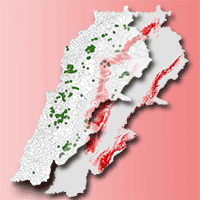
Local ecological niche modelling to provide suitability maps for 27 forest tree species in edge conditions
iForest - Biogeosciences and Forestry, Volume 13, Issue 3, Pages 230-237 (2020)
doi: https://doi.org/10.3832/ifor3331-013
Published: Jun 19, 2020 - Copyright © 2020 SISEF
Research Articles
Abstract
Ecological Niche Modelling (ENM) portrays the relationship between the actual geographical distribution of a species and the environmental factors that induced this distribution. Yet most models study species over the wider range of their distribution; thus, they are rarely appropriate for forest management and forest restoration on the local scale. This study aims to understand the major environmental factors affecting the distribution of 27 species, through limiting ENM at national level (Lebanon). MaxENT software was used for modelling. Area under the curve (AUC) values showed a very good robustness of the models. Minimal biogeographic and climatic parameters such as elevation, distance from the sea, annual mean precipitation, the average minimum temperature of the coldest month, the average maximum temperature of the warmest month, and Emberger Quotient were sufficient to obtain robust modelling results. Cloud coverage during summer was identified as a novelty factor explaining species distribution at the edge of their range. Composite soil and topography predictors such as Potential Direct Incident Radiation (PDIR) and the Integrated Moisture Index (IMI) were reduced to simple factors such as aspect, slope and available water content, whose contribution was conditioned to higher data resolution. The high number of presence points enabled us to study the range of species distribution gathering them according to their ecological characteristics. The generated reforestation suitability maps and the likelihood of occurrence of each species were achieved to define priority species for conservation and forest management. This information could be useful for decision-makers and foresters.
Keywords
Ecological Niche Modelling, Suitability Maps, Cloud Coverage, Range of Distribution, MaxEnt
Introduction
Forests have been traditionally managed and exploited for a variety of purposes in the Mediterranean region. These ecosystems face chronic problems such as forest fires, fragmentation, overexploitation, pest outbreaks and climate change ([30], [8]). Ecosystem restoration through reforestation is currently a major tool to address these challenges. Several countries around the world are currently recurring to such activities to deal with environmental, economic and social concerns. Aversely, to achieve it a substantial funding from governments is required.
The importance of forest ecosystems to increase the resilience of human populations is incontestable. The efficiency and effectiveness of reforestation activities depend on the available resources, funds and how much research is already available to guide stakeholders. Optimizing ecosystem restoration is possible through the understanding of the bioclimatic niche of the target species.
Species Distribution Models (SDMs) or, in other words, Ecological Niche Modelling (ENM) depicts the relationship between the current distribution of a given species (dependent variable) and the environmental factors/predictors (explanatory variables) that induced its distribution ([27], [14], [6], [26]). A wide array of software and statistical techniques coupled with geographic information systems (GIS) and remote sensing has been used in ENM ([13]). Systematics and correlative approaches are the base for developing ENM, where field surveying and environmental predictors are used to produce statistically derived response surfaces. An evaluation of the correlation between the environment and the species is usually derived out of the predicting models. In addition, the ranking of the input factors and the rating of their impact on the species distribution are concluded from the ENM ([15], [3]). The main drivers or predictors affecting the habitat distribution of a given group of taxa like oaks enabled understanding their phylogeny and discriminating the different species ([11], [34]).
ENM allows forecasting the future suitability area of species under different climate scenarios. Outputs are illustrated by generated maps or models that estimate the species’ distribution and (or) their ecological niche in case of a forecasting approximation ([28], [33], [32]).
In terms of ENM, it is crucial to understand the environmental factors that are affecting the distribution of species. Regardless of the flaws of the different models, the overall patterns of predicted species range shifts, often match observed biological tendencies ([40], [3]). In addition to geographical coordinates (altitude, latitude, and longitude), most investigations consider classical climatic parameters, such as annual precipitation, precipitation in the different quartiles of the year (seasonal precipitation), evapotranspiration, average temperature, the minimum temperature of the coldest month, the maximum temperature of the hottest month, continentality index, Emberger quotient, length of the dry season, etc. ([5], [41]). Topography or soil parameters and other microscale predictors are generally omitted due to the large spatial scale of the models.
