-validations to estimate the total extractive content of wood from NIR spectra. (Treat): mathematical treatment; (“-“): original data; (1d): first derivative; (R²c): coefficient of determination for calibration; (RMSEC): mean square error for calibration (%); (R²cv): coefficient of determination for cross validation; (RMSECV): mean square error for cross validation (%); (RPD): deviation to performance ratio; (EMC): equilibrium moisture content; (0%): 0% moisture.
Model | Via | Processing | Moisture | Treat | R²c | RMSEC | R²cv | RMSECV | RPD |
---|---|---|---|---|---|---|---|---|---|
1 | Sphere | Solid | EMC | - | 0.91 | 0.90 | 0.87 | 1.08 | 2.83 |
2 | Sphere | Solid | EMC | 1d | 0.91 | 0.90 | 0.84 | 1.20 | 2.55 |
3 | Sphere | Powder | EMC | - | 0.90 | 0.95 | 0.85 | 1.19 | 2.57 |
4 | Sphere | Powder | EMC | 1d | 0.87 | 1.11 | 0.81 | 1.34 | 2.30 |
5 | Sphere | Powder | 0% | - | 0.81 | 1.32 | 0.72 | 1.63 | 1.89 |
6 | Sphere | Powder | 0% | 1d | 0.84 | 1.21 | 0.72 | 1.63 | 1.89 |
7 | Fiber | Powder | EMC | - | 0.91 | 0.90 | 0.71 | 1.65 | 1.85 |
8 | Fiber | Powder | EMC | 1d | 0.72 | 1.61 | 0.39 | 2.48 | 1.24 |
9 | Fiber | Powder | 0% | - | 0.86 | 1.14 | 0.61 | 1.96 | 1.57 |
10 | Fiber | Powder | 0% | 1d | 0.77 | 1.45 | 0.53 | 2.14 | 1.43 |
In general, the application of the first derivative pretreatment was not efficient in estimating the extractive content of the wood, since all the models presented lower calibration and validation results when submitted to such method (Tab. 2).
All the models based on spectra obtained from integrating sphere had better statistics when compared to the models generated by optical fiber, as seen for Model 3 (sphere) and Model 7 (fiber), which had respectively R²cv values of 0.85 and 0.71, RMSECV values of 1.19% and 1.65% and an RPD of 2.57 and 1.85. Therefore, the integrating sphere is more suitable for the acquisition of spectra when the aim is to estimate the extractive content of the wood. It is important to note that this acquisition path presents a surface area approximately 100 times larger than the surface area of a fiber-optic probe.
Most of the models generated from wood spectra at 0% moisture presented statistics well below the models generated from wood spectra at equilibrium moisture (moisture of approximately 12% - Tab. 2). This could happen because in wet wood the incident light penetrates deeper, capturing more information. In addition, the spectra presented absorption peaks at wave numbers that are assigned to the O-H bonds (Fig. 2), so the presence of water may be the reason of the best results obtained using wood in equilibrium moisture. Also, this facilitates the process and lowers its cost, as the drying step prior to analysis is no longer necessary.
The most robust statistics in estimating extractive content from wood were obtained using Model 1 (R²cv = 0.87, RMSECV = 1.08%) which is based on spectra acquired through integrating sphere and solid wood at equilibrium moisture. Model 3 (R²cv = 0.85. RMSECV = 1.19%) was based on NIR of wood powder at equilibrium moisture. Despite the difference in the associated statistics, the two models presented satisfactory results and could be used successfully.
Independent random batch validation
In the first independent validation, 75% of the samples were used for the calibration of the models while the remaining 25% were used for their validation. For this, we used original spectral data without mathematical treatments. The results of these analyses are summarized in Tab. 3.
Tab. 3 - External calibrations and validations performed from 25% of the samples, using spectral data obtained from the sphere and fiber pathways. (R²cv): coefficient of determination for cross validation; (RMSECV): mean square error for cross validation (%); (R²p): coefficient of determination for prediction; (RMSEP): mean square error for prediction (%); (RPD): deviation to performance ratio; (EMC): equilibrium moisture content; (0%): 0% moisture.
