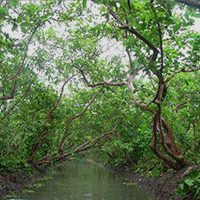
Monitoring of changes in woodlots outside forests by multi-temporal Landsat imagery
iForest - Biogeosciences and Forestry, Volume 11, Issue 1, Pages 162-170 (2018)
doi: https://doi.org/10.3832/ifor2021-010
Published: Feb 19, 2018 - Copyright © 2018 SISEF
Research Articles
Abstract
Woodlots outside forests play a significant role in diversifying household income, reducing poverty, supplying timber and fuel-wood, and regulating the local environment in many countries with low forest cover. This study aimed to develop a method for delineating the spatial footprint of woodlots outside forests and assessing their changes over time. The test site was located in the Jhalokati District of south-western Bangladesh, one of the world’s most densely populated regions. Landsat images from 2010 were classified using a supervised method. Woodlots were extracted, converted to vector layers, and manually edited. The overall accuracy of the 2010 land cover map was 87%-89%. A change vector layer was generated by further updating of the vector layer by overlaying a 1989 Landsat image. The total coverage of woodlots in the district increased between 1989 and 2010, from 19.638 ha (27%) to 27.836 ha (39%). The study identified two primary reasons for changes in woodlot coverage: (i) woodlot expansion associated with the population growth and establishment of new households; and (ii) conversion of cropland to orchards because of economic reasons. The results will improve understanding of the spatial distribution of woodlot coverage in the study area and their dynamics over time.
Keywords
Woodlot, Trees Outside Forests (TOF), Landsat, Change, Mapping
Background
Forests supply and sustain various forest products for household uses, support habitats for wildlife, conserve biological diversity, and provide a range of ecosystem services to local communities. Forests are dynamic and change over time with the influence of human and natural drivers. Earlier efforts at assessing land cover changes have estimated the extent of forest cover change in different regions ([17], [7], [30], [36]). Compared to studies of forest cover change, there have been a limited number of efforts to map and quantify changes in Trees Outside Forests (TOF - [24]), though it is important to assess the spatial footprints of changes in these trees over time ([8]).
TOF usually represents the aggregation of scattered trees grown on cropland, grazing land, marginal land, canal banks, roadsides, along railways, and in human settlements. These trees cannot be included in the category “forests” or “other wooded land” in forest mapping ([4], [28]). Woodlots are typically clusters of trees usually growing outside of forests on cropland and in human settlements; it does not include trees growing in isolation or trees planted in lines or in narrow strips. In Bangladesh, the definition of TOF includes all trees growing outside of forests, usually clusters of trees distributed on the rural landscape. The extent of woodlots is not limited to a specific threshold, for example 0.5 ha, which is the definition the FAO often uses for defining forest and assessing forest resources.
The extent of woodlots under individual ownership is often variable and sometimes smaller than the threshold can be suitably monitored using Landsat data; it becomes larger when the woodlots from many owners are located one after another and form a continuous pattern, which is a common pattern of rural landscape found in the study area. In Bangladesh, the definition of TOF is usually synonymous to village forests which belong to the private ownership and are managed by the respective owners; in contrast, forests generally belong to the Government, and are managed by the state-owned Forest Department.
TOF, in woodlots, in lines or narrow strips or in isolation, can be a major source of timber and non-timber forest products in countries where forest coverage is low ([8]). Woodlots produce forest products (fuel wood and timber), mitigating the demand of local and regional markets and playing a role in diversifying household economies (cash income from selling timber, fruits etc.). Woodlots also contribute to biodiversity conservation and provide habitat for wildlife. Trees help to maintain water quality and quantity (slowing rainfall runoff, reducing sediment and nutrient loading to waterways, and protecting stream banks from erosion), soils (minimizing wind and water erosion), and air quality (filtering air pollutants, creating shade, and cooling - [20]). Woody plants in TOF will play a role in climate change adaptation, mitigation (reducing the impact of floods, storm-surges, droughts etc.), and carbon sequestration ([12], [20]).
The structure and composition of woodlots and other TOF might be more heterogeneous and inconsistent than regular forests; their extent is generally smaller and distribution is sparse. Land tenure is often different, and consequently their assessment might require a different approach than estimation of forest cover ([6]). The economic contribution of these trees to rural livelihoods and their ecological roles in rural landscapes are usually not well-known beyond the local level ([6]). Therefore, local level studies are important to assess and map the spatial footprint of woodlot resources to analyse their changes and to understand their spatial extent, their dynamics, and their role in the local economy and ecology.
Local-level studies have been conducted in different regions to assess and map woodlots and other tree resources growing outside of forests. Liknes et al. ([18]) estimated tree coverage located outside of forests in North and South Dakota, USA using various layers of data-sets. Lister et al. ([19]) conducted an inventory of trees located outside forest areas in the Great Plains states, USA. Perry et al. ([23]) used Forest Inventory and Analysis (FIA) data of the USDA (United States Department of Agriculture) Forest Service to estimate the extent of tree resources on agricultural lands as well as other landscapes in the Midwest region of the United States and compared the estimates to those derived from Moderate Resolution Imaging Spectroradiometer (MODIS) Vegetation Continuous Fields (VCF).