Populations in edge conditions are sure to exhibit the highest variability according to environmental factors changes, being of major concern when they are considered for studying the effects of climate change ([4]). The Near East region is a crossroad for several biomes including the Mediterranean, the Euro-Siberian and the Irano-Turianian, where several tree species populations find their limit of distribution ([8], [36]). ENM investigations were tested on a large scale reaching the total extent of significant species in the region such as Quercus calliprinos (included as infraspecific taxon within Q. coccifera by some authors), Quercus brantii, and Juniperus drupacea ([5], [3], [41]). However, these models are not transferable for forest management and ecosystem restoration at the local scale.
Lebanon has a characteristic geographical situation to study populations in edge conditions for an array of native tree species, on a much smaller scale. The country lies to the eastern shores of the Mediterranean Sea and constitutes the boundary between the Mediterranean and the Irano-Turanian biomes. The presence of two parallel mountain ranges with substantial elevation (3088 m in Mount Lebanon range, and 2814 m in Anti-Lebanon and Mount Hermon) allows also relic species from the temperate zones to thrive ([2], [8]). Hence, the country is the southern or eastern limit of many species such as Abies cilicica, Acer tauricolum, Acer monspessulanum microphyllum, Arbutus andrachne, Cedrus libani, Cupressus sempervirens, Fraxinus ornus, Juniperus drupacea, Juniperus excelsa, Quercus cerris and Sorbus torminalis. Moreover, forests are highly fragmented and species like Amygdalus orientalis, Malus trilobata, Quercus ithaburensis, Quercus cedrorum, Quercus look and Quercus kotschyana are rare or endemic ([31], [38], [35]).
The national reforestation programs face challenges related to land availability, conflicts with different types of land use, the economic value of the planted species, the high cost of plantation and management to secure acceptable seedling survival rates. It is crucial to address such challenges using an ENM that would integrate common and novelty predictors affecting the distribution of the listed species. Thus, it would be capable to downsize the outputs to make them useful for foresters at the local scale, improving the effectiveness and efficiency of afforestation and reforestation programs through higher survival rates.
This study aims at (i) assessing the environmental factors, which affects the distribution of 27 tree species native from Lebanon, and (ii) obtaining their respective suitability maps for afforestation and reforestation programs.
Material and methods
Tab. 1 - The factors (predictors) used in the first and second fits of the model, with their respective codes and sources. Obtained from: (a) the Lebanese Army Geographic Department; (b) National Scientific Research Center; (c) WorldClim (1970-2000); (d) National Scientific Research Center (2002-2013).
Code | Factors | Source | Remarks |
---|---|---|---|
DEM | Digital Elevation Model (m) | 10m Contour Line a | Kept in final fit |
IMI | Integrated Moisture index | Hill shade | Omitted in final fit |
Curvature | Omitted in final fit | ||
Flow Accumulation | Omitted in final fit | ||
Available water holding capacity (AWC) (estimated in mm from soil depth and texture as extracted from CNRS soil maps) b | Kept in final fit of the model as independent factor | ||
PDIR | Potential Direct Incident Radiation (MJ/cm2 year-1) | Slope (extracted from DEM) in percentage | Kept in final fit as independent factor |
Folded Aspect (extracted from DEM) in degree | Kept in final fit as independent factor | ||
Latitude (GIS coordinates) | Omitted in final fit | ||
EQ | Emberger Quotient | Cumulative Annual Precipitation (mm) | Kept in final fit |
Mean of Minimum Temperature of the Coldest Month (°C) c | Kept in final fit | ||
Mean of Maximum Temperature of the Hottest Month (°C) c | Added in final fit also as an independent factor (Tmax) | ||
NDVI | Normalized Difference Vegetation Index | Mosaic of Landsat images | Omitted in final fit |
CC | Mean Cloud Coverage (May through July, %) | Cloud rasters d | Kept in final fit |
DFS | Distance from the Sea (m) | DEM | Kept in final fit |
Tmin | Mean of Minimum Temperature of the Coldest Month (°C) | Mean of Minimum Temperature of the Coldest Month c | Kept in final fit |
P | Cumulative Annual Precipitation (mm) | Precipitation Isohyets b | Kept in final fit |
