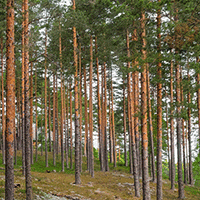
Relationship between environmental parameters and Pinus sylvestris L. site index in forest plantations in northern Spain acidic plateau
iForest - Biogeosciences and Forestry, Volume 9, Issue 3, Pages 394-401 (2016)
doi: https://doi.org/10.3832/ifor1600-008
Published: Jan 16, 2016 - Copyright © 2016 SISEF
Research Articles
Abstract
The assessment of forest productivity at early stages of stand development may help to define the most appropriate silviculture treatment to be applied for each stand. Site index (dominant height at a reference age) is a useful tool for forest productivity estimation. The aim of this study was to develop a model to predict site index for Scots pine (Pinus sylvestris L.) plantations in northern Spain acidic plateau by using soil (physical, chemical and biochemical), climatic and physiographic parameters. To meet this objective, data from 35 stands classified into three different site quality classes and 63 soil, climatic and physiographic parameters were examined in order to develop a discriminant model. After selecting 12 discriminant models which were biologically consistent and presented the higher cross-validated rate of correct classification, a model including four parameters (latitude, inorganic Al, porosity and microbial biomass carbon) as predictors was chosen. The discriminant model classified 71% of cases correctly and no inferior-quality stands were misassigned to the highest quality class. Soil and physiographic parameters included in the above model are easily obtainable in the field or by simple laboratory analysis, thus our results can be easily integrated in operational forestry to determine site quality.
Keywords
Soil-Site Method, Site Productivity, Environmental Factor, Discriminant Analysis, Principal Components
Introduction
Site productivity determination is needed to carry out sustainable management of stands. Productivity estimation at early stages allows defining appropriate silvicultural treatments to be applied. Forest productivity is usually estimated using site and stand factors. Site index (stand dominant height at a reference age) is widely used because it strongly correlates with wood production ([35], [46]). Dominant height is also easy to measure and is unaffected or lightly affected by usual silvicultural practices such as systematic, semisystematic or low thinning ([53]). However, other kinds of practices such as high thinning or high grading modify the dominant height of the stand, causing the underestimation of site index when dominant height is used as a predictor of productivity ([11]). In these cases, the use of soil-site relationships is more suitable for estimating site quality.
Pinus sylvestris L. is the most widely distributed pine species worldwide. Spanish stands constitute the southern limit of its distribution, where it occupies 1.28 million hectares ([43]). Knowing the environmental parameters that determine Pinus sylvestris L. site index is very helpful for developing tools aimed to predict site index when stand factors such as dominant height are unavailable.
Several studies have examined the relationships between site quality and environmental parameters, notably, soil (physical and chemical), physiographic and climatic variables.
Soil physical properties are often included in models as predictor variables to estimate site index from site factors ([9], [2], [3], [12], [44]). Soil chemical properties ([36], [44]) and organic horizon parameters ([39], [1]) are less frequently considered. Studies including biochemical aspects are scarce, despite the fact that microorganisms are known to be a key factor in soil quality ([47], [17]).
Bravo & Montero ([11]) developed a discriminant model for Pinus sylvestris in the Ebro Basin that included silt and clay content and cation exchange capacity as predictors, and correctly classified 64% of plots within their site index classes. White ([55]) found that the rate of height growth of Pinus sylvestris in Great Britain was mainly related to solar radiation, soil texture and soil moisture content. Hagglund & Lundmark ([18]) developed several models to predict Pinus sylvestris site index in Sweden with latitude, altitude, soil depth and texture as predictors. For the same species, Aertsen et al. ([1]) found that granulometric fractions and litterfall N content were the best predictors for forest productivity in Flanders, while Sharma et al. ([45]) included several physiographic factors (latitude, aspect, slope) as well as soil depth, year of stand origin and sum of temperatures in their equations to predict site index for Scots pine in Norway. A discriminant model developed by Bravo-Oviedo & Montero ([9]) for Pinus pinea L. in southeastern Spain also included textural parameters and, together with altitude, correctly classified 75% of the plots into their site index classes. Bravo et al. ([12]) developed a discriminant model for Pinus pinea in Calabria (Italy) which correctly classified 61% of the stands using only two predictor variables: clay content and slope. Consequently, textural parameters (as well as physiographic parameters such as slope, latitude or altitude) are often included in site index models ([11], [10], [1], [44], [45]).