Wiseman et al. ([38]) examined the reflectance of multi-spectral bands of high-resolution aerial imagery and other contextual information (shape, texture) to identify the species composition of shelterbelt trees grown under a prairie shelterbelt program in Manitoba, Canada. Welsch et al. ([37]) investigated the influence of landowner- and property-related characteristics and also landowner perceptions and attitudes on the potential change of woody vegetation across an intensively farmed agricultural landscape in Canterbury, New Zealand. Plieninger ([24]) estimated local level changes of TOF in two test sites of southern Germany between 1901/1905 and 2009 using analysis of multi-temporal maps.
The different image processing techniques used in woodlot mapping include pixel-based methods ([29]), object-based image analysis ([1], [26]), or a hybrid approach that combines both pixel- and object-based classification techniques ([34]). The remotely sensed imagery used in TOF mapping are aerial photographs ([18], [21]), high- to medium-resolution satellite images, i.e., QuickBird satellite imagery ([1]), IRS Cartosat-1 ([26]), IRS LISS-IV ([26]), IRS LISS-III ([29]), and moderate resolution Landsat Enhanced Thematic Mapper Plus (ETM+ - [25]).
The earlier studies mostly generated woodlot and tree cover maps of rural landscapes for a single time frame ([18], [26]); only a few investigations have assessed the changes in tree coverage in multi-temporal domains. Plieninger ([24]) considered published maps as historical information and satellite images as recent information in the change analysis. The objectives of this study were to: (i) develop a method for mapping and analysing change of woodlots (including orchards) located outside the forest from multi-temporal satellite (Landsat) data; (ii) apply the method to mapping the spatial footprint of changes in woodlot coverage on a test site over time; and (iii) investigate the major patterns of changes in woodlot coverage based on high-resolution remote sensing image (i.e., RapidEye) analysis and field verification. The study area is located in Jhalokathi District of south-western Bangladesh, where quantitative information on changes in woodlot coverage is not available.
The Bangladesh Village Forest Inventory ([13]) was the first nationwide TOF assessment and the assessment primarily used a ground-based sampling technique. In the National Forest Assessment conducted during 2005-2007, TOF were considered in the class “Villages”, which was included in other land category. The class consists of small rural settlements without trees, rural settlements with trees (size between 0.1 and 0.5 ha), and rural settlements with trees (size more than 0.5 ha). The data was obtained from National Forest Inventory and calibrated by remote sensing ([3]). Earlier studies conducted in Bangladesh did not aim to generate full coverage maps, statistics and change assessments in trees or woodlots outside forests. The contribution of this study was to present a technique for assessing changes in woodlot coverage over time using multi-temporal Landsat data.
The current study focused on the mapping of woodlots (cluster of trees in regular or irregular shape), including orchards with fruit producing trees located outside forests, using Landsat Thematic Mapper (TM) data. We also assessed their changes over time. Mapping of individual scattered trees or trees planted in lines or narrow strips (e.g., roadside plantation) was beyond the scope of current study. The extent of individual scattered trees or trees planted in linear shapes as tree coverage is much lower in the study areas compared to the woodlots.
Materials and methods
Study areas and datasets
The study area was located in the Jhalokati District of south-western Bangladesh (Fig. 1) and lies between 22° 20′ and 22° 47′ north latitude and between 90° 01′ and 90° 23′ east longitude. The total area of the district is 707 km2. The topography of the study area is flat and the mean elevation is 6.5 m (source: Digital Elevation Model of Shuttle Radar Topography Mission). Annual average temperature ranges from a maximum of 33 °C to a minimum of 12 °C and average annual rainfall is 2506 mm ([2]). The Jhalokati subdivision was established in 1972 and became a District in 1984. The District consists of four Upazilas named Jhalokati Sadar, Kanthalia, Nalchity and Rajapur.
Landsat TM images of 1989 and 2010, and RapidEye image of 2011 were used in preparing and validating the woodlot map (see Tab. S1 in Supplementary Material for details). The nominal spatial resolution of RapidEye imagery is 6.5 m and the image has five spectral bands: Blue (440-510 nm), Green (520-590 nm), Red (630-685 nm), Red Edge (690-730 nm) and NIR (760-850 nm).
Satellite image acquisition and pre-processing
Landsat images were downloaded from the United States Geological Survey (USGS); downloaded scenes were already processed to Standard Terrain Correction (Level 1T precision and terrain correction). RapidEye imagery was procured from the designated vendor and geo-corrected with the Landsat scenes using image-to-image geometric correction. The spatial accuracy of the satellite images was checked and the geometric distortion between Landsat and RapidEye imagery was found to be from 10 to 30 m in different places of the study area, which is lower than the dimension of a Landsat image pixel (30 m). This accuracy was considered appropriate in most of the study area for further analysis.