Data stocktaking relied on two main sources: previously collected points by the authors and a more recent field survey in 2018. Field cruising or traveling across the field with a vehicle allowed better to consider the abundance of a species in order to cover the maximum range of variability and representation. Such an approach combining different tree species, different data sources and abundance counts are key measures required to secure the transferability of the model ([42]). Field cruising was conducted using itineraries covering all the biogeographic regions from the coast, to Mount Lebanon western and eastern slopes, the Beqaa and the eastern slopes of Anti Lebanon. The previously collected points were from random or systematic field plots in protected areas. Out of the classical climatic parameters commonly used in SDM, we selected only the cumulative annual rainfall (P), the mean minimum temperature of the coldest month (Tmin), and Emberger quotient (EQ). The mean maximum temperature of the hottest month (Tmax) was added in the final fit, while the Normalized Difference Vegetation Index (NDVI) was tested only in the first variables fit, as shown in Tab. 1. In addition, we tested the mean cloud coverage (CC) of the months of May through July calculated for a 12-year period (2002 to 2013). Two biogeographical predictors were used: distance from the sea (DFS) and digital elevation model (DEM). The Integrated Moisture Index (IMI), which is a composite predictor combining geographic, topographic and soil indices were adapted from Iverson et al. ([20]) with different combinations of weights for the components of the predictor (eqn. 1):
where HS is the hillshade, C is the curvature, FA is the flow accumulation and AWC is the available water holding capacity. ArcGIS® (ESRI, Redlands, CA, USA) tools allowed calculating the three factors, using the DEM and soil maps as input data.
Potential Direct Incident Radiation (PDIR) as per McCune & Keon ([25]) was also tested, using the following equation (eqn. 2):
where L is the latitude, S is the slope and A is the folded aspect.
The sources of these respective parameters are presented in Tab. 1, and include the National Centre for Remote Sensing at the National Scientific Research Council, which provided the cloud coverage data in addition to topographic, rainfall and soil maps to generate IMI and PDIR. Climatic parameters were retrieved from Worldclim database over a time series spanning from 1970 to 2000. A first fit of the model was initially run with all the above-mentioned predictors, and another fit omitting PDIR and IMI due to their limited effect on the results, and NDVI because it limits the presence points of the model. Simple topographic factors that are components of IMI and PDIR were added to the second fit: aspect, slope and available water holding capacity (AWC). Correlation between different predictors was calculated. Despite the high correlation between Tmax and Tmin, Tmin and DEM, or between EQ and P, these were retained since their contribution is highly variable from one species to another. Another reason to keep them was that DEM, EQ and Tmin are simultaneously used in the definition of vegetation levels and bioclimatic zones in the Mediterranean region ([9]).
From the collected presence points, crossed with the different layers of the selected predictors, the range of distribution of the species was calculated according to those predictors or environmental factors. MaxEnt software v. 3.4.1, a discriminative model that assigns a class to an observation by computing a probability was used ([29]). Even in the case of presence-only data, it can compare favourably to presence/pseudo-absence models when tested against real presence/absence data ([12]). Every suitability map shows the likelihood of the occurrence of a species, which corresponds to the average predicted logistic suitability for a species in a certain location.
The extent of the background cells for all the species is set to the boundaries of Lebanon. We used auto features and a regularization parameter of 1 for feature selection for all species. The weight of background cells is assumed equal to the extent of the study area. The size of a background cell is taken as 30m, which is similar to the resolution of the predictor variables and reflective of the conditions in the field.
In general, we used MaxEnt default parameters to produce the suitability maps for the 27 species since we assumed minimal knowledge of species distribution ranges and abundancy. Another reason for our choice of the pre-mentioned model’s settings is the significant number of species to model, which made the adoption of default settings that are generally tested and best fit for general conditions a reasonable choice for our study.
The Area under the Curve (AUC) generated by the model allowed for the evaluation of the robustness of the multiple MaxEnt models that we tested ([37], [29]). Jack-knife test of variable importance displayed the training gain of each factor if the model was run using that factor in isolation. It also allowed identifying which variables mostly contributed to the models for the respective species.
Results
Fig. 1 shows the total points used for modelling by species. Quercus infectoria and Q. calliprinos were the most recorded species with 2092 and 1914 points respectively. By contrast, Malus trilobata and Amygdalus orientalis were modelled with 55 and 92 points respectively. In total, 13.837 points were recorded for modelling 27 species.