Bravo-Oviedo et al. ([10]) developed two equations to predict Pinus pinaster Ait. site index in Spanish stands by using multiple linear regression, with altitude, autumn and winter precipitation and mean annual temperature as predictor variables for the first model, and altitude, autumn and winter precipitation and clay content as predictor variables for the second model. For the same species, Pacheco ([36]) also predicted site index in Portugal using multiple linear regression with minimum autumn temperature, available K, porosity and sand content as predictors. Alvarez-Alvarez et al. ([3]) found that soil depth and mean summer temperature were the main factors that explained Pinus pinaster productivity in Asturias (Spain). Studies on Pinus radiata D. Don frequently used soil chemical properties related to nutrients because the species has a high nutrient demand ([40], [39], [2]).
The principle aim of this study was to develop a discriminant model to estimate site index for Pinus sylvestris L. plantations on the acid plateau in northern Spain using soil (physical, chemical and biochemical), climatic and physiographic parameters.
Material and methods
Study area and sampling plots
According to the Third National Forest Inventory ([14]), Pinus sylvestris accounts for more than 79 000 ha in northern Spain’s acid plateau (in the Castilla y León region) and is mainly found between 800 and 1600 m a.s.l. These stands are mainly protective and productive.
This study used 35 plots belonging to the Sustainable Forest Management Research Institute (Fig. 1) located in the acid plateau in northern Spain (Castilla y León region). The 35 plots were located in Pinus sylvestris monospecific stands originated from afforestation. The understory was dominated by Calluna vulgaris (L.) Hull, Erica australis L., Halimium alysoides (Lam.) Spach and Pterospartum tridentatum L. Stand ages ranged from 28 to 54 years. Plots were located between 926 and 1180 m a.s.l. Most plots presented zero slope and the maximum slope found was 12%. Mean annual temperature of the study area was 9.5 °C and mean annual precipitation was 730 mm. Soils were classified as Inceptisols ([22]). They were strongly acidic (pH 3.7-5.6) and the amount of available P was very low because of its immobilization by acid cations such as Fe and Al, with which phosphorus forms insoluble compounds. According to Lang, Martonne and the Annual Hydric indexes, the study area was classified as humid. Summary statistics for stand characteristics for the sample plots are presented in Tab. 1.
Tab. 1 - Stand characteristics of Pinus sylvestris L. plots (n=35) used to develop a discriminant model to predict site index on acidic plateau plantations in northern Spain. (SD): standard deviation.
Parameters | Mean | SD | Min | Max |
---|---|---|---|---|
Stand age (years) | 39.8 | 7.4 | 28.0 | 54.0 |
Stocking (trees ha-1) | 1102.9 | 423.0 | 400.0 | 2083.3 |
Dominant height (m) | 14.5 | 3.6 | 8.5 | 22.8 |
Mean height (m) | 13.6 | 3.7 | 7.3 | 22.9 |
Quadratic mean diameter (cm) | 20.3 | 4.1 | 13.9 | 34.7 |
Basal area (m2 ha-1) | 33.1 | 8.2 | 16.8 | 53.6 |
Site Index (m at 50 years age) | 18.0 | 2.8 | 12.6 | 22.6 |
Dominant height (H
0), defined according to the Assmann ([4]) criterion (medium height of the 100 thickest trees per ha), and age were determined for every plot. Site index of each plot was calculated based on the current dominant height and age of each plot, using the equation developed by Rio et al. ([38] - eqn. 1):
where H
01 is the current dominant height in meters (at current age T
1) and H
02 is the site index, i.e., the dominant height at the reference age of 50 years (T
2). The plots were assigned to quality classes based on their site index, and the site index limits between classes established by Río et al. ([38]). Five quality classes were assigned to the plots based on Río et al. ([38]): Class I = 24 m of dominant height at a reference age of 50 years (one plot); Class II = 21 m (12 plots); Class III = 18 m (8 plots); Class IV = 15 m (13 plots); and Class V = 12 m (one plot).
Classes I and V were represented by only one plot; therefore, we pooled Classes I and II; we also merged Classes IV and V. Finally, three different site productivity classes were redefined as follows: (i) high productivity (Classes I and II); (ii) medium productivity (Class III); and (iii) low productivity (Classes IV and V).
Sampling
Sampling was done on the organic horizon and 10-cm mineral topsoil according to the method by Jokela et al. ([26]), which has also been adopted by Bravo & Montero ([11]) and Bravo et al. ([12]), because environmental changes are more strongly reflected in this layer.
Four sampling points were established on each plot in the N, S, E and W directions at a distance of 5 m from the center of the plot. One undisturbed sample and one disturbed sample were taken from the mineral soil at each point. A steel cylinder (5 cm diameter and 5 cm height) was used to take undisturbed samples to keep their primitive structure. The four disturbed samples from each plot were thoroughly mixed to get a composite soil sample per plot.