Interpretation and classification of Landsat image of 2010
Landsat satellite image of 2010 was displayed on computer screen in different band combinations to interpret different land covers. Descriptions of common land cover categories found in the study area are presented in Tab. 1. Various elements of interpretation such as colour, texture, patterns, and association were helpful in image interpretation.
Tab. 1 - Major land cover categories and their characteristics.
Category | Sub-category | Characteristics |
---|---|---|
Bare land /agriculture | Bare land | This is a contemporary class. Often this land belongs to seasonal agricultural crops. However, during the time of image acquisition this land was barren, not covered by crops. |
Agriculture | This land cover type belongs to paddies, seasonal vegetables, pulses etc. | |
Water-body | River | The study is located close to the sea and crossed by many rivers. |
Pond | Ponds are scattered in close vicinity to homesteads. Water from the ponds is used as part of the daily life of people living in the area. |
|
Ditches | Depressed land, seasonally used for agricultural production. | |
Woodlot | Village home gardens / Small woodlots in the vicinity of homestead | Multi-storied home gardens composed of a mixture of perennial crops. Most common tree species are mahogani (Swietenia macrophylla), koroi (Albizia spp.), raintree (Samanea samon), and akashmoni (Acacia auriculiformis). Trees provide fruits, fodder, fuel wood, timber etc. and cash income for the rural livelihood. |
Small woodlots on agricultural landscape |
Farmers raise plantations on small pieces of agricultu-ral land for timber production. Most common tree species include mahogani (Swietenia macrophylla, S. mahagony) and sisso (Dalbergia sisso) In some areas, fruit trees, i.e., guava (Psidium guajava) and amra (Spondias mombin), are planted in block plantations. If guava is planted, small canals have been dug parallel to the line of fruit-trees in order to facilitate guava harvesting during the fruit production season. |
|
Roadside or canal-bank plantation |
Trees are planted along the roadside or on canal banks. These trees help to protect the bank from erosion and assist in slope stabilization. Using Landsat satellite data, acquiring these plantations is difficult but it is distinguishable on RapidEye images. |
Landsat data was classified using unsupervised classification, applying the maximum likelihood classification algorithm. Ten classes were generated in the first step based on the reflectance properties acquired by satellite sensor data. The classes were finally merged into two categories: with and without tree cover pixels based on Landsat image interpretation and expert judgment. The study area does not contain any natural or planted forests managed by Bangladesh Forest Department, so the generated vector layer only represents woodlots outside forests.
Generation and updating vector layer of 2010
Landsat image pixels of 2010 classified as tree cover were extracted and converted to vector layers. Woodlot class generated in this study was based on two assumptions. Firstly, the minimum mapping unit was assumed to be equal to 0.18 ha, i.e., equivalent to two Landsat pixels, as Landsat data has a pixel size of 30 m. Another assumption used in the interpretation of mixed pixels was that a tree cover threshold of 50% was applied to classify Landsat pixels as tree cover. Since accurate estimation of tree cover percentage within a Landsat pixel is not possible, it has been used as background information for the interpreter during the interpretation and mapping of mixed pixels.
The derived vector layer was overlaid on an unclassified Landsat scene and was manually edited by an interpreter. If any land parcel represented tree cover and extending over minimum mapping unit but was not extracted as a tree-pixel by automatic classification, the vector layer was drawn/extended by on-screen digitizing. Similarly, in vector layer, any pixel-group extracted as tree cover but actually not containing trees was deleted by editing. Simultaneously, if a single pixel was classified as tree cover it was deleted as well since its spatial extent was lower than the minimum mapping unit. The updated vector layer was crosschecked by an independent interpreter.
Preparation of change vector layer and change analysis
After completion of the woodlot vector layer for 2010, the layer was overlaid on a Landsat image of 1989 and visible changes were updated by adding a 1989 woodlot boundary, keeping the boundary of 2010 unchanged, as it was drawn earlier. The layer was saved as a change vector layer for 1989 to 2010. Three land cover classes were considered in the change vector layer: woodlot, other land, and water. All of the polygons were assigned with a unique identification number representing different land covers both for recent (2010) and the previous time period (1989).
Changes in woodlot coverage in the study area have been investigated using remote sensing image analysis, ground verification and other ancillary information. Analysis of historical (Landsat) and recent satellite images (Landsat and RapidEye) supported by ground verification identified the patterns of woodlot change in the study region. Analysis of population statistics derived from census report and interviews with local people was helpful for further investigation on the driving forces involved in the change process of woodlot coverage.
Accuracy assessment of 2010 woodlot map
Landsat derived 2010 woodlot map was verified and validated with the RapidEye imagery and ground verification. In order to assess the accuracy three hundred sample points were generated by simple random sampling (no rules were used) and stratified random sampling (the number of points were stratified based on all different thematic classes) with allocation criteria considering different land cover classes as strata. Three strata were considered for allocating samples, namely, Woodlot, Other Land and Water. The sampling intensity was approximately 0.038%, 0.035% and 0.028% in simple random sampling for the class Woodlot, Other Land and Water, respectively, while these values were approximately 0.036%-0.037% for these three classes in stratified random sampling. Actual land cover for every point was manually checked by overlaying the points on the RapidEye dataset. An independent interpreter completed the crosschecking.