Tab. 2 displays the factors used in the second fit model and their relative predictive contribution in comparison to each other. The higher the contribution, the more impact the variable will have on the occurrence of the target species. Tmax and P were the most significant explanatory variables for 7 and 6 of the studied species respectively. Tmin was also of great interest, being the second or third most important contributor in 16 out of 27 species. On the other hand, aspect played an opposite role by not being among the three first most significant contributors for any of the species. The remaining variables (AWC, DEM, DFS, EQ, CC and slope) had an intermediate position with disparate results depending on the studied species. DFS found the highest contribution reaching 60.5% in Ceratonia siliqua, although in most of the cases, the first contributor did not reach 50%.
Tab. 2 - Factors predictive contribution (in %) to the model, by species for the second model (superscript values indicate the first and the second contributors).
ID | Species | Aspect | AWC | DEM | DFS | EQ | Tmax | CC | Tmin | P | Slope |
---|---|---|---|---|---|---|---|---|---|---|---|
1 | Abies cilicica | 0.1 | 0.3 | 0.5 | 0.6 | 3.2 | 45.21 | 39.92 | 6.1 | 3.8 | 0.4 |
2 | Acer monspessulanum microphyllum | 3.6 | 1.5 | 55.31 | 5.7 | 0.7 | 15.72 | 2.1 | 8.9 | 3.7 | 2.7 |
3 | Acer obtusifolium | 2.4 | 1.0 | 16.1 | 18.62 | 17.3 | 4.0 | 2.1 | 8.8 | 3.2 | 26.61 |
4 | Acer tauricolum | 2.1 | 14.12 | 1.0 | 9.8 | 12.5 | 47.21 | 4.2 | 6.0 | 2.9 | 0.2 |
5 | Amygdalus orientalis | 4.5 | 3.9 | 14.0 | 17.22 | 8.3 | 3.7 | 5.1 | 7.2 | 29.41 | 6.7 |
6 | Arbutus andrachne | 4.8 | 0.9 | 3.9 | 23.32 | 14.3 | 1.9 | 2.2 | 15.0 | 7.8 | 25.91 |
7 | Cedrus libani | 0.3 | 3.2 | 0.6 | 17.72 | 0.2 | 58.41 | 1.1 | 11.3 | 6.8 | 0.3 |
8 | Ceratonia siliqua | 0.1 | 0.3 | 7.9 | 60.51 | 8.4 | 4.2 | 0.5 | 10.92 | 1.1 | 6.1 |
9 | Cercis siliquastrum | 6.7 | 1.1 | 3.2 | 4.7 | 35.91 | 1.9 | 4.7 | 13.0 | 6.8 | 22.02 |
10 | Crataegus azarolus | 1.8 | 8.6 | 27.51 | 9.5 | 1.0 | 12.5 | 11.3 | 2.4 | 19.32 | 6.1 |
11 | Cupressus sempervirens | 1.5 | 2.2 | 2.4 | 12.1 | 35.01 | 2.2 | 1.5 | 4.7 | 15.0 | 23.32 |
12 | Fraxinus ornus | 6.5 | 6.7 | 2.4 | 1.9 | 32.81 | 6.9 | 8.5 | 17.1 | 4.5 | 12.82 |
13 | Juniperus drupacea | 1.9 | 3.2 | 4.4 | 6.8 | 4.7 | 26.72 | 4.8 | 13.9 | 32.71 | 0.8 |
14 | Juniperus excelsa | 0.6 | 0.8 | 36.81 | 10.1 | 2.4 | 28.22 | 4.3 | 12.4 | 4.2 | 0.3 |
15 | Laurus nobilis | 4.5 | 4.1 | 9.3 | 26.81 | 7.2 | 3.3 | 6.0 | 23.72 | 3.7 | 11.4 |
16 | Malus trilobata | 1.6 | 1.3 | 10.2 | 6.6 | 1.0 | 20.92 | 32.81 | 16.6 | 1.4 | 7.5 |
17 | Pyrus syriaca | 4.7 | 3.1 | 24.02 | 12.3 | 3.1 | 11.8 | 0.9 | 3.3 | 26.11 | 10.7 |
18 | Pinus pinea | 0.9 | 19.42 | 0.8 | 3.8 | 0.8 | 1.6 | 1.7 | 18.6 | 48.01 | 4.5 |
19 | Quercus coccifera calliprinos | 1.0 | 2.3 | 2.9 | 5.1 | 12.2 | 5.2 | 4.1 | 25.62 | 13.2 | 28.41 |
20 | Quercus cedrorum | 5.5 | 16.0 | 0.3 | 0.1 | 16.82 | 43.11 | 1.8 | 11.7 | 4.3 | 0.5 |
21 | Quercus cerris | 1.5 | 1.2 | 0.7 | 2.5 | 17.2 | 32.81 | 22.62 | 17.0 | 4.1 | 0.4 |
22 | Quercus infectoria | 1.1 | 1.1 | 17.4 | 1.2 | 4.2 | 2.9 | 1.9 | 19.42 | 48.41 | 2.4 |