Organic horizon thickness (OHT) on the soil surface was measured in the field. It was also sampled at the same 4 points in 20×20 cm quadrants and then thoroughly mixed to get a composite sample per plot of each fraction. Two fractions at the organic horizon were separated: almost undecomposed litter fraction (L), and fragmented fraction plus humified fraction (FH).
Physical, chemical and biochemical soil analysis
Disturbed soil samples were air dried to constant weight, sieved through a 2-mm mesh and used to determine physical, chemical and biochemical properties, in duplicate samples. Undisturbed soil samples were used to determine bulk density and field capacity.
Physical parameters included percentage of coarse particles (>2 mm - CO) and fine particles (< 2 mm - FI), particle distribution determined by pipette method; porosity (PO) using bulk density (BD) and real density (RD) determination, and available water (AW) as the difference between water contents at field capacity (FC) and permanent wilting point (WP) determined using a pF equipment (Eijkelkamp, Giesbeek, Netherlands).
Chemical parameters included: pH using a 1:2.5 (soil:water) suspension ([29]); total C (TC) and total N (TN) by dry combustion using a Leco CHN 2000® elemental analyser (LECO Inc., St. Joseph, Michigan, USA); easily oxidizable carbon (EOC - [54]); cation exchange capacity (CEC); exchangeable acidity (EA - [31]); exchangeable cations (Ca+2, Mg+2, K+ and Na+) by means of extracting with 1N ammonium acetate (pH=7 - [42]) and then determining the cations in the extract using an atomic absorption/emission spectrometer; base saturation (SAT) as the ratio between total exchangeable cations and cation exchange capacity; available P (AP) extracted using anion exchange membranes ([51]) and colorimetric determination of P in the extracts ([33]); amorphous Fe, Al and Mn (FeA, AlA, MnA) extracted with 0.2 M (pH=3) ammonium oxalate ([8]); organically bound Fe, Al and Mn (FeO, AlO, MnO) extracted with 0.1M Na4P2O7 ([5]); exchangeable Al (AlE) extracted with 1M KCl ([6]). Subsequently, Fe, Al and Mn were determined in all these extracts using inductively coupled plasma/ optical emission spectrometry (ICP-OES). Inorganic Al (AlI) was determined as the difference between amorphous and organically bound fractions of this element ([30]).
Biochemical parameters included: mineralizable C (Cmin - [23]); microbial biomass C, N and P (Cmic, Nmic and Pmic) using the fumigation-extraction method ([52]) with determination of C and N content in extracts with a Skalar TOC autoanalyser® (Skalar Inc., Breda, Netherlands) and colorimetric determination of P content ([33]). The relationships Cmic/Nmic, Cmic/TC, Cmin/TC and the microbial metabolic quotient (qCO2 = Cmin/Cmic) were also calculated.
Organic horizon samples were dried at 60 °C and weighed to determine the amount of biomass per hectare for L (OL) and FH (OFH) fractions. A representative portion was ground and analyzed with Leco CHN 2000® element analyser to determine total C and total N concentrations of L fraction ([TC]L, [TN]L) and FH fraction ([TC]FH, [TN]FH), as well as their [TC/TN]L and [TC/TN]FH ratios.
Climatic and physiographic data
Climatic and physiographic data were also considered. The Digital Climatic Atlas of the Iberian Peninsula ([34]) was used to obtain precipitation and temperature data. The climatic parameters calculated were: mean seasonal precipitation (PW: winter precipitation; PSP: spring precipitation; PSU: summer precipitation; PA: autumn precipitation), annual total precipitation (TP), mean annual temperature (MAT), mean temperature of the coldest/ warmest months (MTCM, MTWM), mean minimum temperature in the coldest month (MMCM), mean maximum temperature in the warmest month (MMWM), potential evapotranspiration (PET), real evapotranspiration (RET), deficit (DEF), surplus (SUR), Martonne Index, Lang Index and Annual Hydric Index (Ih - [50]).
The physiographic parameters studied were elevation (ELV), latitude (LAT) and slope (SLP).
Statistical analysis
The software Statgraphics Centurion® XVI ([48]) was used to carry out the Principal Component Analysis (PCA) on each group of variables (physical, chemical and biochemical soil variables; climatic variables; physiographic variables; and those related to organic horizon) to select non-correlated variables that contained most of the site variability. This analysis was applied to the groups of variables because multiple correlations are thought to appear among variables of the same group. We selected principal components that, as a whole, accounted for 70% of the site variation and presented an eigenvalue greater than 0.7. For each principal component, the variable with the highest absolute value coefficient (loading) was chosen, as proposed by Jolliffe ([27]).