Field visits were conducted several times during 2011-2012 and 2014 to verify the woodlot map and to collect other ancillary information related to tree resources. During field verification, changes in land cover on the ground were noticed since the satellite imagery was acquired in 2010/2011. However, large-scale changes were not visible.
Results
Woodlot mapping in the Jhalokati District
The maps representing the woodlots in Jhalokati District are presented in Fig. 2. The maps for 1989, 2010, and the change map for 1989-2010 are presented in Fig. 2a, Fig. 2b, and Fig. 2c, respectively. The change map shows a general trend of woodlot expansion particularly concentrated in the north-western part of the District and sporadically distributed in other locations. The total coverage of woodlot for Jhalokati District has increased from 19.638 ha (27%) to 27.836 ha (39%) during 1989-2010 (Tab. 2) and the expansion was estimated at 8.198 ha (approximately 11%).
Fig. 2 - Distribution and changes of woodlot coverage in Jhalokati District: (a) Woodlot of 1989, (b) Woodlot of 2010, and (c) Changes of woodlot in 1989-2010. Legend in top is applicable to (a) and (b); legend in bottom is applicable to (c).
Tab. 2 - Upazila-wide estimates of tree coverage and their changes over time (1989-2010). Area was computed from the classified vector layer and the digital map of Survey of Bangladesh (SOB). (‡): Area was computed from the digital map of Survey of Bangladesh (SOB).
Name of Upazila |
Total area (‡) (ha) |
Woodlot area (1989) |
Woodlot area (2010) |
Changes in woodlot coverage (1989-2010) |
|||
---|---|---|---|---|---|---|---|
(ha) | (%) | (ha) | (%) | (ha) | (%) | ||
Jhalokati Sadar | 18,765 | 6,278 | 33.46 | 8,599 | 45.83 | 2,321 | 12.37 |
Rajapur | 15,773 | 4,015 | 25.45 | 5,710 | 36.20 | 1,695 | 10.75 |
Nalchity | 22,067 | 5,751 | 26.06 | 7,815 | 35.42 | 2,064 | 9.35 |
Kanthalia | 15,049 | 3,594 | 23.88 | 5,712 | 37.96 | 2,118 | 14.07 |
Total | 71,654 | 19,638 | 27.41 | 27,836 | 38.85 | 8,198 | 11.44 |
Upazila-wide woodlot estimation revealed that Jhalokati Sadar has the highest percentage of tree coverage (46%) in 2010, which is followed by Kanthalia (38%), Rajapur (36%) and Nalchity (35%). This result is similar to the estimate of 1989. Jhalokati Sadar had the highest percentage of tree coverage (33%) and it was followed by Nalchity (26%), Rajapur (25%), and Kanthalia (24%). In terms of tree cover change, the largest expansion was noted for Jhalokati Sadar Upazila and it was 2.321 ha (12%) in 1989-2010. In terms of percentage, the highest expansion was noted for Kanthalia Upazila and it was approximately 14% (2.118 ha).
The study covered all types of trees though the trees grown in woodlots are primarily timber and fruit producing trees, but trees with low economic importance (neither produce high-quality timber nor provide edible fruits with economic value) are sometimes present in the woodlots. Major timber species identified during the ground visit in village home gardens are mahogani (Swietenia macrophylla), koroi (Albizia spp.), raintree (Samanea saman), and akashmoni (Acacia auriculiformis). Fruit trees like mango (Mangifera indica), jackfruit (Artocarpus heterophyllus), guava (Psidium guajava), amra (Bengali name, in English-speaking Caribbean islands it is known as yellow mombin or hog plum, Spondias mombin) etc., are also seen in the home gardens. Trees planted on agricultural landscapes include guava (P. guajava) and mahogani (S. macrophylla). Major tree species planted on roadsides or canal banks are mahogani (S. macrophylla) and koroi (Albizia species). The selected field photographs acquired during field visits and representing woodlot are presented in Fig. 3.
Fig. 3 - Photographs of woodlots in the study region: (a) guava orchard (woodlot planted with guava trees); (b) amra trees planted in an agricultural landscape (in rows); (c) multi-storied home-garden/woodlot in the neighbourhood of homesteads; (d) mahogani trees in a woodlot.
Change assessment in woodlot coverage
Remote sensing image analysis and field verification identified two major patterns of woodlot expansion in the study region: (i) expansion of households with tree coverage; and (ii) conversion of cropland to orchard.
Expansion of households with tree coverage
New households associated with woodlots have been expanded in the study area and these are sporadically distributed in different locations. Population growth is one of the main drivers of establishing new households and planting woodlots in the neighbouring areas. Human settlements are mostly expanded on the existing cropland; thus woodlot is expanding by replacing the agricultural areas (Fig. 4).
Fig. 4 - Woodlot expansion associated with the establishment of new household in Jhalokati district during 1989-2010. (a) Landsat TM image of 1989; (b) Landsat TM image of 2010; and (c) RapidEye image of 2011. The images are overlaid with (a) a classified vector map of 1989 and (b and c) a vector change map for 1989-2010. Landsat images are displayed in bands 4, 5, and 3. RapidEye imagery is visualized in 5, 4, and 3 band combinations.