23 | Quercus ithaburensis | 0.4 | 0.2 | 15.22 | 14.6 | 0.0 | 3.2 | 60.61 | 0.1 | 5.7 | 0.1 |
24 | Quercus kotschyana | 0.4 | 0.4 | 0.3 | 0.4 | 11.7 | 46.61 | 2.3 | 11.2 | 25.62 | 1.1 |
25 | Quercus look | 1.8 | 23.62 | 36.71 | 4.1 | 2.2 | 4.9 | 1.2 | 11.9 | 12.6 | 1.0 |
26 | Sorbus torminalis | 1.7 | 8.2 | 1.8 | 6.2 | 7.6 | 48.91 | 6.1 | 12.72 | 5.4 | 1.5 |
27 | Styrax officinalis | 4.3 | 1.7 | 2.5 | 2.4 | 1.3 | 4.7 | 10.3 | 15.7 | 35.61 | 21.72 |
- | Average contribution | 2.4 | 4.8 | 11.0 | 10.5 | 9.7 | 18.11 | 9.1 | 12.0 | 13.92 | 8.3 |
The performance of the model was assessed by the AUC. All the obtained results were higher than the random value (0.5), even most of them higher than 0.9 proving that MaxEnt was an efficient predictive software (see Tab. S1 in Supplementary material). Quercus cedrorum reached the highest AUC (0.994) whilst Quercus calliprinos reached the lowest (0.788). Tab. 3 shows the highest values of the Likelihood of occurrence (LOC) for the target species and the respective area of the likelihood of occurrence exceeding a ratio of 0.5 (LOC50).
Tab. 3 - Likelihood of occurrence (LOC) values and area where values are > 0.5 (LOC50) for the respective taxa.
ID | Taxon | Highest LOC value |
Area with LOC50 (ha) |
---|---|---|---|
1 | Abies cilicica | 0.71 | 5,167 |
2 | Acer monspessulanum microphyllum | 0.94 | 55,008 |
3 | Acer obtusifolium | 0.97 | 40,706 |
4 | Acer tauricolum | 0.87 | 5,524 |
5 | Amygdalus orientalis | 0.97 | 10,318 |
6 | Arbutus andrachne | 0.97 | 54,153 |
7 | Cedrus libani | 0.78 | 8,022 |
8 | Ceratonia siliqua | 0.82 | 61,248 |
9 | Cercis siliquastrum | 0.98 | 50,352 |
10 | Crataegus azarolus | 0.97 | 110,067 |
11 | Cupressus sempervirens | 0.96 | 54,193 |
12 | Fraxinus ornus | 0.98 | 16,065 |
13 | Juniperus drupacea | 0.86 | 5,167 |
14 | Juniperus excelsa | 0.83 | 64,054 |
15 | Laurus nobilis | 0.99 | 43,119 |
16 | Malus trilobata | 0.96 | 30,399 |
17 | Pyrus syriaca | 0.99 | 152,112 |
18 | Pinus pinea | 0.90 | 74,259 |
19 | Quercus calliprinos | 0.83 | 174,139 |
20 | Quercus cedrorum | 0.78 | 655 |
21 | Quercus cerris | 0.94 | 13,923 |
22 | Quercus infectoria | 0.80 | 151,052 |
23 | Quercus ithaburensis | 0.67 | 3,543 |
24 | Quercus kotschyana | 0.90 | 8,189 |
25 | Quercus look | 0.91 | 6,147 |
26 | Sorbus torminalis | 0.92 | 7,728 |
27 | Styrax officinalis | 0.97 | 82,406 |
A second run exhibited higher AUC values for some of the species; therefore, it was adopted for further analysis. Tab. S1 and Tab. S2 (Supplementary material) shows the values used in Jack-knives to express training gain if a certain factor is omitted and training gain if a certain factor is used in isolation respectively. The range of distribution of the selected species according to the investigated environmental factors is illustrated in boxplots aiming to visualize the distribution of 50% of individuals, along with average maximal and minimal values (Fig. S1 to Fig. S7 - Supplementary material). Due to the large number of studied species in this work, we illustrate in Fig. 2 the suitability map and presence points of Laurus nobilis, while those of the remaining taxa are shown in Fig. S8 (Supplementary material).