Model | Via | Processing | Moisture | R²cv | RMSECV | R²p | RMSEP | RPD |
---|---|---|---|---|---|---|---|---|
11 | Sphere | Solid | EMC | 0.84 | 1.17 | 0.93 | 0.95 | 3.10 |
12 | Sphere | Powder | EMC | 0.84 | 1.16 | 0.87 | 1.40 | 2.12 |
13 | Sphere | Powder | 0% | 0.68 | 1.68 | 0.67 | 1.93 | 1.53 |
14 | Fiber | Powder | EMC | 0.74 | 1.51 | 0.64 | 2.08 | 1.42 |
15 | Fiber | Powder | 0% | 0.67 | 1.71 | 0.58 | 2.19 | 1.35 |
Again, the integrating sphere showed better performance, as indicated by the statistics related to the generated models (Tab. 3). In most cases, the R²p values were superior to the R²cv values, showing the good performance of the validation. However, models based on spectra recorded by fiber-optic probe did not present this behavior.
Better predictions were obtained by spectra measured in solid wood (model 11: R²p = 0.93, RMSEP = 0.95%) when compared to spectra from ground wood (model 12: R²p = 0.87, RMSEP = 1.40%). However, in both cases these models can be considered promising tools for the prediction of total extractive contents of unknown wood.
Fig. 5 presents the relationship between the extractive content of the wood determined in the laboratory and that predicted by NIR analysis. Fig. 5a depicts the results obtained by Model 3 (Tab. 2) using powdered wood and cross-validation, while Fig. 5b shows the results of Model 1 (Tab. 2) generated from the spectra of solid wood measured by the integrating sphere. Also shown is the relationship between observed and predicted values of extractive content based on spectra from wood powder (Fig. 5c) and solid wood (Fig. 5d) after validation.
Fig. 5 - Graph of calibrations and cross and external validations with spectral data obtained from the integrating sphere in powdered wood (a and c) and solid wood (b and d).
A strong correlation between the values predicted by NIR analysis and those measured by wet-chemistry in laboratory was detected both using calibration and external validation. Correlation was higher for spectra recorded by integrating sphere on wood at equilibrium moisture. Regarding the use of wood in the solid or powder condition, a slight improvement in the correlation was observed for solid wood.
The lower dispersion of the predicted values observed for planted species as compared to native species confirmed their lower variability in extractive content (Fig. 5a, Fig. 5b).
Gierlinger et al. ([5]), Poke & Raymond ([18]) and Li & Altaner ([9]) have compared the use of solid wood and powdered wood in the estimation of extractive contents using NIR spectroscopy. Contrary to the results in Tab. 2, the authors reported more robust results using spectra from wood powder. Gierlinger et al. ([5]) studied the species Larix sp. with two different solvents for extraction. They reported promising models for predicting extractives in hot water from spectra taken on wood powder (R²cv = 0.96, RMSECV = 0.86%) and solid wood (R2c = 0.81, RMSECV = 1.85%). For extractives in acetone, the models presented lower statistics: R²cv = 0.85 and RMSECV = 0.32% for wood powder and R²cv = 0.70 and RMSECV = 0.43% for solid wood. Poke & Raymond ([18]) studied Eucalyptus globulus wood extractives in methanol and reported values of R²cv = 0.89 and RMSECV = 0.56% (powder wood) and R²cv = 0.87 and RMSECV = 1.37% (solid wood). Li & Altaner ([9]) used NIR analysis for assessing the extractive content of Eucalyptus bosistoana from extraction in ethanol solution, reporting values of R²cv = 0.94 and RMSECV = 0.87% for wood powder and R²cv = 0.87 and RMSECV = 1.27% for solid wood.