The selected variables were tested for normality using the Shapiro-Wilk’s test, and those showing lack of normality were transformed using the functions log(X
), exp(X
), 1/X
, X
2 and √X
. Transformed variables showing lack of normality were excluded from subsequent analysis. Pearson’s correlation coefficients were calculated among the selected variables to avoid including strongly correlated variables into the Discriminant Analysis.
Finally, Discriminant Analysis were performed to obtain a discriminant model to predict site index from selected variables. Discriminant Analysis is a classification technique through which a new case is assigned to an established group according to its properties. Discriminant functions are a linear combination of the original variables that best discriminate among groups. Classification functions have the following general structure (eqn. 2):
where Y
i is the score obtained for each group (i), β
0, β
1, …, β
n are the obtained coefficients and X
i the values of the n
variables selected as predictors that represent soil, physiographic and climatic factors ([19]). New observations are classified into the i
group whose function present the largest value. This classification technique has previously been used in similar studies ([20], [11], [9], [12]). Prior probabilities of belonging to a group were considered equal for the three groups in the discriminant analysis. The model was evaluated by means of cross-validation, a procedure which consists of excluding one datum each time and then fitting the model with the remaining data points, and validating the model with the omitted observation ([53], [25]). Models were tested with three, four and five variables as predictors. Those that were biologically consistent and presented a higher correct cross-validated rate (percentage of plots correctly classified into their actual quality class) were selected. We preferred the models containing a smaller number of predictor variables, as well as models that correctly classified a higher percentage of plots within the highest quality class, because forest management efforts are concentrated on these classes.
Pearson’s correlation coefficient was calculated to determine the correlations between latitude and climate-related parameters.
Results
Summary statistics for soil, climatic and physiographic variables used to develop a discriminant model to predict site index for Pinus sylvestris in acidic plateau plantations in northern Spain are reported in Appendix 1 (Tab. S1, Tab. S2, Tab. S3, respectively),
Four principal components were selected from the PCA performed on the soil physical variables, which accounted for 93.5% of the total site variation. Sand defined following ISSS criteria (SANDIS), CO, silt defined following USDA criteria (SILTUS) and PO were selected as the best soil physical variables. From the PCA of the soil chemical variables, four principal components accounted for 84.6% of the variability. Soil chemical variables selected were AlE, EOC, AlI and TC/TN. Two principal components were selected from PCA performed on the soil biochemical variables, with 87.4% of the total variation explained. The variables selected were Cmic and Cmin/TC. Three principal components were selected from PCA of organic horizon variables accounting for 79.6% of variability. The variables selected were [TC/TN]FH, OHT and [TC/TN]L. Two principal components were selected from the PCA of climatic variables explaining 96% of the total variability. The climatic variables selected were Lang Index and MTWM . Finally, two principal components accounting for 97.4% of the variability were selected from the physiographic variables; the variables selected were LAT and ELV. Tab. 2 summarizes the statistics obtained from the PCA for the 17 selected variables.
Tab. 2 - Summary of principal component analysis and variables selected for each principal component. (SANDIS): Sand following ISSS criteria; (CO): Coarse particles; (SILTUS): Silt following USDA criteria; (PO): Porosity; (AlE): Exchangeable Al; (EOC): Easily Oxidizable Carbon; (AlI): Inorganic Al; (TC/TN): Total C/Total N; (Cmic): Microbial Biomass C; (Cmin/TC): Mineralizable C/Total C; ([TC/TN]FH): Total C/Total N in fragmented plus humified fraction of organic horizon; (OHT): Organic Horizon Thickness; ([TC/TN]L): Total C/Total N in litter fraction of organic horizon; (LANG): Lang Index; (MTWM): Mean Temperature of the Warmest Month; (LAT): Latitude; (ELV): Elevation.
Group of variables | Component number |
Cumulative Variance Percentage |
Selected Variables |
---|---|---|---|
Soil Physical Variables | 1 | 55.5 | SANDIS |
2 | 71.9 | CO | |
3 | 85.9 | SILTUS | |
4 | 93.5 | PO | |
Soil Chemical Variables | 1 | 51.0 | AlE |
2 | 73.4 | EOC | |
3 | 79.4 | AlI | |
4 | 84.6 | TC/TN | |
Soil Biochemical Variables | 1 | 54.2 | Cmic |
2 | 87.4 | Cmin/TC | |
Organic Horizon Variables | 1 | 35.3 | [TC/TN]FH |
2 | 59.4 | OHT | |
3 | 79.6 | [TC/TN]L | |
Climatic Variables | 1 | 82.1 | LANG |
2 | 96.0 | MTWM | |
Physiographic Variables | 1 | 65.8 | LAT |
2 | 97.4 | ELV |
Using Shapiro-Wilk’s test with α = 0.05, significant departures from normality was detected for several variables: SILTUS, AlE, AlI, Cmin/TC, MTWM and ELV. Several transformations were applied to these variables (log (X
), exp (X
), 1/X
, X
2, and √X
). ELV and MTWM also showed lack of normality after transformation and were not considered further. The remaining variables were transformed as follows: 1/SILTUS, exp (AlE), √AlI, log (Cmin/TC).