Fig. 2 - Observed (a) and potential (b) distribution of Laurus nobilis in Lebanon. LOC value classes determine the color gradient in the potential distribution map (b).
Discussion
The present work investigated the potential distribution of 27 species by ENM in Lebanon. The study area is of great interest because extreme environmental and topographical ranges are found from the Mediterranean Sea to the highest mountains. Qurnat as Sawda reaches the highest point with 3088 m a.s.l., only 30 km far away from the sea. These traits are also found in other circum-Mediterranean areas like North Africa or Southern Spain. In the latter, recent ENM studies have been achieved with successful results ([21], [22], [23], [24]). Thus, these areas are pretty suitable in the context of global change where temperatures are supposed to raise as well as a changing pattern in rainfall is expected ([19]). It is the first tentative among ENM surveys in which 27 native species are modelled simultaneously, most of them at the edge of the area of distribution with high resolution. The high AUC values for most of the species reflect a very good predictability power since models with AUC values above 0.75 are potentially useful ([37], [29]). It is evident that species with a high number of points (i.e., Quercus calliprinos or Q. infectoria), faced aggregation and resulted in lower AUC values, yet without compromising the model.
Cloud coverage (CC) during spring and summer is a novelty factor for species thriving in edge conditions, since this factor would compensate for the lack of precipitation in summer, and would help to explain the distribution of relic species such as Abies cilicica and Quercus cerris, which are found on their southernmost limits. Despite the possible correlation between other environmental factors related to temperature, elevation, precipitation or Emberger quotient, solid results were obtained converging with several similar studies ([39], [5], [1]). Composite factors such as PDIR or IMI need further refinement and higher resolution data if modelling for local use is desired ([20], [17]). Nevertheless, satisfactory results are possible if these composite factors are segregated into simple factors such as slope or AWC considered in the present work.
The contribution of each predictor in the model and the strength of the results highly depend on: (i) the resolution of the data from the source; (ii) the distribution of presence points across the studied area; and (iii) how spread they are to cover all possible ranges without being aggregated in one area where no changes in environmental variables can be depicted. Species showing wider distribution over different environmental gradients resulted in wider suitability areas, even if they are not abundant (i.e., Malus trilobata, Laurus nobilis, Crataegus azarolus). Species with presence points grouped into specific areas (Juniperus excelsa, Quercus cedrorum, Quercus ithaburensis) obtained a restricted potential area. The latter species witnessed aggregation and overfitting which affected the output map, LOC values and LOC50 predicted area. Distribution maps indicate ratios of the likelihood of occurrence (LOC), calculated by MaxEnt for each concerned species. The values of LOC and the area with LOC > 50% (LOC50) are shown in Tab. 3. The generated suitability maps are coherent with the respective presence points of each species, their environmental requirements and their realized niche ([10], [43], [2], [16], [7], [31], [5], [34], [41]).
Fig. 3, Fig. 4, Fig. 5 and Fig. S1 to Fig. S7 (Supplementary material) show the range of the selected environmental factors per target species. The potential niche matches with the range of the realized niche. Thus, the studied species can be categorized into four major ecologic groups, based on the biogeographic and climatic factors as shown in the above-mentioned figures:
Fig. 3 - Range of distribution of species according to elevation (m). Species of groups I, II, III and IV are respectively illustrated in red, blue, yellow and green.
Fig. 4 - Range of distribution of species according to the mean of minimum temperature of the coldest month (°C). Species of groups I, II, III and IV are respectively illustrated in red, blue, yellow and green.
Fig. 5 - Range of distribution of species according to the cumulative annual precipitation (mm). Species of groups I, II, III and IV are respectively illustrated in red, blue, yellow and green.
