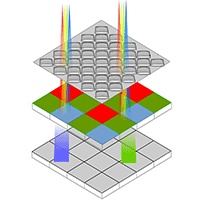
Detecting tree water deficit by very low altitude remote sensing
iForest - Biogeosciences and Forestry, Volume 10, Issue 1, Pages 215-219 (2017)
doi: https://doi.org/10.3832/ifor1690-009
Published: Feb 11, 2017 - Copyright © 2017 SISEF
Technical Reports
Abstract
In a context of climate change and expected increasing drought frequency, it is important to select tree species adapted to water deficit. Experimentation in tree nurseries makes it possible to control for various factors such as water supply. We analyzed the spectral responses for two genetic varieties of Douglas fir sapling exposed to different levels of water deficit. Our results show that the mean NDVI derived from remote sensing at very low altitudes clearly differentiated stress levels while genetic varieties were partially distinguished.
Keywords
Very Low Altitude Remote Sensing, Water Deficit, Variety, Douglas Fir
Introduction
Important innovations in satellite sensors over the last decades have made available a great deal of information about landscape monitoring ([12]). Using plant reflectance of both high and low wavelengths in infrared and red bandwidths, many vegetation indices have been created. Among these, the NDVI (Normalized Difference Vegetation Index) developed by ([15]) is being widely used to study agricultural activities, biomass, plant productivity, chlorophyll concentrations and phenology. In forests, the NDVI has been used for about thirty years to assess fire risks ([6]). In terms of stand productivity, NDVI values are strongly linked with leaf area index, which is a good indicator of plant biomass ([18]). Moreover, other studies have used the NDVI for tree species identification on monoculture plots ([5]). In addition, Camarero et al. ([4]) used variations in NDVI to detect an increase in canopy cover preceding mast years for Quercus ilex. For drought monitoring, this vegetation index is of particular interest ([11], [17]). Drought conditions result in a reduction in soil water availability, and reduced levels of water in the soil layers alter both soil-root and leaf-atmosphere interfaces ([3]). With global climate change, droughts are expected to become more frequent, and it is important to plant tree varieties selected for their resistance to dry conditions. Douglas fir (Pseudotsuga menziesii [Mirb.] Franco) was introduced in France for its exceptional stand productivity and the quality of its wood. However, this species comes from regions where annual rainfall exceeds 800 mm year-1, a level that is not always reached in the areas where the tree was introduced ([16]). Particularly for saplings, water stress can cause mortality because the young tree’s shallow roots are subjected to high temperatures in the topsoil ([2]). In tree nurseries, we can experiment with drought effects on young trees by controlling the water supply. Coupled with the recently developed NDVI technology for crop monitoring ([8], [10]), we used modified commercial digital cameras and a mobile darkroom in order to extract NDVI at low cost. We studied the spectral response of two Douglas Fir varieties exposed to different stress levels.
Material and methods
Plant material and experimental design
The experiment took place on two-year-old Douglas fir saplings in a greenhouse during the 2013 growing season. Twenty-four boxes were planted with saplings following a two-way randomized block design crossing two genetic varieties (A and B) and six water stress levels, with one replication. Each box had been planted in 2012 on bare soil with 30 one-year-old trees. The rectangular boxes were 1.40 m by 1.10 m and 0.78 m deep (Fig. 1). The volume of soil available for roots in each box was 0.70 m3. Stress levels were measured by probes (TRIME-IPH) and 6 categories from 1 (wettest) to 6 (driest) were distinguished by Time-Domain Reflectometry (TDR) monitoring. Tab. 1 presents the percentage of soil water content for each stress level. Note that the intermediate level (Stress 3) was characterized by normal watering (15% water content) during the first phase of the experiment, then watering was completely suspended on August 6th, 2013 in order to simulate summer drought conditions. The soil water content for this level was nearly the same as stress level 6 at the end of the experiment (November 2013).
Tab. 1 - Percentage of soil water content by stress level.