Analysis of population statistics revealed a general trend of population expansion in three Upazilas of Jhalokati District, namely Jhalokati Sadar, Rajapur, and Kanthalia, and a declining trend in Nalchity Upazila during 1991-2011 (Tab. 3 - source: Bangladesh Bureau of Statistics, BBS). Migration to other parts of the country is the probable reason for the declining population in Nalcity Upazila. Satellite image analysis indicates that many parts of the Upazila belong to the lowlands and hence, economic activity in the area could be limited.
Tab. 3 - Upazila-wide estimate of the population in Jhalokati District (source: Bangladesh Bureau of Statistics).
Name of Upazila | Population statistics (in thousands) | Change (in thousands) |
Change (in %) |
|||
---|---|---|---|---|---|---|
1981 | 1991 | 2001 | 2011 | 1991-2011 | 1991-2011 | |
Jalokati Sadar | 179.3 | 195.6 | 194.0 | 216.3 | 20.7 | 11.56 |
Rajapur | 123.1 | 143.7 | 145.6 | 148.5 | 4.8 | 3.93 |
Nalchity | 179.5 | 203.6 | 216.5 | 193.6 | -10.0 | -5.57 |
Kanthalia | 104.7 | 123.3 | 136.5 | 124.3 | 1.0 | 0.93 |
Total | 586.6 | 666.1 | 692.7 | 682.7 | 16.5 | 2.82 |
An Upazila-wide estimate of per capita woodlot was assessed from the estimate of tree coverage obtained from satellite images and available population statistics (Tab. 4). Change in per capita woodlot over the study period can be used as an indicator of whether the rate of population growth rose faster than the expansion of tree coverage in the district during the period of investigation. Per capita woodlot for the Jhalokati District was 0.029 ha in 1990 (area estimate in 1989 and population census of 1991) and 0.04 in 2010 (area estimate in 2010 and population census in 2011); the total increase in per capita woodlot was 0.011 ha. This indicates that the rate of woodlot expansion was faster than the rate of population growth; in other words, people have been planting more trees in the 2010s compared to the 1990s. In 1990, Jhalokati Sadar had the highest amount of per capita woodlot (0.032 ha) among the other three Upazilas. In 2010, Rajapur had the highest amount of per capita woodlot (0.046 ha). The largest expansion of per capita woodlot (0.017 ha) occurred in Rajapur Upazila. A possible explanation is that people living in the Upazila have a better motivation to plant trees.
Tab. 4 - Changes in Upazila-wide estimate of per capita woodlot over time (1989-1991 to 2010-2011).
Name of Upazila | Per capita woodlot (ha) | Changes in per capita woodlot 1989-1991 to 2010-2011 (ha) |
|
---|---|---|---|
1989-1991 | 2010-2011 | ||
Jalokati Sadar | 0.032 | 0.040 | 0.008 |
Kanthalia | 0.028 | 0.038 | 0.011 |
Nalchity | 0.028 | 0.040 | 0.012 |
Rajapur | 0.029 | 0.046 | 0.017 |
Total | 0.029 | 0.041 | 0.011 |
Conversion of cropland to orchard
In the north-western part of the study region belonging to Jhalokathi Sadar Upazila, croplands have been converted to orchard during the study period (Fig. 5). There is a long practice of maintaining guava and amra orchards in Jhalokati and the adjacent Pirojpur Districts. Interviews with local people indicated that the annual financial return from the orchards is higher compared to the return from agricultural crops. Hence, people are converting their cropland to orchards in the north-eastern part of the study area and in Jhalokati Sadar Upazila. Now, more lands are under tree cover in that area than in the 1990s because of orchard expansion.
Fig. 5 - Woodlot expansion in Jhalokati district during 1989-2010 because of the conversion of cropland to orchard: (a) Landsat TM image of 1989; (b) Landsat TM image of 2010; (c) RapidEye image of 2011. The images are overlaid with (a) a classified vector map of 1989 and (b and c) a vector change map for 1989-2010. The band combination of Landsat and RapidEye imagery is the same as in Fig. 4. The guava orchard is not distinguishable on the Landsat scene but can be separated on the RapidEye scene.
The general trend of increasing woodlots is noticed in the study region during the last two decades despite the losses of few woodlot patches in some locations. The analysis of multi-temporal satellite data found woodlot areas lost by river erosion located in close proximity to the rivers. In other locations, woodlots have been cleared because of urban expansion. These areas are scattered throughout the district.
Accuracy assessment of woodlot map
The accuracy of the 2010 woodlot map was assessed with RapidEye imagery and ground verification. The overall classification accuracy and the kappa statistics derived from the error matrix based on simple randomly sampled points were, respectively, 87% and 0.76 (Tab. S2 in Supplementary Material). The figures are 89% and 0.79 for overall accuracy and kappa statistics, respectively for the points generated by stratified random sampling (Tab. S3 in Supplementary Material).