He & Hu ([6]) applied NIR spectroscopy for the prediction of the extractive content of several wood species, including coniferous and hardwood, and reported RMSEP = 0.403% when extracted with sodium hydroxide and RMSEP = 0.206% when extracted in ethanol/benzene. Uner et al. ([27]) investigated wood powder of Pinus brutia and presented models for extractive content with a RMSEP varying between 0.26% and 1.85%.
Gierlinger et al. ([5]) investigated wood in both conditions (solid and powder) and obtained results contrary to the findings of this study. They found more robust prediction models using wood powder (R²p = 0.84, RMSEP = 0.30%) in comparison to solid wood (R²p = 0.78, RMSEP = 0.36%). However, both models presented close values and could be successfully applied for estimating extractive content of unknown wood.
The models used in this study had close and robust statistics and yielded satisfactory predictions of the extractive content of unknown wood samples. Based on our results, the models developed from NIR signatures taken on solid wood presented more robust statistics, probably because these are based on data from several different species, while the above-mentioned studies were done using a single species. Li & Altaner ([8]) also found better results for models including data from mixed species rather than from single species, both for calibration and validation, using spectra collected by optical fiber probe in powdered wood. In addition, the above authors used a single solvent for the extraction, while in the present study the models were developed to estimate total extraction using a sequence of solvents.
Independent validation by species
To assess the model accuracy in predicting the extractive content from unknown wood, calibrated models based on five species were validated against a different species not included in the calibration step. The analyses were carried out in three different steps: (i) from NIR spectra recorded with integrating sphere from solid wood; (ii) from NIR spectra recorded with integrating sphere from powdered wood; and (iii) from NIR spectra recorded with fiber-optic probe in milled wood. Tab. 4 shows the statistics for each model in each of the three steps described above.
Tab. 4 - Calibrations and external validations made from only one species using spectral data obtained from spectra recorded with integrating sphere and fiber-optic probe. (R²cv): coefficient of determination for cross validation; (RMSECV): mean square error for cross validation (%); (R²p): coefficient of determination for prediction; (RMSEP): mean square error for prediction (%); (RPD): deviation to performance ratio. (C): Cedrela sp.; (EC): Eucalyptus C; (EV): Eucalyptus V; (J): Jacaranda sp.; (P): Aspidosperma sp.; (G): Apuleia sp.
Model | Via / Processing |
Validation set |
R²cv | RMSECV | R²p | RMSEP | RPD |
---|---|---|---|---|---|---|---|
16 | Sphere / Solid | EC | 0.88 | 1.04 | 0.34 | 1.52 | 2.00 |
17 | EV | 0.87 | 1.16 | 0.63 | 0.80 | 4.02 | |
18 | G | 0.81 | 1.29 | 0.31 | 2.23 | 1.35 | |
19 | C | 0.86 | 1.22 | 0.66 | 3.12 | 1.07 | |
20 | J | 0.89 | 0.99 | 0.36 | 5.87 | 0.51 | |
21 | P | 0.84 | 0.98 | 0.74 | 4.81 | 0.52 | |
22 | Sphere / Powder | EC | 0.83 | 1.25 | 0.66 | 1.04 | 2.93 |
23 | EV | 0.84 | 1.29 | 0.44 | 1.20 | 2.69 | |
24 | G | 0.80 | 1.34 | 0.17 | 7.24 | 0.41 | |
25 | C | 0.86 | 1.24 | 0.001 | 1.78 | 1.87 | |
26 | J | 0.85 | 1.15 | 0.67 | 6.21 | 0.48 | |
27 | P | 0.81 | 1.08 | 0.57 | 5.46 | 0.45 | |
28 | Fiber / Powder | EC | 0.68 | 1.73 | 0.01 | 1.82 | 1.67 |
29 | EV | 0.73 | 1.67 | 0.002 | 1.57 | 2.06 | |
30 | G | 0.72 | 1.58 | 0.002 | 3.00 | 1.00 | |
31 | C | 0.71 | 1.79 | 0.05 | 1.88 | 1.77 | |
32 | J | 0.70 | 1.63 | 0.44 | 5.65 | 0.53 | |
33 | P | 0.62 | 1.56 | 0.39 | 6.63 | 0.37 |
Tab. 4 shows that most of the models presented a satisfactory calibration using only five species. The best results in the validation step were found using solid wood spectra obtained through integrating sphere. The best efficacy was observed using the model validated by EV (R²p = 0.63, RMSEP = 0.80%). The models validated by the species C (R²p = 0.66, RMSEP = 3.12%) and species P (R²p = 0.74, RMSEP = 4.81%) were also satisfactory for rapid screenings but presented a prediction error considered high for extractive content.