Stress level | Soil Water (%) |
---|---|
Water deficit 1 | 19.6 |
Water deficit 2 | 17.3 |
Water deficit 3 | 15 |
Water deficit 4 | 12.7 |
Water deficit 5 | 10.4 |
Water deficit 6 | 8.1 |
Image acquisition
We used a modified commercial digital camera to access the infrared bandwidth and an identical non-modified digital camera to assess the red band. Digital camera sensors are sensitive to the full light spectrum but manufacturers affix a Color Filter Array (CFA), called “Bayer Matrix” (Fig. 2), in order to record only visible wavelengths (red, green and blue). When this filter is removed, commercial cameras become sensitive to infrared wavelengths ([14]). By photographing the same target with one modified and one non-modified Canon® EOS 350D digital camera, we recorded both near infrared (NIR) and red (from RGB) bands that could be used to calculate the NDVI. A mobile darkroom was built in order to ensure identical photography conditions: target center points (tree box), light intensity and camera height (Fig. 3a, Fig. 3b). Cameras were attached to a sliding support on the top of the dark chamber in order to move alternatively each camera on a common image acquisition vertical axis ideally located in the center of the dark chamber roof. For light intensity we chose tungsten lights due to the major part of radiation emitted in the red and infrared wavelengths. The roof of the dark room was equipped with 6 tungsten lights (model Philips Aluline 111®, 50W, G53, 12V 8D, 1CT) provided by batteries with accurate tension monitoring in order to guaranty color temperature of 3000 K. Camera settings were kept unchanged throughout the experiment and images were recorded in RAW format with a constant focal length of 34 mm and the best resolution (3456 × 2304 pixels). We photographed the 24 boxes at three dates during the 2013 growing season: August 30th, October 4-5th, November 4-5th.
Image treatment
We processed the images with the ArcGIS® software version 10.2. In lack of spectral calibration of cameras, we divided red and near infrared bands with a gray target (more visible in infrared spectrum) of constant reflectance in order to overcome different lighting effects related to bandwidth (Fig. 4). A panel was painted with three layers of gray matt acrylic paint (AFNOR NF T 36-005) to obtain a homogeneous reference surface with low reflectance. An image of the gray target was acquired with the modified and non-modified cameras in order to quantify lighting heterogeneity, reflectance from lateral walls and other uncontrolled effects. Due to different wavelengths in infrared and visible bandwidths, settings differ from cameras (Tab. 2). Thereafter we kept same settings of cameras for the plant material image acquisition. Only one picture of red and infrared spectrum was used as reference for correcting all pictures acquired on plant material.
Tab. 2 - Canon® EOS 350D Camera settings.
Camera | Shutter speed | Sensitivity | Aperture |
---|---|---|---|
NIR | 0.4 | 100 | 22.0 |
NIR GRAY TARGET | 0.4 | 100 | 22.0 |
RGB | 0.5 | 100 | 11.0 |
RGB GRAY TARGET | 0.5 | 100 | 11.0 |
We performed our analysis on a square mask centered on the middle of the image to avoid vignette effects (500 × 500 pixels). The NDVI of each box was calculated by automatic image processing for the three dates as follows (eqn. 1):
where ρ
is the reflectance value in spectral bands.
Statistical analyses
We used linear mixed effects models with gaussian error distribution to analyse the effects of stress (6 levels) and varieties (2 levels) and their interaction on the mean NDVI (calculated on 25 × 104 pixels per box and per date). A random “box” effect was added on the intercept to take into account a potential effect of replication (there were two boxes for a given variety and a level of stress, 12 replications in total). We used an analysis of variance (ANOVA, α = 0.05) to assess the effects of treatments (stress × variety) on the response variable. All analyses were performed in R version 3.1.0 ([13]), using the library “nlme”.