Simple random sampling generated sample points without considering the allocation of samples to individual land cover categories, while stratified random sampling ensured to allocate representative samples in every land cover class. Stratified random sampling technique is particularly useful for assessing accuracy in any land cover map, if one or more land cover categories in the map are available in a limited extent; and the chance of allocating adequate samples in those land cover category is low using simple random sampling. Therefore, accuracy of those land cover class with smaller extent may not be appropriately estimated when simple random sampling is used.
The summary representing the sources of classification errors using points derived by simple random and stratified random sampling is presented in Tab. 5. The number of misclassified points was 39 for simple random sampling and 34 for the stratified random sampling procedure. The largest source of classification error was generated by mixed pixels and pixels located at boundaries: it affected 16 and 11 points generated by simple random sampling and stratified random sampling, respectively.
Tab. 5 - Different sources of errors with number of random points in accuracy assessment. Points were generated by simple random and stratified random sampling techniques.
Error type | Number of points | |
---|---|---|
Simple random sampling |
Stratified random sampling |
|
Boundary pixel/ Mixed pixel | 16 | 11 |
House in the woodlots | 5 | - |
Vacant space inside woodlots | 6 | 3 |
Linear tree formation | 5 | 3 |
Fragmented landscape inside woodlot | 2 | 2 |
Interpreter’s error | 5 | 3 |
Mistake in class assignment | - | 6 |
Small in size | - | 4 |
Image shipment | - | 1 |
Combined effect of mixed pixel / small in size | - | 1 |
Total | 39 | 34 |
The accuracy assessment of the Landsat derived woodlot map has been based on the interpretation of RapidEye images, being the fact that RapidEye image has higher spatial resolution (6.5 m) than the Landsat (30 m). The main sources of errors can be categorized into interpreter error, boundary pixel/mixed pixel, small size of objects, error in class assignment, fragmented landscape inside woodlot, trees arranged in linear shape, vacant space or houses inside woodlots etc. (details in Tab. S4 in Supplementary Material; examples in Fig. S1, Supplementary Material). Some errors were due to the small size of woodlots, which did not reach the threshold of 0.18 ha, equal to the minimum mapping unit for this study. If only woodlots larger than 0.18 ha were considered, the accuracy results would be further improved.
The accuracy results refer to the 2010 woodlot map. It was not possible to estimate the accuracy of 1989 woodlot map because any satellite data with higher spatial resolution was not available for that period and there was no obtainable ground truth information from that area. As a result, this study also did not attempt to estimate the accuracy of change map and change estimate. Therefore, the accuracy reported in this study has been assessed for recent time; if an historical map had similar accuracy the change map would have a lower, probably slightly, accuracy because of the adding errors from the two maps.
Discussion
Tree cover changes in rural landscape
Land use and land cover in rural areas is changing in different parts of the globe, which can create adverse impact in local economy and ecology. The changes are linked to environmental deterioration, depletion of soil resources, loss of biodiversity, urban growth, intensive cropping, land abandonment etc.; as a consequence, there is an urgency to develop an integrated strategy and policy for sustainable rural development keeping the historical and traditional cropping pattern and land utilization practice which are susceptible to anthropogenic intervention and conversion ([11], [15], [14], [10], [5]). A better knowledge on the transformations and conversion of landscape structure and composition and the drivers of changes is helpful for land resource planning ([5]).
Previous studies on land use and land cover change in the rural areas located in different regions of the globe have also contributed towards understanding the landscape change patterns in local, regional and global scale. Some examples of those changes in the context of Europe and America are enumerated in the following section. Biasi et al. ([5]) investigated the changes in land-use and landscape structure (2000-2008) in three districts of central Italy. The results indicated that crop intensification has created more homogenous and simplified landscape, and urban expansion led to a more fragmented and diversified landscape. Analysis of land use change and population density for the period of 1954-2008 was performed in two areas, one in plain-land and another in hilly-mountain area, of northern Italy ([33]). The processes of landscape change include urban expansion and, to some extent, intensification of cropping in the plain land, while land abandonment for natural regeneration and reforestation activity dominates the changes in the hilly-mountain area.
Changes in the distribution of different land cover classes and their spatial relationships have been assessed in a Mediterranean region located in Attica, Greece for the period of 1990-2000 ([32]). The results indicated that there was a shift from a spatially-heterogeneous and diversified agro-forest landscape towards a land-use structure diverged in medium- and low-quality areas with homogeneous (natural or urban) land uses. In a longer time-scale, Parcerisas et al. ([22]) studied environmental change of a coastal Mediterranean area, northeast of Barcelona, from 1850 to 2005. The study revealed that there was a massive environmental deterioration between 1950 and 2005. The main drivers of these changes identified in this study were the development of urban sprawl on croplands located in the coastal plains, and land abandonment and reforestation intermingled by residential areas, highways etc. on the hilly slopes.