Finally, the variables selected to be used in the discriminant analysis were SANDIS, CO, SILTUS, PO, exp (AlE), EOC, √AlI, TC/TN, Cmic, log (Cmin/TC), [TC/TN]FH, OHT, [TC/TN]L, LAT and Lang Index.
The pairwise correlation matrix for the 15 variables selected (Tab. 3) shows that LAT and Lang Index, LAT and AlE, AlE and SILTUS, SANDIS and SILTUS were strongly correlated (Pearson’s correlation coefficient > 0.7). Consequently, these pairs of variables were not used in the discriminant analysis. Therefore, variables used in the classification functions studied were normally distributed and not strongly correlated. Twelve models were biologically consistent and presented a cross-validated error (percentage of plots classified into an incorrect quality class) lower than 35%. The selected discriminant models are shown in Tab. 4. The cross-validated error rates of the selected discriminant models ranged from 28.6% (models 3, 6, 7, 9 and 11) to 34.3% (models 4 and 10). Model 12 misclassified 31.4% of plots within an incorrect quality class with only three parameters (Tab. 4). However, misclassifications of the model 12 were considerable for the highest quality class (46% error rate). Models 3, 6, 7, 9 and 11 classified correctly 78.6% of plots belonging to the lowest quality class, 75.0% belonging to the medium quality class and 61.5% of plots belonging to the highest quality class. Nevertheless, models 3, 7, 9 and 11 misassigned 7.1% of plots belonging to the lowest quality class into the highest quality class. With model 6, no inferior-quality plot was misassigned to the highest quality class (Tab. 5). A plot of the discriminant functions of the model is displayed in Fig. 2 . Function 1 (mainly influenced by latitude and AlI) discriminated quite well among the lowest quality class and the others. Function 2 (mainly influenced by porosity and Cmic) discriminated between the medium and highest quality classes. Function 1 was negatively influenced by AlI but positively influenced by latitude. Function 2 was positively influenced by porosity and Cmic. As can be seen in Fig. 2, the lower quality class presents lower latitude and higher inorganic aluminium content. Medium and higher quality classes present higher latitude and lower inorganic aluminium content. Medium and higher quality classes differ as to their porosity and Cmic (both are higher in the highest quality class). For forest management purposes, we aimed to identify the most productive stands accurately in order to focus managerial attention. It is important to select models with a high correct classification rate for the higher quality class. Model 6 was consequently selected to predict site index class of Pinus sylvestris plantations located in Northern Spain acidic plateau. The parameters of model 6 are shown in Tab. 6.
Tab. 3 - Pairwise correlation matrix for the 15 variables selected. Pearson’s correlation coefficients (r) are shown. (LAT): Latitude; (TC/TN): Total C/Total N; (AlI): Inorganic Al; (AlE): Exchangeable Al; (EOC): Easily Oxidizable Carbon; (CO): Coarse particles; (SANDIS): Sand ISSS criteria; (SILTUS): Silt following USDA criteria; (PO): Porosity; (Cmic): Microbial Biomass C; (Cmin/C): Mineralizable C/Total C; ([C/N]FH): Total C/Total N in fragmented plus humified fraction of organic horizon; ([C/N]L): Total C/Total N in litter fraction of organic horizon; (OHT): Organic Horizon Thickness; (LANG): Lang Index.