Considering the powdered wood spectra, the highest prediction coefficient and lowest prediction error was obtained using the model validated by the EC species (R²p = 0.66, RMSEP = 1.04%). The models validated by species J (R²p = 0.67, RMSEP = 6.21%) and P (R²p = 0.57, RMSEP = 5.46%), though presenting good prediction coefficients, had errors considered too high.
The models based on spectra obtained through fiber-optic probe did not yield satisfactory results, with low prediction coefficients and/or high prediction errors. Fig. 6 shows the calibration and validation graphs for each of the models generated from the solid wood spectra.
Fig. 6 - Graphs of calibrations and external validations from a species with spectral data obtained from the solid wood by the integrating sphere acquisition path. (C): Cedrela sp.; (EC): Eucalyptus C; (EV): Eucalyptus V; (J): Jacaranda sp.; (P): Aspidosperma sp.; (G): Apuleia sp.
The graphs in Fig. 6 showed a good distribution of the calibration samples and a curious distribution for the validation samples, the latter being in some cases quite distant from the trend line of the calibration samples. The best distribution of the validation samples in the three sets of graphs is observed using the planted materials (EC and EV). The explanation for these results lies in the fact that when one of the Eucalyptus clone materials is used to validate the models, consequently the other clone material was part of the calibration model. Since they belong to the same species and have great chemical similarity, this led to achieve the best results in the validation step.
Concluding remarks
NIR spectroscopy proved to be an efficient and fast technique for estimating the total extractive content of wood. For this purpose, models from spectra acquired by the two acquisition pathways of the equipment can be used. However, the acquisition by integrating sphere showed more robust results in different cases.
The preparation of samples (solid wood or powder wood) affects the acquired spectra and therefore the final results. In this study, models from spectra drawn from solid wood had relatively better results when compared to models from spectra from wood powder. However, in both cases the results were considered satisfactory and the models could be successfully applied to estimate the extractive content of the wood.
Based on PCA results, it is concluded that the NIR is sensitive to the chemical variations of the woods investigated in this study. Although a classification of the species based on extract contents was not fully evident in several cases, a tendency towards the discrimination among species based on such data could be noticed.
The external validation of the NIR models using 25% of the samples to validate the calibration batch presented satisfactory results, as well as their validation using an extra species. In spite of the good predictions obtained in the majority of cases, errors considered high for the content of extractives were observed.
Acknowledgements
The authors express special thanks to the Wood Science and Technology Laboratory of the Federal University of Lavras (UFLA, Brazil), to CNPQ, FAPEMIG and CAPES for supporting the experimental work. This work was supported by the National Council for Scientific and Technological Development (CNPq, Brazil. grant no. 405085/2016-8), the Higher Education Personnel Improvement Coordination (CAPES, Brazil) and Foundation for Research Support of the State of Minas Gerais (FAPEMIG, Brazil). PRG Hein was supported by CNPq (grant no. 303675/2017-9) grants.