Land cover change of North American croplands was estimated from 1850 to 1992 and the result showed a rapid loss in forests/woodlands and savannas/grasslands/steppes since 1850 ([27]). Substantial portion of abandoned croplands are located in eastern North America, which could be returned to natural vegetation. The assessment of land cover change in the Chaco forests of central Argentina revealed that approximately 80% area of undisturbed forest was converted to crops, pastures, and secondary scrub during the period 1969-1999 and the main driving forces of deforestation were the agricultural expansion, particularly soybean cultivation ([40]).
The current study contributed towards understanding of tree cover change processes in a rural landscape of Bangladesh. Cultivable cropping lands are declining in the study area because of the expansion of orchards and increase of human settlements associated with tree cover. Such conversion needs to be regulated by policy intervention; otherwise the conversion, if it continues further and further, may negatively impact the production of food grain and finally impose a threat to the food security of the country.
Consideration of spatial resolution in woodlot mapping
For mapping of woodlot resources, appropriate satellite data with suitable spatial resolution beyond spectral, temporal, and radiometric characteristics should be considered. Landsat data is suitable for woodlot mapping in the study area particularly where human settlements are surrounded by woodlots located in home gardens and distributed in a continuous manner.
Landsat datasets (or satellite sensor data with a spatial resolution of 20-30 m) are appropriate for mapping of woodlots; the datasets are cost-effective and easier to use in data processing compared to images with finer spatial resolution. The purchasing cost of satellite data will increase if the resolution becomes finer and more time is required in data interpretation, processing, and mapping. Appropriate mapping techniques should be considered as well. The use of data with a finer spatial resolution would increase the spectral variation originated from different image objects unveiled because of finer resolution data ([39]). Higher variation in reflectance properties would lead to create difficulty in class separation using statistical analysis and thus lead to increase uncertainty in classification if pixel-based classification technique is applied ([21]). Moreover, the availability of Landsat archive data is helpful for time series studies (i.e., for the estimation of changes in the extent of woodlot coverage), as it was assessed in the current study. Apart from Landsat data, historical satellite data with finer spatial resolution is often not consistently available for many countries.
The general consideration in change detection analysis using optical remote sensing is that multi-temporal satellite data should be originated from the same sensor, image resolutions (spatial and spectral) and acquisition dates (nearby date in the anniversary year), which will minimize the effects of sun angle, phenological condition of vegetation, seasonal influence, topography and sensor view angle on multi-date satellite images ([35], [16]). The availability of historical image (1989) for this change detection covering the study area is Landsat. Therefore, the current study considered only Landsat data (both for historical and recent time) for mapping and change detection analysis of woodlots in order to avoid any inconsistency originated because of the above reasons.
Limitations
The study faced limitations including the time requirement, particularly for the manual part of methodology. A considerable amount of time was required to add or drop the vector polygons. Another limitation was the interpretation of mixed pixels or the pixels representing more than a single land cover type. However, it should be kept in mind that the presence of mixed pixels (defined as having a tree cover of approximately 50%) covering both tree and non-tree cover is much lower than the pure pixels or pixels with a dominant fraction of tree or non-tree cover.
Conclusions
The study presented a semi-automated approach that uses automatic pixel based classification and manual updating of the extracted woodlot database. The method is applicable for mapping of woodlots in a densely populated rural landscape using Landsat data. Landsat imagery can be used for mapping of woodlot resources if the trees are distributed in a continuous pattern and extended over several households covering more than two Landsat pixels (parcel size of 0.18 ha). In the study area, the dimensions (polygons in map) of woodlots are mostly larger enough to prepare land cover map and land cover change map as well as assess their extent and changes for the study period using the multi-temporal Landsat scenes. The accuracy of Landsat derived 2010 woodlot map varies within 87%-89% when checked with RapidEye datasets.
Landsat data is suitable for woodlot mapping particularly when woodlots are distributed in a continuous pattern. However, small isolated woodlots lower than two Landsat pixels, individual isolated trees or trees in small groups, trees planted in roadside or railway side (linear shaped plantation with one or two rows of trees) are not detectable using Landsat; data with finer spatial resolution will be required. Most of the errors originated from woodlot map generated by Landsat scene are related to the spatial resolution of Landsat data (for example, pixels located at the boundary or mixed pixel).
The advantage of using Landsat is the freely available archive data. The availability Landsat Thematic Mapper data since 1984 has widened the scope of woodlot monitoring from the mid-1980s. Moreover, the availability of panchromatic band with spatial resolution of 15 m in Landsat 7 Enhanced Thematic Mapper Plus (ETM+ -since 1999, Scan Line Corrector, SLC, failure after 2003) and Landsat 8 OLI (Operational Land Imager - since 2013, in operational) also gives better possibility of woodlot mapping and change analysis using Landsat data.
The minimum size of a land parcel representing woodlot in agricultural landscapes should be extended over two pixels in order to be appropriately represented in the maps. The consideration of spatial resolution is important in mapping woodlot. Woodcock & Strahler ([39]) recommend in fact an image resolution of one-half to three-fourths the size of target objects.
Woodlot coverage in Jhalokati District has been increased from 27% to 39% during 1989-2010; the changes are associated with the establishment of new households in croplands and conversion of croplands to orchard. Population growth triggers the necessity of establishment of new households in the area. The underlying driver of land conversion to orchard is the higher financial returns. The drivers of landscape change are often associated with the socioeconomically driven changes in terms of population dynamics, institutional factors, changes in labor markets, educational levels, etc. ([9]).