Variable | TC/TN | √AlI | exp(AlE) | EOC | CO | SANDIS | 1/SILTUS | PO | Cmic | log(Cmin/TC) | [TC/TN]FH | [TC/TN]L | OHT | LANG |
---|---|---|---|---|---|---|---|---|---|---|---|---|---|---|
LAT | 0.49 | 0.53 | 0.70 | 0.42 | -0.13 | 0.36 | 0.60 | 0.09 | -0.32 | -0.69 | 0.24 | 0.23 | 0.44 | 0.93 |
TC/TN | - | 0.38 | 0.57 | 0.19 | 0.10 | 0.65 | 0.57 | -0.27 | -0.47 | -0.30 | 0.22 | 0.37 | 0.05 | 0.38 |
√AlI | - | - | 0.55 | 0.36 | -0.23 | 0.14 | 0.31 | 0.21 | -0.28 | -0.51 | 0.26 | 0.26 | 0.39 | 0.42 |
exp(AlE) | - | - | - | 0.32 | -0.21 | 0.44 | 0.70 | -0.14 | -0.34 | -0.67 | 0.31 | 0.18 | 0.38 | 0.60 |
EOC | - | - | - | - | -0.25 | -0.18 | 0.05 | 0.09 | 0.41 | -0.57 | -0.13 | 0.27 | 0.30 | 0.50 |
CO | - | - | - | - | - | 0.37 | 0.12 | -0.11 | -0.36 | 0.24 | -0.18 | -0.09 | 0.10 | -0.19 |
SANDIS | - | - | - | - | - | - | 0.81 | -0.26 | -0.52 | -0.21 | 0.25 | 0.12 | 0.14 | 0.21 |
1/SILTUS | - | - | - | - | - | - | - | -0.23 | -0.33 | -0.54 | 0.36 | 0.20 | 0.28 | 0.46 |
PO | - | - | - | - | - | - | - | - | 0.08 | 0.06 | 0.39 | -0.05 | 0.00 | 0.10 |
Cmic | - | - | - | - | - | - | - | - | - | 0.03 | -0.08 | 0.04 | -0.05 | -0.23 |
log(Cmin/TC) | - | - | - | - | - | - | - | - | - | - | -0.15 | -0.36 | -0.35 | -0.63 |
[TC/TN]FH | - | - | - | - | - | - | - | - | - | - | - | 0.29 | -0.10 | 0.08 |
[TC/TN]L | - | - | - | - | - | - | - | - | - | - | - | - | -0.16 | 0.07 |
OHT | - | - | - | - | - | - | - | - | - | - | - | - | - | 0.42 |
Tab. 4 - Discriminant models studied and correct classification rates. (LAT): Latitude; (TC/TN): Total C/Total N; (AlI): Inorganic Al; (CO): Coarse particles; (SANDIS): Sand ISSS criteria; (PO): Porosity; (Cmic): Microbial Biomass C; (Cmin/TC): Mineralizable C/Total C; ([TC/TN]FH): Total C/Total N in fragmented plus humified fraction of organic horizon; ([TC/TN]L): Total C/Total N in litter fraction of organic horizon.
No | Discriminant models a | Correct classification cross-validated rate (%) |
---|---|---|
1 | constant + LAT + √AlI + PO + Cmic + [TC/TN]FH | 68.6 |
2 | constant + LAT + √AlI + CO + log (Cmin/TC) + [TC/TN]FH | 68.6 |
3 | constant + LAT + √AlI + SANDIS + log(Cmin/TC) + [TC/TN]FH | 71.4 |
4 | constant + LAT + TC/TN + SANDIS + log(Cmin/TC) + [TC/TN]FH | 65.7 |
5 | constant + LAT + TC/TN + SANDIS + Cmic + [TC/TN]L | 68.6 |
6 | constant + LAT + √AlI + PO + Cmic | 71.4 |
7 | constant + LAT + √AlI + SANDIS + Cmic | 71.4 |
8 | constant + LAT + TC/TN + SANDIS + log(Cmin/TC) | 68.6 |
9 | constant + LAT + √AlI + SANDIS + [TC/TN]FH | 71.4 |
10 | constant + LAT + TC/TN + SANDIS + [TC/TN]FH | 65.7 |
11 | constant + LAT + TC/TN + SANDIS + [TC/TN]L | 71.4 |
12 | constant + LAT + TC/TN + SANDIS | 68.6 |
Tab. 5 - Correct classification cross-validated rates of model 6.
Actual quality class |
Predicted quality class (%) | ||
---|---|---|---|
Lowest | Medium | Highest | |
Lowest | 78.6 | 21.4 | 0.0 |
Medium | 12.5 | 75.0 | 12.5 |
Highest | 23.1 | 15.4 | 61.5 |
Fig. 2 - Plot of discriminant functions for the discriminant model selected to predict site quality for Pinus sylvestris L. plantations in northern Spain acidic plateau (blue squares represent the lowest quality class, red triangles represent the medium quality class and pink circles represent the highest quality class)
Tab. 6 - Classification functions in the model selected (Model 6) to predict site index class of Pinus sylvestris L. plantations in northern Spain acidic plateau. (LAT): Latitude; (AlI): Inorganic Al; (PO): Porosity; (Cmic): Microbial Biomass C.