- Group I: Strictly Mediterranean species that prefer low altitudes (below 1000m a.s.l.), close distance to the sea (below 20km), that do not tolerate freezing minimal temperatures in winter, but can endure high temperatures in summer. The taxa belonging to this group are indifferent to cloud coverage, but require a certain minimum of annual precipitations (above 600mm). This group includes Acer obtusifolium, Arbutus andrachne, Ceratonia siliqua, Cercis siliquastrum, Cupressus sempervirens, Laurus nobilis, Pinus pinea, and Quercus ithaburensis.
- Group II: Mediterranean mountain and temperate relic species that are on middle to high altitudes, the average distance to the sea (15 to 25km), with cooler temperatures, ample rainfall (above 1000 mm) and substantial cloud coverage (more than 20%). These include Abies cilicica, Acer tauricolum, Cedrus libani, Fraxinus ornus, Juniperus drupacea, Malus trilobata, Quercus cerris, Quercus cedrorum, Quercus kotschyana, and Sorbus torminalis. The ecological niche of these species is highly variable in extent, depending on their respective requirements. They are tolerant to freezing temperatures, whilst P and CC are variable. Tmin seems to be the major factor defining the niche of these species, although the strict presence of Abies cilicica, Quercus cerris and Malus trilobata on the seaward western slopes of Mount Lebanon is mostly driven by CC.
- Group III: Irano-Turanian elements that are relatively distant from the sea (over 22km), on high altitudes (mostly above 1500m a.s.l.), enduring low CC in spring (below 18%) and withstanding both high and low temperature extremes as well. Their tolerance to precipitation ranges are variable, but showing higher tolerance to arid environment. In this group are included Acer monspessulanum microphyllum, Amygdalus orientalis, Crataegus azarolus, Quercus look and Juniperus excelsa. Elevation is the major factor affecting their distribution.
- Group IV: includes all species showing a wide range of distribution for several climatic factors, being found in the Mediterranean biome and transition areas toward the Irano-Turanian biome. These include Pyrus syriaca, Quercus calliprinos, Quercus infectoria and Styrax officinalis. The most determining factor in their distribution is precipitation followed by Tmin. Species of this group benefit from the widest LOC50 and highest LOC values because of their high plasticity.
This work showed a robust output inferred by the AUC values. Therefore, stakeholders aiming to face the expected upcoming changing conditions ([19]) can use 27 suitability maps for forest management and conservation programs. Several scenarios are available according to either harsher or smoother conditions. Different RCP (Representative Concentration Pathways) from WorldClim 1.4 project ([18]) are of great interest to understand how suitability areas of species could shift. It is encouraged to use at least two of these RCPs in the way to assess the likely uncertainty in their ranges. Recent investigations simulated changes in the ecological niche under future climate scenarios for few species (i.e., Quercus calliprinos, Juniperus drupacea). The results showed a reduction of the suitable area of these species at their global range, with limited changes in Lebanon which benefit from high mountains and the possibility for shifting towards higher altitudes ([5], [41]). Nonetheless, it would be particularly interesting to investigate the remaining species, namely those that are endemic or thriving in restricted environmental ranges or in edge conditions (i.e., species of Group II).
Conclusion
The contribution of each environmental factor to models varies with the studied species. Limiting the models by reducing the number of predictors did not affect the validity of models. Basic climatic and biogeographic factors such as minimal and maximal temperatures, annual precipitation, elevation and distance from the sea are pertinent on a small scale. Cloud cover during spring and summer is a key contributing factor for ecological niche modelling of species on the edge of their distribution range. Composite factors related to terrain and soil characteristics should be simplified and their contribution is conditional to higher data resolution. Limiting multiple models to local context for simultaneously studied species allowed grouping them according to their environmental preferences, producing suitability maps for potential reforestation plans, determining priority species for conservation actions, and contributing to the IUCN red list for the concerned species.
Acknowledgments
Jean Stephan designed and conceived this research; Christel Bercachy conducted field data collection, model running and mapping; Joseph Bechara provided assistance in model development and data stocktaking; Eliane Charbel and Javier Lopez-Tirado reviewed the paper, namely the material and method and discussion. The Lebanese Reforestation Initiative provided the technical assistance and the financial support of this work. We are thankful for the National Center for Remote Sensing of Lebanon for providing precipitation and cloud cover data and for Maya Nehme and Samar Haddad for editing the manuscript.