Results
Stress effect
In general, NDVImean decreased with stress intensity during the growing season (Fig. 5a, Fig. 5b, Fig. 5c). Specifically, starting in August, NDVImean decreased almost linearly from stress level 1 (well watered) to 5 (almost never watered). Fig. 5c shows the lowest NDVImean values for stress level 6 at around 8 percent soil water content. By the end of the experiment, all the plants in this category had died. For the second period (Fig. 5b), when watering was suddenly stopped in August, NDVImean decreased for stress 3. This trend was more pronounced at the end of the growing season (Fig. 5c). Throughout the experiment, stress effect levels were significantly different (Tab. 3).
Fig. 5 - Mean NDVI at different dates. Different letters indicate significant differences in stress intensity (Tukey’s HSD test, with P < 0.05).
Tab. 3 - ANOVA results of the effects of stress and origin on mean tree NDVI per box for three dates (calculated on 25 × 104 pixels). (df): degrees of freedom; (*): p ≤ 0.05; (**): p ≤ 0.01; (***): p ≤ 0.001.
Date | Variable | df | Mean Square |
F value | Pr>(F) |
---|---|---|---|---|---|
NDVI 30 Aug 2013 |
STRESS | 5 | 0.65 | 82.02 | 7.45e-09 *** |
VARIETIES | 1 | 0.00 | 0.00 | 0.93 | |
ST × VA | 5 | 0.00 | 0.84 | 0.54 | |
NDVI 4-5 Oct 2013 |
STRESS | 5 | 0.17 | 545.14 | 1.03e-13 *** |
VARIETIES | 1 | 0.00 | 12.65 | 0.003 ** | |
ST × VA | 5 | 0.00 | 3.15 | 0.04 * | |
NDVI 4-5 Nov 2013 |
STRESS | 5 | 0.14 | 251.23 | 1.04e-11 *** |
VARIETIES | 1 | 0.00 | 7.57 | 0.01 * | |
ST × VA | 5 | 0.00 | 1.22 | 0.35 |
Variety effect
During the growing season, differences between varieties were slightly statistically significant starting since October. There was only one significant interaction between stress level and genetic variety in the second period (Tab. 3).
Discussion
In this experiment, we studied the spectral response of two Douglas Fir varieties exposed to different water stress levels during the 2013 growing season. To be cost effective, we adapted a method used in crop studies and used a modified digital camera and a non-modified digital camera ([14], [8], [7]) and a mobile darkroom. This technology has also been used for forest monitoring with Unmanned Aerial Vehicles ([9]).The results in Tab. 3, Fig. 5a, Fig. 5b and Fig. 5c are promising for very low altitude NDVI in water deficit monitoring. Because water deficit events strongly impact young tree roots ([2]), it is important to test the most suitable tree species in a global climate change context. We were not able to provide results on genetic variety effects in our experiment due to methodological limitations (not enough replications) and similar spectral responses by the two genetic varieties. From a forestry point of view, these results are insufficient. Indeed, an experiment on only one growing season cannot predict stress behavior over the life of a stand. We should measure dendrometric variables for at least two successive years, as suggested in Becker’s work ([1]), in order to test correlations between tree sizes and spectral responses.
Conclusions
Very low altitude remote sensing could have a positive impact in tree nurseries. Further research may focus on testing photography with direct solar light in order to avoid the use a mobile darkroom. Other vegetation indices, including simpler ones based only on RGB bands, could also be tested, following the suggestion for crop monitoring by Meyer & Camargo Neto ([10]).
Acknowledgements
We thank Anne Villemey, Yoan Paillet and Emmanuel Cornieux for their constructive comments and Stéphane Matz, Vincent Bourlon, Pascal Croizet, Franck Stocchero, Aurélien Brochet and Cécile Joyeau for constructing the mobile darkroom and for image acquisition.
Author Contribution
S.L., P.B., G.P., conceived and designed the experiment, H.M., F.A., analyzed data, and all authors equally contributed in writing the paper.