References
Gscholar
Gscholar
Gscholar
CrossRef | Gscholar
Gscholar
Gscholar
Gscholar
Gscholar
Gscholar
Authors’ Info
Authors’ Affiliation
Fernanda Maria Guedes Ramalho 0000-0001-5701-4479
Paulo Fernando Trugilho 0000-0002-6230-5462
Paulo Ricardo Gherardi Hein 0000-0002-9152-6803
Departamento de Ciências Florestais, DCF, Universidade Federal de Lavras, CEP 37200-000 Lavras, MG (Brazil)
Corresponding author
Paper Info
Citation
Teixeira Mancini L, Guedes Ramalho FM, Trugilho PF, Gherardi Hein PR (2021). Estimation of total extractive content of wood from planted and native forests by near infrared spectroscopy. iForest 14: 18-25. - doi: 10.3832/ifor3472-013
Academic Editor
Giacomo Goli
Paper history
Received: Apr 15, 2020
Accepted: Nov 06, 2020
First online: Jan 09, 2021
Publication Date: Feb 28, 2021
Publication Time: 2.13 months
Copyright Information
© SISEF - The Italian Society of Silviculture and Forest Ecology 2021
Open Access
This article is distributed under the terms of the Creative Commons Attribution-Non Commercial 4.0 International (https://creativecommons.org/licenses/by-nc/4.0/), which permits unrestricted use, distribution, and reproduction in any medium, provided you give appropriate credit to the original author(s) and the source, provide a link to the Creative Commons license, and indicate if changes were made.
Web Metrics
Breakdown by View Type
Article Usage
Total Article Views: 6363
(from publication date up to now)
Breakdown by View Type
HTML Page Views: 4436
Abstract Page Views: 444
PDF Downloads: 1296
Citation/Reference Downloads: 5
XML Downloads: 182
Web Metrics
Days since publication: 1295
Overall contacts: 6363
Avg. contacts per week: 34.39
Article Citations
Article citations are based on data periodically collected from the Clarivate Web of Science web site
(last update: Nov 2020)
(No citations were found up to date. Please come back later)
Publication Metrics
by Dimensions ©
Articles citing this article
List of the papers citing this article based on CrossRef Cited-by.
Related Contents
iForest Similar Articles
Research Articles
NIR-based models for estimating selected physical and chemical wood properties from fast-growing plantations
vol. 15, pp. 372-380 (online: 05 October 2022)
Research Articles
Mechanical and physical properties of Cunninghamia lanceolata wood decayed by brown rot
vol. 12, pp. 317-322 (online: 06 June 2019)
Research Articles
Acoustic evaluation of wood quality with a non-destructive method in standing trees: a first survey in Italy
vol. 10, pp. 700-706 (online: 17 July 2017)
Research Articles
Characterization of technological properties of matá-matá wood (Eschweilera coriacea [DC.] S.A. Mori, E. odora Poepp. [Miers] and E. truncata A.C. Sm.) by Near Infrared Spectroscopy
vol. 14, pp. 400-407 (online: 01 September 2021)
Research Articles
Examining the evolution and convergence of wood modification and environmental impact assessment in research
vol. 10, pp. 879-885 (online: 06 November 2017)
Research Articles
Characterization of VOC emission profile of different wood species during moisture cycles
vol. 10, pp. 576-584 (online: 08 May 2017)
Research Articles
Improving sustainability in wood coating: testing lignin and cellulose nanocrystals as additives to commercial acrylic wood coatings for bio-building
vol. 14, pp. 499-507 (online: 11 November 2021)
Research Articles
Identification of wood from the Amazon by characteristics of Haralick and Neural Network: image segmentation and polishing of the surface
vol. 15, pp. 234-239 (online: 14 July 2022)
Research Articles
Density, extractives and decay resistance variabilities within branch wood from four agroforestry hardwood species
vol. 14, pp. 212-220 (online: 02 May 2021)
Research Articles
Physical, chemical and mechanical properties of Pinus sylvestris wood at five sites in Portugal
vol. 10, pp. 669-679 (online: 11 July 2017)
iForest Database Search
Search By Author
Search By Keyword
Google Scholar Search
Citing Articles
Search By Author
Search By Keywords
PubMed Search
Search By Author
Search By Keyword