References
Gscholar
Gscholar
Gscholar
CrossRef | Gscholar
Gscholar
Online | Gscholar
CrossRef | Gscholar
Gscholar
Gscholar
Gscholar
CrossRef | Gscholar
CrossRef | Gscholar
Gscholar
Authors’ Info
Authors’ Affiliation
Christelle Bercachy
L2GE, Department of Life and Earth Sciences, Faculty of Science, Lebanese University, Fanar (Lebanon)
Integrative Sciences Department, Faculty of Experimental Sciences, University of Huelva, Avda. Tres de Marzo s/n, 21071 Huelva (Spain)
Corresponding author
Paper Info
Citation
Stephan J, Bercachy C, Bechara J, Charbel E, López-Tirado J (2020). Local ecological niche modelling to provide suitability maps for 27 forest tree species in edge conditions. iForest 13: 230-237. - doi: 10.3832/ifor3331-013
Academic Editor
Maurizio Marchi
Paper history
Received: Dec 30, 2019
Accepted: Apr 27, 2020
First online: Jun 19, 2020
Publication Date: Jun 30, 2020
Publication Time: 1.77 months
Copyright Information
© SISEF - The Italian Society of Silviculture and Forest Ecology 2020
Open Access
This article is distributed under the terms of the Creative Commons Attribution-Non Commercial 4.0 International (https://creativecommons.org/licenses/by-nc/4.0/), which permits unrestricted use, distribution, and reproduction in any medium, provided you give appropriate credit to the original author(s) and the source, provide a link to the Creative Commons license, and indicate if changes were made.
Web Metrics
Breakdown by View Type
Article Usage
Total Article Views: 9893
(from publication date up to now)
Breakdown by View Type
HTML Page Views: 7248
Abstract Page Views: 701
PDF Downloads: 1407
Citation/Reference Downloads: 2
XML Downloads: 535
Web Metrics
Days since publication: 1499
Overall contacts: 9893
Avg. contacts per week: 46.20
Article Citations
Article citations are based on data periodically collected from the Clarivate Web of Science web site
(last update: Nov 2020)
(No citations were found up to date. Please come back later)
Publication Metrics
by Dimensions ©
Articles citing this article
List of the papers citing this article based on CrossRef Cited-by.
Related Contents
iForest Similar Articles
Research Articles
Hemlock woolly adelgid niche models from the invasive eastern North American range with projections to native ranges and future climates
vol. 12, pp. 149-159 (online: 04 March 2019)
Research Articles
Spatial modeling of the ecological niche of Pinus greggii Engelm. (Pinaceae): a species conservation proposal in Mexico under climatic change scenarios
vol. 13, pp. 426-434 (online: 16 September 2020)
Research Articles
Predicting the impacts of climate change on the distribution of Juniperus excelsa M. Bieb. in the central and eastern Alborz Mountains, Iran
vol. 11, pp. 643-650 (online: 04 October 2018)
Research Articles
Predictive capacity of nine algorithms and an ensemble model to determine the geographic distribution of tree species
vol. 15, pp. 363-371 (online: 20 September 2022)
Research Articles
Spatio-temporal modelling of forest monitoring data: modelling German tree defoliation data collected between 1989 and 2015 for trend estimation and survey grid examination using GAMMs
vol. 12, pp. 338-348 (online: 05 July 2019)
Research Articles
Some refinements on species distribution models using tree-level National Forest Inventories for supporting forest management and marginal forest population detection
vol. 11, pp. 291-299 (online: 13 April 2018)
Research Articles
Suitability of Fagus orientalis Lipsky at marginal Fagus sylvatica L. forest sites in Southern Germany
vol. 15, pp. 417-423 (online: 19 October 2022)
Research Articles
Climate change may threaten the southernmost Pinus nigra subsp. salzmannii (Dunal) Franco populations: an ensemble niche-based approach
vol. 11, pp. 396-405 (online: 15 May 2018)
Research Articles
Environmental niche and distribution of six deciduous tree species in the Spanish Atlantic region
vol. 8, pp. 214-221 (online: 28 August 2014)
Short Communications
Dynamic modelling of target loads of acidifying deposition for forest ecosystems in Flanders (Belgium)
vol. 2, pp. 30-33 (online: 21 January 2009)
iForest Database Search
Search By Author
Search By Keyword
Google Scholar Search
Citing Articles
Search By Author
Search By Keywords
PubMed Search
Search By Author
Search By Keyword