References
Online | Gscholar
Gscholar
CrossRef | Gscholar
Authors’ Info
Authors’ Affiliation
Patrick Baldet
Frédéric Archaux
Gwénaël Philippe
IRSTEA National Research Institute of Science and Technology for Environment and Agriculture, Domaine des Barres, F-45290 Nogent-sur-Vernisson (France)
IRSTEA, National Research Institute of Science and Technology for Environment and Agriculture, 361 rue J.F. Breton, BP 5095, F-34196 Montpellier Cedex 5 (France)
Corresponding author
Paper Info
Citation
Martin H, Labbé S, Baldet P, Archaux F, Philippe G (2017). Detecting tree water deficit by very low altitude remote sensing. iForest 10: 215-219. - doi: 10.3832/ifor1690-009
Academic Editor
Giorgio Matteucci
Paper history
Received: Apr 27, 2015
Accepted: Nov 16, 2016
First online: Feb 11, 2017
Publication Date: Feb 28, 2017
Publication Time: 2.90 months
Copyright Information
© SISEF - The Italian Society of Silviculture and Forest Ecology 2017
Open Access
This article is distributed under the terms of the Creative Commons Attribution-Non Commercial 4.0 International (https://creativecommons.org/licenses/by-nc/4.0/), which permits unrestricted use, distribution, and reproduction in any medium, provided you give appropriate credit to the original author(s) and the source, provide a link to the Creative Commons license, and indicate if changes were made.
Web Metrics
Breakdown by View Type
Article Usage
Total Article Views: 20684
(from publication date up to now)
Breakdown by View Type
HTML Page Views: 15628
Abstract Page Views: 953
PDF Downloads: 2842
Citation/Reference Downloads: 37
XML Downloads: 1224
Web Metrics
Days since publication: 2723
Overall contacts: 20684
Avg. contacts per week: 53.17
Article Citations
Article citations are based on data periodically collected from the Clarivate Web of Science web site
(last update: Nov 2020)
(No citations were found up to date. Please come back later)
Publication Metrics
by Dimensions ©
Articles citing this article
List of the papers citing this article based on CrossRef Cited-by.
Related Contents
iForest Similar Articles
Review Papers
Remote sensing-supported vegetation parameters for regional climate models: a brief review
vol. 3, pp. 98-101 (online: 15 July 2010)
Review Papers
Accuracy of determining specific parameters of the urban forest using remote sensing
vol. 12, pp. 498-510 (online: 02 December 2019)
Review Papers
Remote sensing of selective logging in tropical forests: current state and future directions
vol. 13, pp. 286-300 (online: 10 July 2020)
Research Articles
Assessing water quality by remote sensing in small lakes: the case study of Monticchio lakes in southern Italy
vol. 2, pp. 154-161 (online: 30 July 2009)
Research Articles
Afforestation monitoring through automatic analysis of 36-years Landsat Best Available Composites
vol. 15, pp. 220-228 (online: 12 July 2022)
Technical Reports
Remote sensing of american maple in alluvial forests: a case study in an island complex of the Loire valley (France)
vol. 13, pp. 409-416 (online: 16 September 2020)
Review Papers
Remote sensing support for post fire forest management
vol. 1, pp. 6-12 (online: 28 February 2008)
Research Articles
Evaluation of hydrological and erosive effects at the basin scale in relation to the severity of forest fires
vol. 12, pp. 427-434 (online: 01 September 2019)
Review Papers
Analysis of full-waveform LiDAR data for forestry applications: a review of investigations and methods
vol. 4, pp. 100-106 (online: 01 June 2011)
Research Articles
Modelling dasometric attributes of mixed and uneven-aged forests using Landsat-8 OLI spectral data in the Sierra Madre Occidental, Mexico
vol. 10, pp. 288-295 (online: 11 February 2017)
iForest Database Search
Search By Author
Search By Keyword
Google Scholar Search
Citing Articles
Search By Author
Search By Keywords
PubMed Search
Search By Author
Search By Keyword