The methodology derived in this study should be replicated in other parts of the globe to generate better knowledge and understanding on the dynamics of landscape change in rural areas. Develop continuous monitoring system and initiate necessary steps for the conservation of rural landscape will minimize the negative environmental impacts of landscape modifications ([31], [32]). The quantification of landscape change and the analysis of change processes will be helpful to decide whether there is a need of any policy formulation and intervention to regulate further land conversions in any region.
The study faced limitations including time required for the manual portions of the mapping methodology. Further study should be aimed at generating faster and automatic approaches, replacing the manual part of the methodology for woodlot mapping.
Acknowledgements
The authors acknowledge the United States Geological Survey (USGS) for freely available Landsat scenes used in this research. RapidEye imagery was procured by Climate Change Research & Impact Study (CRAIST) Project of Bangladesh Space Research and Remote Sensing Organization (SPARRSO) funded by Bangladesh Climate Change Trust Fund. Shaheen Khan, Chairman of SPARRSO is acknowledged to grant permission to publish the research work.
References
Gscholar
Gscholar
Gscholar
Gscholar
CrossRef | Gscholar
Gscholar
CrossRef | Gscholar
Authors’ Info
Authors’ Affiliation
M Shafiqul Islam
M Abu Taleb Pramanik
Forestry Division, Bangladesh Space Research and Remote Sensing Organization, Agargaon, Sher-E-Bangla Nagar, 1207, Dhaka (Bangladesh)
Corresponding author
Paper Info
Citation
Rahman MM, Islam MS, Pramanik MAT (2018). Monitoring of changes in woodlots outside forests by multi-temporal Landsat imagery. iForest 11: 162-170. - doi: 10.3832/ifor2021-010
Academic Editor
Luca Salvati
Paper history
Received: Feb 21, 2016
Accepted: Nov 23, 2017
First online: Feb 19, 2018
Publication Date: Feb 28, 2018
Publication Time: 2.93 months
Copyright Information
© SISEF - The Italian Society of Silviculture and Forest Ecology 2018
Open Access
This article is distributed under the terms of the Creative Commons Attribution-Non Commercial 4.0 International (https://creativecommons.org/licenses/by-nc/4.0/), which permits unrestricted use, distribution, and reproduction in any medium, provided you give appropriate credit to the original author(s) and the source, provide a link to the Creative Commons license, and indicate if changes were made.
Web Metrics
Breakdown by View Type
Article Usage
Total Article Views: 19204
(from publication date up to now)
Breakdown by View Type
HTML Page Views: 13996
Abstract Page Views: 859
PDF Downloads: 3503
Citation/Reference Downloads: 12
XML Downloads: 834
Web Metrics
Days since publication: 2350
Overall contacts: 19204
Avg. contacts per week: 57.20
Article Citations
Article citations are based on data periodically collected from the Clarivate Web of Science web site
(last update: Nov 2020)
Total number of cites (since 2018): 2
Average cites per year: 0.67
Publication Metrics
by Dimensions ©
Articles citing this article
List of the papers citing this article based on CrossRef Cited-by.
Related Contents
iForest Similar Articles
Review Papers
Remote sensing of selective logging in tropical forests: current state and future directions
vol. 13, pp. 286-300 (online: 10 July 2020)
Review Papers
Remote sensing-supported vegetation parameters for regional climate models: a brief review
vol. 3, pp. 98-101 (online: 15 July 2010)
Review Papers
Accuracy of determining specific parameters of the urban forest using remote sensing
vol. 12, pp. 498-510 (online: 02 December 2019)
Research Articles
Afforestation monitoring through automatic analysis of 36-years Landsat Best Available Composites
vol. 15, pp. 220-228 (online: 12 July 2022)
Research Articles
Assessing water quality by remote sensing in small lakes: the case study of Monticchio lakes in southern Italy
vol. 2, pp. 154-161 (online: 30 July 2009)
Research Articles
Landsat TM imagery and NDVI differencing to detect vegetation change: assessing natural forest expansion in Basilicata, southern Italy
vol. 7, pp. 75-84 (online: 18 December 2013)
Research Articles
Experimenting the design-based k-NN approach for mapping and estimation under forest management planning
vol. 5, pp. 26-30 (online: 27 February 2012)
Technical Reports
Detecting tree water deficit by very low altitude remote sensing
vol. 10, pp. 215-219 (online: 11 February 2017)
Research Articles
Estimation of aboveground forest biomass in Galicia (NW Spain) by the combined use of LiDAR, LANDSAT ETM+ and National Forest Inventory data
vol. 10, pp. 590-596 (online: 15 May 2017)
Review Papers
Remote sensing support for post fire forest management
vol. 1, pp. 6-12 (online: 28 February 2008)
iForest Database Search
Search By Author
Search By Keyword
Google Scholar Search
Citing Articles
Search By Author
Search By Keywords
PubMed Search
Search By Author
Search By Keyword