Variable | Site index class | ||
---|---|---|---|
Lower | Medium | High | |
LAT | 25357.4 | 25395.4 | 25383.5 |
√AlI | -894.859 | -896.121 | -895.783 |
PO | 9.29309 | 9.2923 | 9.39763 |
Cmic | 5.62468 | 5.62664 | 5.6414 |
CONSTANT | -540528 | -542150 | -541648 |
Discussion
The 12 models selected included the latitude as a predictor variable, which means that latitude is a determinant factor of forest productivity in the area studied. All these models contained one soil physical variable (PO, CO or SANDIS) and one soil chemical variable (TC/TN or AlI). Some of them also included a biochemical variable (Cmic or Cmin/TC) and/or an organic horizon related variable ([TC/TN]L or [TC/TN]FH).
The model selected to predict site index class for Pinus sylvestris (model 6) included LAT, AlI, PO and Cmic as predictor variables. These variables represent three environmental aspects that affect tree growth: physiography, soil physics and nutrient availability.
The physiographic variable included in the model was LAT, an easily obtainable variable. Positive significant correlations (p< 0.05) were found between latitude and climatic parameters such as precipitations, SUR and Lang, Martone and Hydric indexes. The correlations between LAT and temperatures and DEF were significant and negative (p<0.05). Therefore, LAT indirectly includes climatic information in the model. Pinus sylvestris is sensitive to drought ([16]) and growth is partly driven by water availability. In this area, lower latitudes present higher hydric deficit and tree growth is lower, as observed in previous studies ([13], [41], [49]). Hagglund & Lundmark ([18]) and Sharma et al. ([45]) also found that latitude was a good predictor variable for Pinus sylvestris L. site index in Sweden and Norway stands.
Soil porosity (spaces between soil particles) adds soil physical information into the model. This variable combines textural and structural information and determines the aeration of soil and its water retention capacity. Together with LAT, PO determines soil water availability for the trees. Textural parameters have frequently been used to predict Pinus sylvestris L. forest productivity all over Europe ([18], [55], [11], [1], [44], [45]). AlI and Cmic represent the nutritional aspect. Trees require the same nutrients to grow and reproduce as other superior plants. However, due to nutrient cycling and the deep roots of most tree species, as well as mycorrhizal associations that allow the uptake of minimally available nutrients from soil, nutrient deficiencies are rare in forests ([37]). Nevertheless, some studies in northern and northeastern Spain have shown nutrient deficiencies in several Pinus species stands, mainly due to P in acidic soils ([39], [2]). Available P is an important factor in the studied area because the soils are strongly acidic. Soil acidity is related to limiting nutrient (such as P) availability and also influences soil microbial populations and their activity ([7]). Sewerniak & Piernik ([44]) found than pH was one of the best variables to describe site index for Pinus sylvestris L. in southwestern Poland. Molina et al. ([32]) found that inorganic Al correlated significantly with P immobilization, so this variable introduces information about P availability into the model. The biochemical variable included in the model was Cmic. This parameter reflects the amount of microflora present in the soil; this is a very active soil component as it takes part in mineralization processes ([15]), playing a key role in nutrient cycling ([24]) and determining the availability of nutrients such as N, P and S for plants ([21]). Mahía et al. ([28]) found higher values of biochemical parameters (such as microbial biomass C) in higher site index stands of Pinus sylvestris and Pinus pinaster in northwestern Spain.
The soil-site method developed allows predicting site index by means of a relatively small set of easily measurable parameters.
Conclusions
Our results showed that soil physical, chemical and biochemical parameters, as well as physiographic parameters, are driving factors in determining site index for Pinus sylvestris in acidic plateau plantations of northern Spain. The model selected to predict site index included four predictor variables: LAT, PO, AlI and Cmic. LAT in the studied area was strongly positively correlated with precipitations and water surplus. Along with PO, which relates with soil aeration and water retention capacity of soils, the model showed that forest productivity in the study area was partly driven by water availability. AlI reflected the strong acidity of soils that provoked nutrient immobilization and limited forest growth, while Cmic related to the amount of microflora present in the soil and the turnover rate of nutrients.
The selected model correctly classified 71% of cases, higher than usual in this kind of studies. No plots belonging to the lower quality class were misclassified in the higher quality class using this model, which is very important for forest managers. Soil and physiographic parameters included in the model are easily obtainable in the field or by means of simple laboratory analysis, so our results can be integrated in operational forestry to determine site quality.
Acknowledgements
TB carried out the field and laboratory work, ran the data analysis, discussed the results and wrote the paper; FB coordinated the research project, designed the experiment and corrected the manuscript; VP supported TB with the statistical analysis and corrected the manuscript and MBT designed the experiment, supported TB with the laboratory analysis, supervised the progress of the work and corrected the manuscript.
The authors are grateful to Elisa Mellado, Temesgen Desalegn, Olga López and Carlos Alejandro Mendoza for their assistance in the field work, and to Carmen Blanco and Juan Carlos Arranz for their advice in laboratory analysis.
This work was funded by the Ministry of Economy and Competitiveness of the Spanish Government (AGL2011-29701-C02-02, AGL2014-51964-C2-1-R) and the University of Valladolid and Banco Santander (predoctoral grant to TB).
References
Gscholar
Gscholar
Gscholar
Gscholar
Gscholar
Gscholar
Gscholar
Gscholar
Gscholar
Gscholar
Gscholar
Gscholar
Gscholar
Gscholar
Gscholar
Gscholar
Gscholar
Gscholar
Gscholar
Authors’ Info
Authors’ Affiliation
Felipe Bravo
Valentín Pando
María-Belén Turrión
Sustainable Forest Management Research Institute, University of Valladolid & INIA, Avda. Madrid 44, 34004 Palencia (Spain)
María-Belén Turrión
Departamento de Ciencias Agroforestales, E.T.S. Ingenierías Agrarias, Universidad de Valladolid (Spain)
Departamento de Producción Vegetal y Recursos Forestales, E.T.S. Ingenierías Agrarias, Universidad de Valladollid (Spain)
Departamento de Estadística e Investigación Operativa, E.T.S. Ingenierías Agrarias, Universidad de Valladolid (Spain)
Corresponding author
Paper Info
Citation
Bueis T, Bravo F, Pando V, Turrión M-B (2016). Relationship between environmental parameters and Pinus sylvestris L. site index in forest plantations in northern Spain acidic plateau. iForest 9: 394-401. - doi: 10.3832/ifor1600-008
Academic Editor
Andrea Cutini
Paper history
Received: Feb 13, 2015
Accepted: Sep 09, 2015
First online: Jan 16, 2016
Publication Date: Jun 01, 2016
Publication Time: 4.30 months
Copyright Information
© SISEF - The Italian Society of Silviculture and Forest Ecology 2016
Open Access
This article is distributed under the terms of the Creative Commons Attribution-Non Commercial 4.0 International (https://creativecommons.org/licenses/by-nc/4.0/), which permits unrestricted use, distribution, and reproduction in any medium, provided you give appropriate credit to the original author(s) and the source, provide a link to the Creative Commons license, and indicate if changes were made.
Web Metrics
Breakdown by View Type
Article Usage
Total Article Views: 20474
(from publication date up to now)
Breakdown by View Type
HTML Page Views: 14055
Abstract Page Views: 1036
PDF Downloads: 4250
Citation/Reference Downloads: 24
XML Downloads: 1109
Web Metrics
Days since publication: 3204
Overall contacts: 20474
Avg. contacts per week: 44.73
Article Citations
Article citations are based on data periodically collected from the Clarivate Web of Science web site
(last update: Nov 2020)
Total number of cites (since 2016): 10
Average cites per year: 2.00
Publication Metrics
by Dimensions ©
Articles citing this article
List of the papers citing this article based on CrossRef Cited-by.
Related Contents
iForest Similar Articles
Research Articles
Soil and forest productivity: a case study from Stone pine (Pinus pinea L.) stands in Calabria (southern Italy)
vol. 4, pp. 25-30 (online: 27 January 2011)
Research Articles
Assessment of age bias in site index equations
vol. 9, pp. 402-408 (online: 11 January 2016)
Research Articles
Potential impacts of regional climate change on site productivity of Larix olgensis plantations in northeast China
vol. 8, pp. 642-651 (online: 02 March 2015)
Research Articles
Site quality assessment of degraded Quercus frainetto stands in central Greece
vol. 8, pp. 53-58 (online: 12 May 2014)
Research Articles
The effect of soil conditions on submountain site suitability for Norway spruce (Picea abies Karst.) in Central Europe
vol. 16, pp. 210-217 (online: 31 July 2023)
Research Articles
Estimation of forest biomass components using airborne LiDAR and multispectral sensors
vol. 12, pp. 207-213 (online: 25 April 2019)
Research Articles
Estimating changes in soil organic carbon storage due to land use changes using a modified calculation method
vol. 8, pp. 45-52 (online: 17 June 2014)
Research Articles
Sensitivity analysis of RapidEye spectral bands and derived vegetation indices for insect defoliation detection in pure Scots pine stands
vol. 10, pp. 659-668 (online: 11 July 2017)
Research Articles
The effect of the calculation method, plot size, and stand density on the accuracy of top height estimation in Norway spruce stands
vol. 10, pp. 498-505 (online: 12 April 2017)
Research Articles
Using self-organizing maps in the visualization and analysis of forest inventory
vol. 5, pp. 216-223 (online: 02 October 2012)
iForest Database Search
Search By Author
Search By Keyword
Google Scholar Search
Citing Articles
Search By Author
Search By Keywords
PubMed Search
Search By Author
Search By Keyword